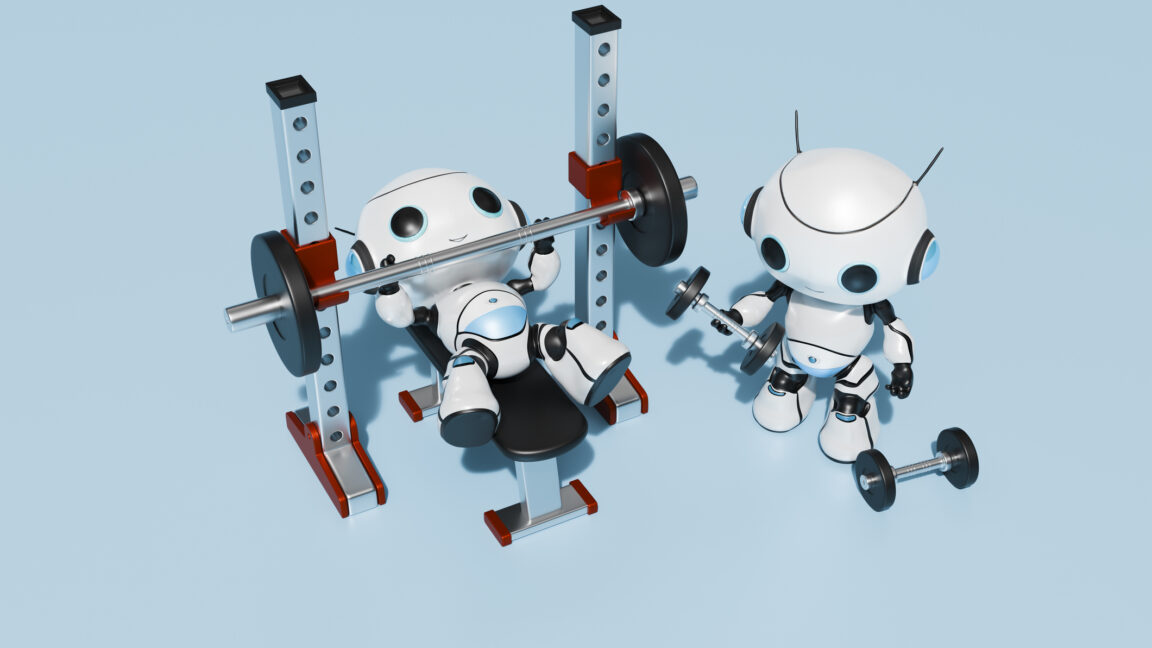
ARSTECHNICA.COM
Microsoft’s “1‑bit” AI model runs on a CPU only, while matching larger systems
Small packages
Microsoft’s “1‑bit” AI model runs on a CPU only, while matching larger systems
Future AI might not need supercomputers thanks to models like BitNet b1.58 2B4T.
Kyle Orland
–
Apr 18, 2025 3:46 pm
|
7
Can big AI models get by with "smaller" weights?
Credit:
Getty Images
Can big AI models get by with "smaller" weights?
Credit:
Getty Images
Story text
Size
Small
Standard
Large
Width
*
Standard
Wide
Links
Standard
Orange
* Subscribers only
Learn more
When it comes to actually storing the numerical weights that power a large language model's underlying neural network, most modern AI models rely on the precision of 16- or 32-bit floating point numbers. But that level of precision can come at the cost of large memory footprints (in the hundreds of gigabytes for the largest models) and significant processing resources needed for the complex matrix multiplication used when responding to prompts.
Now, researchers at Microsoft's General Artificial Intelligence group have released a new neural network model that works with just three distinct weight values: -1, 0, or 1. Building on top of previous work Microsoft Research published in 2023, the new model's "ternary" architecture reduces overall complexity and "substantial advantages in computational efficiency," the researchers write, allowing it to run effectively on a simple desktop CPU. And despite the massive reduction in weight precision, the researchers claim that the model "can achieve performance comparable to leading open-weight, full-precision models of similar size across a wide range of tasks."
