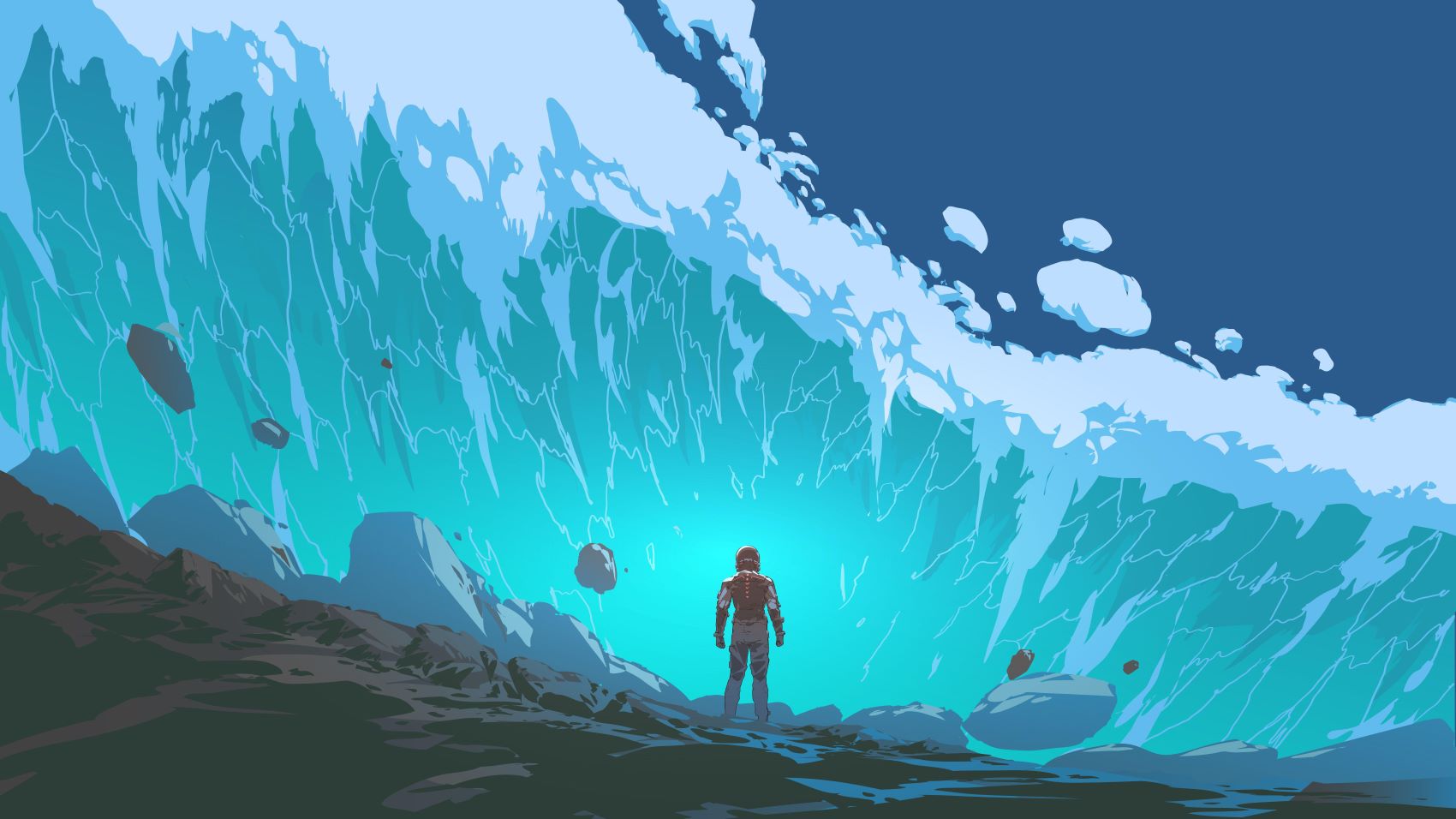
How to Make AI Projects Greener, Without the Greenwashing
www.informationweek.com
Samuel Greengard, Contributing ReporterFebruary 19, 20255 Min ReadTithi Luadthong via Alamy StockFor businesses across every industry, artificial intelligence is rapidly reshuffling the deck. The technology opens the door to deeper insights, advanced automation, operational efficiencies, and cost savings.Yet, AI also delivers some baggage. The power-hungry nature of the technology, which impacts everything from data centers to training and using generative AI models, raises critical questions about sustainability. AI could double data center electricity demand in the US by 2030.As a result, business and IT leaders can easily find themselves caught in the crossfire between AIs benefits and risks. As organizations pursue carbon targets and other sustainability issues, a lack of clarity about the technology -- and perceptions of inconsistencies -- can evoke charges of greenwashing.Sustainable AI touches everything from the direct energy requirements that power artificial intelligence models to supply chain, reporting, hardware and data center operations. It can also raise questions about when and where organizations should use AI -- and when they shouldnt.Sustainable AI is about using AI in ways that minimize environmental impact while promoting sustainability throughout its lifecycle, says Sammy Lakshmanan, a partner at PwC Sustainability. The goal isnt to just reduce AIs footprint. Its to make AI both effective and sustainable.Related:Beyond the AI HypeA growing challenge for CIOs and other tech leaders is to fully understand the impact of AI, including GPUs that devour energy at about a 10x rate over other chips. While no company wants to miss the opportunities that AI can deliver, its also important to recognize that the technology comes with costs. Theres a temptation for organizations to get caught up in an AI arms race without looking at the returns, states Autumn Stanish, a director and analyst at Gartner.A haphazard or inconsistent approach to AI can contribute to the perception that a company is engaging in greenwashing. Many of the common uses of AI link directly to climate change, says David Rolnick, an assistant professor in the School of Computer Science at McGill University. Framing specific AI initiatives as net positives or negatives isnt the right approach, he argues. Its vital to gain a more holistic understanding of how AI impacts sustainability.Greenwashing problems often revolve around two key issues, Rolnick says. First, companies that use carbon offsets must recognize that they arent reducing emissions produced by AI systems. Second, sloppy reporting creates more questions than answers. While quantifying the carbon generated from AI is difficult -- especially Scope 3 emissions -- a lack of transparency increases the odds that a company will find itself in the crosshairs of activists and the media.Related:But theres also the fundamental question of how an organization uses AI, Rolnick says. Its important to put AI to work strategically. There are many places where it can improve efficiency -- particularly when it comes to automating processes and optimizing system -- but there also are many instances where it doesnt provide any significant advantages. This includes tossing generative AI at every problem. In many cases, humans make better decisions, he states.As companies pursue carbon reduction targets, its important to identify where delivers specific strategic advantages -- and how it impacts sustainability in both positive and negative ways. Sustainable AI does not happen by accident -- it involves proper governance and engineering to create systems that are efficient and beneficial for productivity and innovation, Lakshmanan explains.Cracking the CodeTurning an AI strategy into broader sustainability issues helps build an energy framework based in renewables -- including wind, solar and