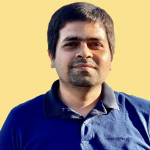
WWW.MARKTECHPOST.COM
A Coding Implementation with Arcad: Integrating Gemini Developer API Tools into LangGraph Agents for Autonomous AI Workflows
Arcade transforms your LangGraph agents from static conversational interfaces into dynamic, action-driven assistants by providing a rich suite of ready-made tools, including web scraping and search, as well as specialized APIs for finance, maps, and more. In this tutorial, we will learn how to initialize ArcadeToolManager, fetch individual tools (such as Web.ScrapeUrl) or entire toolkits, and seamlessly integrate them into Google’s Gemini Developer API chat model via LangChain’s ChatGoogleGenerativeAI. With a few steps, we installed dependencies, securely loaded your API keys, retrieved and inspected your tools, configured the Gemini model, and spun up a ReAct-style agent complete with checkpointed memory. Throughout, Arcade’s intuitive Python interface kept your code concise and your focus squarely on crafting powerful, real-world workflows, no low-level HTTP calls or manual parsing required.
!pip install langchain langchain-arcade langchain-google-genai langgraph
We integrate all the core libraries you need, including LangChain’s core functionality, the Arcade integration for fetching and managing external tools, the Google GenAI connector for Gemini access via API key, and LangGraph’s orchestration framework, so you can get up and running in one go.
from getpass import getpass
import os
if "GOOGLE_API_KEY" not in os.environ:
os.environ["GOOGLE_API_KEY"] = getpass("Gemini API Key: ")
if "ARCADE_API_KEY" not in os.environ:
os.environ["ARCADE_API_KEY"] = getpass("Arcade API Key: ")
We securely prompt you for your Gemini and Arcade API keys, without displaying them on the screen. It sets them as environment variables, only asking if they are not already defined, to keep your credentials out of your notebook code.
from langchain_arcade import ArcadeToolManager
manager = ArcadeToolManager(api_key=os.environ["ARCADE_API_KEY"])
tools = manager.get_tools(tools=["Web.ScrapeUrl"], toolkits=["Google"])
print("Loaded tools:", [t.name for t in tools])
We initialize the ArcadeToolManager with your API key, then fetch both the Web.ScrapeUrl tool and the full Google toolkit. It finally prints out the names of the loaded tools, allowing you to confirm which capabilities are now available to your agent.
from langchain_google_genai import ChatGoogleGenerativeAI
from langgraph.checkpoint.memory import MemorySaver
model = ChatGoogleGenerativeAI(
model="gemini-1.5-flash",
temperature=0,
max_tokens=None,
timeout=None,
max_retries=2,
)
bound_model = model.bind_tools(tools)
memory = MemorySaver()
We initialize the Gemini Developer API chat model (gemini-1.5-flash) with zero temperature for deterministic replies, bind in your Arcade tools so the agent can call them during its reasoning, and set up a MemorySaver to persist the agent’s state checkpoint by checkpoint.
from langgraph.prebuilt import create_react_agent
graph = create_react_agent(
model=bound_model,
tools=tools,
checkpointer=memory
)
We spin up a ReAct‐style LangGraph agent that wires together your bound Gemini model, the fetched Arcade tools, and the MemorySaver checkpointer, enabling your agent to iterate through thinking, tool invocation, and reflection with state persisted across calls.
from langgraph.errors import NodeInterrupt
config = {
"configurable": {
"thread_id": "1",
"user_id": "user@example.com"
}
}
user_input = {
"messages": [
("user", "List any new and important emails in my inbox.")
]
}
try:
for chunk in graph.stream(user_input, config, stream_mode="values"):
chunk["messages"][-1].pretty_print()
except NodeInterrupt as exc:
print(f"\n🔒 NodeInterrupt: {exc}")
print("Please update your tool authorization or adjust your request, then re-run.")
We set up your agent’s config (thread ID and user ID) and user prompt, then stream the ReAct agent’s responses, pretty-printing each chunk as it arrives. If a tool call hits an authorization guard, it catches the NodeInterrupt and tells you to update your credentials or adjust the request before retrying.
In conclusion, by centering our agent architecture on Arcade, we gain instant access to a plug-and-play ecosystem of external capabilities that would otherwise take days to build from scratch. The bind_tools pattern merges Arcade’s toolset with Gemini’s natural-language reasoning, while LangGraph’s ReAct framework orchestrates tool invocation in response to user queries. Whether you’re crawling websites for real-time data, automating routine lookups, or embedding domain-specific APIs, Arcade scales with your ambitions, letting you swap in new tools or toolkits as your use cases evolve.
Here is the Colab Notebook. Also, don’t forget to follow us on Twitter and join our Telegram Channel and LinkedIn Group. Don’t Forget to join our 90k+ ML SubReddit.
Asif RazzaqWebsite | + postsBioAsif Razzaq is the CEO of Marktechpost Media Inc.. As a visionary entrepreneur and engineer, Asif is committed to harnessing the potential of Artificial Intelligence for social good. His most recent endeavor is the launch of an Artificial Intelligence Media Platform, Marktechpost, which stands out for its in-depth coverage of machine learning and deep learning news that is both technically sound and easily understandable by a wide audience. The platform boasts of over 2 million monthly views, illustrating its popularity among audiences.Asif Razzaqhttps://www.marktechpost.com/author/6flvq/Meta AI Introduces Token-Shuffle: A Simple AI Approach to Reducing Image Tokens in TransformersAsif Razzaqhttps://www.marktechpost.com/author/6flvq/A Comprehensive Tutorial on the Five Levels of Agentic AI Architectures: From Basic Prompt Responses to Fully Autonomous Code Generation and ExecutionAsif Razzaqhttps://www.marktechpost.com/author/6flvq/NVIDIA AI Releases OpenMath-Nemotron-32B and 14B-Kaggle: Advanced AI Models for Mathematical Reasoning that Secured First Place in the AIMO-2 Competition and Set New Benchmark RecordsAsif Razzaqhttps://www.marktechpost.com/author/6flvq/Meta AI Releases Web-SSL: A Scalable and Language-Free Approach to Visual Representation Learning
0 Comentários
0 Compartilhamentos
31 Visualizações