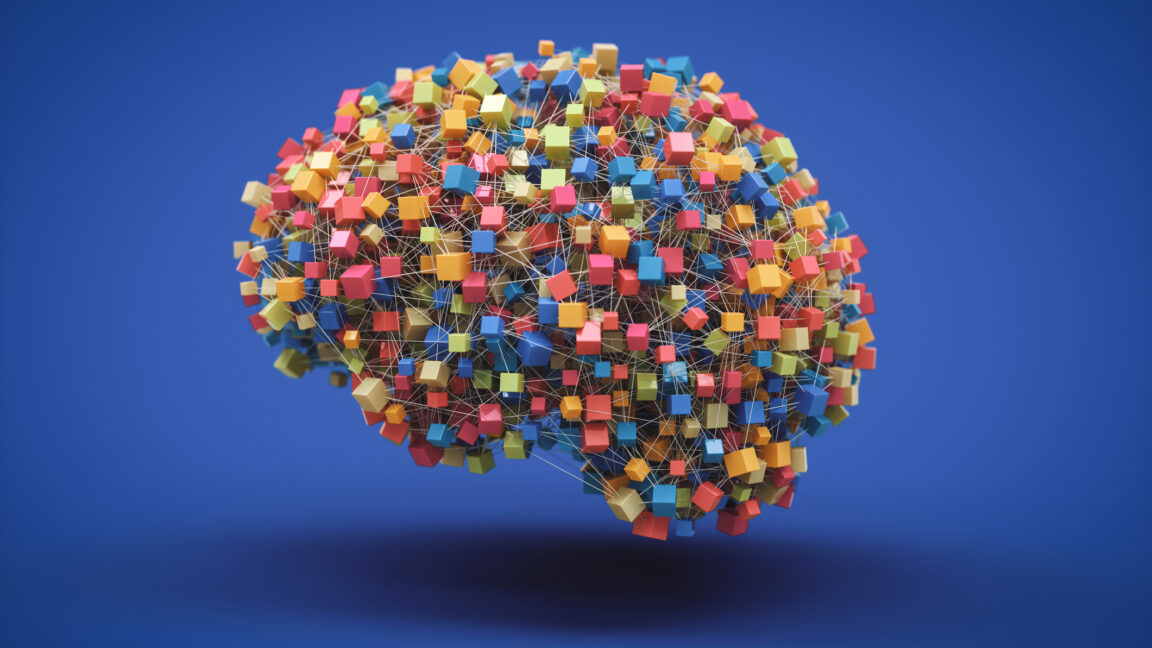
Why do LLMs make stuff up? New research peers under the hood.
arstechnica.com
Just say "I don't know" Why do LLMs make stuff up? New research peers under the hood. Claude's faulty "known entity" neurons sometime override its "don't answer" circuitry. Kyle Orland Mar 28, 2025 6:33 pm | 19 Which of those boxes represents the "I don't know" part of Claude's digital "brain"? Credit: Getty Images Which of those boxes represents the "I don't know" part of Claude's digital "brain"? Credit: Getty Images Story textSizeSmallStandardLargeWidth *StandardWideLinksStandardOrange* Subscribers only Learn moreOne of the most frustrating things about using a large language model is dealing with its tendency to confabulate information, hallucinating answers that are not supported by its training data. From a human perspective, it can be hard to understand why these models don't simply say "I don't know" instead of making up some plausible-sounding nonsense.Now, new research from Anthropic is exposing at least some of the inner neural network "circuitry" that helps an LLM decide when to take a stab at a (perhaps hallucinated) response versus when to refuse an answer in the first place. While human understanding of this internal LLM "decision" process is still rough, this kind of research could lead to better overall solutions for the AI confabulation problem.When a known entity isn'tIn a groundbreaking paper last May, Anthropic used a system of sparse auto-encoders to help illuminate the groups of artificial neurons that are activated when the Claude LLM encounters internal concepts ranging from "Golden Gate Bridge" to "programming errors" (Anthropic calls these groupings "features," as we will in the remainder of this piece). Anthropic's newly published research this week expands on that previous work by tracing how these features can affect other neuron groups that represent computational decision "circuits" Claude follows in crafting its response.In a pair of papers, Anthropic goes into great detail on how a partial examination of some of these internal neuron circuits provides new insight into how Claude "thinks" in multiple languages, how it can be fooled by certain jailbreak techniques, and even whether its ballyhooed "chain of thought" explanations are accurate. But the section describing Claude's "entity recognition and hallucination" process provided one of the most detailed explanations of a complicated problem that we've seen.At their core, large language models are designed to take a string of text and predict the text that is likely to followa design that has led some to deride the whole endeavor as "glorified auto-complete." That core design is useful when the prompt text closely matches the kinds of things already found in a model's copious training data. However, for "relatively obscure facts or topics," this tendency toward always completing the prompt "incentivizes models to guess plausible completions for blocks of text," Anthropic writes in its new research.Fine-tuning helps mitigate this problem, guiding the model to act as a helpful assistant and to refuse to complete a prompt when its related training data is sparse. That fine-tuning process creates distinct sets of artificial neurons that researchers can see activating when Claude encounters the name of a "known entity" (e.g., "Michael Jordan") or an "unfamiliar name" (e.g., "Michael Batkin") in a prompt. A simplified graph showing how various features and circuits interact in prompts about sports stars, real and fake. Credit: Anthropic A simplified graph showing how various features and circuits interact in prompts about sports stars, real and fake. Credit: Anthropic Activating the "unfamiliar name" feature amid an LLM's neurons tends to promote an internal "can't answer" circuit in the model, the researchers write, encouraging it to provide a response starting along the lines of "I