emerging sources of nuclear energy, such as small modular reactors (SMRs). While this approach doesnt directly lower the energy demand for AI, it can significantly curtail carbon output.Related:The challenge lies in verifying that energy labeled as sustainable or carbon free is genuinely renewable, Lakshmanan points out. As a result, he recommends that organizations adopt transparency tools such as renewable energy certificates (RECs) and Power Purchase Agreements (PPAs) that help track the lifecycle impacts of renewable infrastructure.There are also practical steps organizations that help align AI with sustainability initiatives. This includes improvements in data center efficiency, such as better hardware and understanding when CPUs are a better option than GPUs. It also involves responsible data practices such as optimizing AI algorithms and models through pruning and sampling, and with transfer learning, which can significantly decrease computational demands by recycling pre-trained models.Transfer learning involves using a model trained for one task to improve results for a related task.Training and inferencing models in a horizontal or cross-cutting manner can alleviate the need to repeat processes across departments and groups, Lakshmanan points out. For example, Summarizing documents is a repeatable process whether it relates to sustainability or tax documents. Theres no need to train the system twice for the same capability, he explains.The end goal, Lakshmanan says, is to adopt a holistic approach that spins a tight orbit around both innovation and the greater use of renewables. For instance, if an organization uses carbon offsets, he recommends pairing the program with a meaningful decarbonization strategy. This ensures offsets complement broader sustainability targets rather than replacing them. It makes AI projects both innovative and environmentally responsible.Beyond the AlgorithmAvoiding greenwashing accusations also requires sound carbon accounting practices that can measure and track AI emissions. A growing array of consulting firms and private companies offer tools to track AI emissions and optimize energy usage based on real-time grid conditions.Measurement, combined with deeper analysis of AI and data center energy consumption, can boost efficiency in other ways. There are ways to use AI to analyze and improve power consumption, including putting AI on the edge, says Gillian Crossen, Risk Advisory Principal and Global Technology Leader at Deloitte. Not everything has to go through the data center. AI can also right-size models and produce other insights and gains that offset its power requirements.Finally, its important to avoid over-marketing claims or publishing data that presents an unrealistically positive picture to the public and investors, says Thomas P. Lyon, Dow Professor of Sustainable Science, Technology and Commerce at the University of Michigans Ross School of Business. An organization must be able to fully substantiate its claims about AI and sustainability, typically through metrics and third-party verification.With transparency across key segments, including customers, investors, partners and employees, the risks of greenwashing subside. Organizations should step back and think about how they can use AI effectively, Rolnick says. There are legitimate and productive use cases but theres also a lot of energy waste associated with AI. Without a detailed assessment and a clear understanding of the various factors the risks increase.About the AuthorSamuel GreengardContributing ReporterSamuel Greengard writes about business, technology, and cybersecurity for numerous magazines and websites. He is author of the books "The Internet of Things" and "Virtual Reality" (MIT Press).See more from Samuel GreengardNever Miss a Beat: Get a snapshot of the issues affecting the IT industry straight to your inbox.SIGN-UPYou May Also LikeWebinarsMore WebinarsReportsMore Reports
0 Commentarios
·0 Acciones
·49 Views