Watching your weights
The idea of simplifying model weights isn't a completely new one in AI research. For years, researchers have been experimenting with quantization techniques that squeeze their neural network weights into smaller memory envelopes. In recent years, the most extreme quantization efforts have focused on so-called "BitNets" that represent each weight in a single bit (representing +1 or -1).
BitNet's dad jokes aren't exactly original, but they are sufficiently groan-worthy.
BitNet demo
BitNet's dad jokes aren't exactly original, but they are sufficiently groan-worthy.
BitNet demo
This feels a bit too respectful and cogent for a 2000s-era CPU debate.
BitNet demo
This feels a bit too respectful and cogent for a 2000s-era CPU debate.
BitNet demo
A concise answer that doesn't offer a whole lot of context.
BitNet demo
A concise answer that doesn't offer a whole lot of context.
BitNet demo
This feels a bit too respectful and cogent for a 2000s-era CPU debate.
BitNet demo
A concise answer that doesn't offer a whole lot of context.
BitNet demo
The new BitNet b1.58b model doesn't go quite that far—the ternary system is referred to as "1.58-bit," since that's the average number of bits needed to represent three values (log(3)/log(2)). But it sets itself apart from previous research by being "the first open-source, native 1-bit LLM trained at scale," resulting in a 2 billion token model based on a training dataset of 4 trillion tokens, the researchers write.
The "native" bit is key there, since many previous quantization efforts simply attempted after-the-fact size reductions on pre-existing models trained at "full precision" using those large floating-point values. That kind of post-training quantization can lead to "significant performance degradation" compared to the models they're based on, the researchers write. Other natively trained BitNet models, meanwhile, have been at smaller scales that "may not yet match the capabilities of larger, full-precision counterparts," they write.
Does size matter?
Memory requirements are the most obvious advantage of reducing the complexity of a model's internal weights. The BitNet b1.58 model can run using just 0.4GB of memory, compared to anywhere from 2 to 5GB for other open-weight models of roughly the same parameter size.
But the simplified weighting system also leads to more efficient operation at inference time, with internal operations that rely much more on simple addition instructions and less on computationally costly multiplication instructions. Those efficiency improvements mean BitNet b1.58 uses anywhere from 85 to 96 percent less energy compared to similar full-precision models, the researchers estimate.
A demo of BitNet b1.58 running at speed on an Apple M2 CPU.
By using a highly optimized kernel designed specifically for the BitNet architecture, the BitNet b1.58 model can also run multiple times faster than similar models running on a standard full-precision transformer. The system is efficient enough to reach "speeds comparable to human reading (5-7 tokens per second)" using a single CPU, the researchers write (you can download and run those optimized kernels yourself on a number of ARM and x86 CPUs, or try it using this web demo).
Crucially, the researchers say these improvements don't come at the cost of performance on various benchmarks testing reasoning, math, and "knowledge" capabilities (although that claim has yet to be verified independently). Averaging the results on several common benchmarks, the researchers found that BitNet "achieves capabilities nearly on par with leading models in its size class while offering dramatically improved efficiency."
Despite its smaller memory footprint, BitNet still performs similarly to "full precision" weighted models on many benchmarks.
Despite its smaller memory footprint, BitNet still performs similarly to "full precision" weighted models on many benchmarks.
Despite the apparent success of this "proof of concept" BitNet model, the researchers write that they don't quite understand why the model works as well as it does with such simplified weighting. "Delving deeper into the theoretical underpinnings of why 1-bit training at scale is effective remains an open area," they write. And more research is still needed to get these BitNet models to compete with the overall size and context window "memory" of today's largest models.
Still, this new research shows a potential alternative approach for AI models that are facing spiraling hardware and energy costs from running on expensive and powerful GPUs. It's possible that today's "full precision" models are like muscle cars that are wasting a lot of energy and effort when the equivalent of a nice sub-compact could deliver similar results.
Kyle Orland
Senior Gaming Editor
Kyle Orland
Senior Gaming Editor
Kyle Orland has been the Senior Gaming Editor at Ars Technica since 2012, writing primarily about the business, tech, and culture behind video games. He has journalism and computer science degrees from University of Maryland. He once wrote a whole book about Minesweeper.
7 Comments
0 التعليقات
0 المشاركات
38 مشاهدة