apologize, but I cannot..." In fact, the researchers found that the "can't answer" circuit tends to default to the "on" position in the fine-tuned "assistant" version of the Claude model, making the model reluctant to answer a question unless other active features in its neural net suggest that it should.That's what happens when the model encounters a well-known term like "Michael Jordan" in a prompt, activating that "known entity" feature and in turn causing the neurons in the "can't answer" circuit to be "inactive or more weakly active," the researchers write. Once that happens, the model can dive deeper into its graph of Michael Jordan-related features to provide its best guess at an answer to a question like "What sport does Michael Jordan play?"Recognition vs. recallAnthropic's research found that artificially increasing the neurons' weights in the "known answer" feature could force Claude to confidently hallucinate information about completely made-up athletes like "Michael Batkin." That kind of result leads the researchers to suggest that "at least some" of Claude's hallucinations are related to a "misfire" of the circuit inhibiting that "can't answer" pathwaythat is, situations where the "known entity" feature (or others like it) is activated even when the token isn't actually well-represented in the training data.Unfortunately, Claude's modeling of what it knows and doesn't know isn't always particularly fine-grained or cut and dried. In another example, researchers note that asking Claude to name a paper written by AI researcher Andrej Karpathy causes the model to confabulate the plausible-sounding but completely made-up paper title "ImageNet Classification with Deep Convolutional Neural Networks." Asking the same question about Anthropic mathematician Josh Batson, on the other hand, causes Claude to respond that it "cannot confidently name a specific paper... without verifying the information." Artificially suppressing Claude's the "known answer" neurons prevent it from hallucinating made-up papers by AI researcher Andrej Karpathy. Credit: Anthropic Artificially suppressing Claude's the "known answer" neurons prevent it from hallucinating made-up papers by AI researcher Andrej Karpathy. Credit: Anthropic After experimenting with feature weights, the Anthropic researchers theorize that the Karpathy hallucination may be caused because the model at least recognizes Karpathy's name, activating certain "known answer/entity" features in the model. These features then inhibit the model's default "don't answer" circuit even though the model doesn't have more specific information on the names of Karpathy's papers (which the model then duly guesses at after it has committed to answering at all). A model fine-tuned to have more robust and specific sets of these kinds of "known entity" features might then be able to better distinguish when it should and shouldn't be confident in its ability to answer.This and other research into the low-level operation of LLMs provides some crucial context for how and why models provide the kinds of answers they do. But Anthropic warns that its current investigatory process still "only captures a fraction of the total computation performed by Claude" and requires "a few hours of human effort" to understand the circuits and features involved in even a short prompt "with tens of words." Hopefully, this is just the first step into more powerful research methods that can provide even deeper insight into LLMs' confabulation problem and maybe, one day, how to fix it.Kyle OrlandSenior Gaming EditorKyle OrlandSenior Gaming Editor Kyle Orland has been the Senior Gaming Editor at Ars Technica since 2012, writing primarily about the business, tech, and culture behind video games. He has journalism and computer science degrees from University of Maryland. He once wrote a whole book about Minesweeper. 19 Comments
0 Comentários
·0 Compartilhamentos
·63 Visualizações