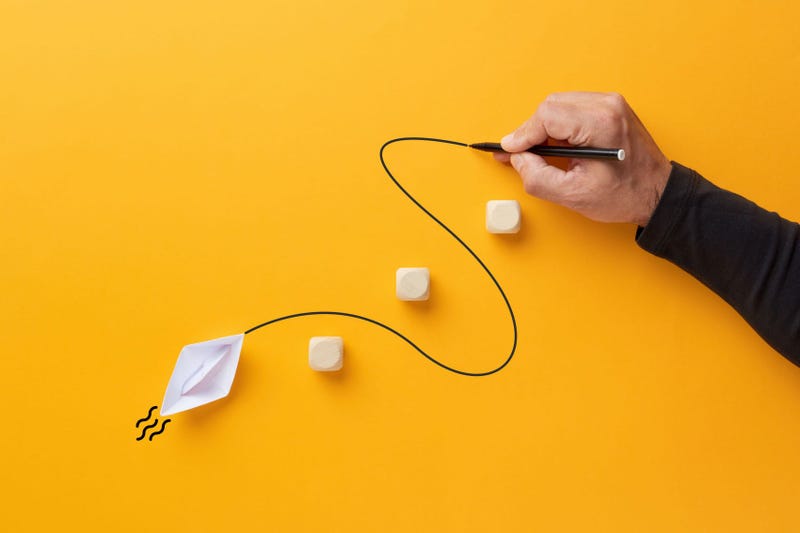
WWW.INFORMATIONWEEK.COM
The CIO's Guide to Managing Agentic AI Systems
As chief information officers, you've likely spent the past few years integrating various forms of artificial intelligence into your enterprise architecture. Perhaps you've implemented machine learning models for predictive analytics, deployed large language models (LLMs) for content generation, or automated routine processes with robotic process automation (RPA). But a fundamental shift is underway that will transform how we think about AI governance: the emergence of AI agents with autonomous decision-making capabilities. The Evolution of AI: From Robotic to Decision-Making The AI landscape has evolved through distinct phases, each progressively automating more complex cognitive labor: Robotic AI: Expert systems, RPAs, and workflow tools that follow rigid, predefined rules Suggestive AI: Machine learning and deep learning systems that provide recommendations based on patterns Instructive AI: Large language models that generate content and insights based on prompts Decision-making AI: Autonomous agents that take action based on their understanding of environments This most recent phase, AI agents with decision-making authority, introduces governance challenges of an entirely different magnitude. Understanding AI Agents: Architecture and Agency Related:At their core, AI agents are systems conferred with agency, the capacity to act independently in a given environment. Their architecture typically includes: Reasoning capabilities: Processing multi-modal information to plan activities Memory systems: Persisting short-term or long-term information from the environment Tool integration: Accessing backend systems to orchestrate workflows and effect change Reflection mechanisms: Assessing performance pre/post-action for self-improvement Action generators: Creating instructions for actions based on requests and environmental context The critical difference between agents and previous AI systems lies in their agency. This is either explicitly provided through access to tools and resources or implicitly coded through roles and responsibilities. The Autonomy Spectrum: A Lesson from Self-Driving Cars The concept of varying levels of agency is well-illustrated by the autonomy classification used for self-driving vehicles: Level 0: No autonomous features Level 1: Single automated tasks (e.g., automatic braking) Level 2: Multiple automated functions working in concert Level 3: "Dynamic driving tasks" with potential human intervention Level 4: Fully driverless operation in certain environments Related:Level 5: Complete autonomy without human presence This framework provides a useful mental model for CIOs considering how much agency to grant AI systems within their organizations. The AI Agency Trade-Off: Opportunities vs Risks Setting the appropriate level of agency is the key governance challenge facing technology leaders. It requires balancing two opposing forces: Higher agency creates greater possibilities for optimal solutions, compared to lower agency when the AI agent is reduced to a mere RPA solution. Higher agency increases the probability of unintended consequences This isn't merely theoretical. Even simple AI agents with limited agency can cause significant disruption if governance controls aren't properly calibrated. As Thomas Jefferson aptly noted, "The price of freedom is eternal vigilance." This applies equally to AI agents with decision-making freedom in your enterprise systems. The Fantasia Parable: A Warning for Modern CIOs Disney's "Fantasia" offers a surprisingly relevant cautionary tale for today's AI governance challenges. In the film, Mickey Mouse enchants a broom to fill buckets with water. Without proper constraints, the broom multiplies endlessly, flooding the workshop in a cascading disaster. Related:This allegorical scenario mirrors the risk of deployed AI agents: they follow their programming without comprehension of consequences, potentially creating cascading effects beyond human control. Looking to the real world and modern times, last year Air Canada's chatbot provided incorrect information about bereavement fares, leading to a lawsuit. Air Canada initially tried to defend itself by claiming the chatbot was a "separate legal entity," but was ultimately held responsible. Also, Tesla experienced several AI-driven autopilot incidents where the system failed to recognize obstacles or misinterpreted road conditions, leading to accidents. The Alignment Problem: Five Critical Risk Categories Alignment -- ensuring AI systems act in accordance with human intentions -- becomes increasingly difficult as agency increases. CIOs must address five interconnected risk categories: Negative side effects: Preventing agents from causing collateral damage while fulfilling tasks Reward hacking: Ensuring agents don't manipulate their internal reward functions Scalable oversight: Monitoring agent behavior without prohibitive costs Safe exploration: Allowing agents to make exploratory moves without damaging systems Distributional shift robustness: Maintaining optimal behavior as environments evolve There is currently a lot of promising work being done by researchers to address alignment challenges that involves algorithms, machine learning frameworks, and tools for data augmentation and adversarial training. Some of these include constrained optimization, inverse reward design, robust generalization, interpretable AI, reinforcement learning from human feedback (RLHF), contrastive fine-tuning (CFT), and synthetic data approaches. The goal is to create AI systems that are better aligned with human values and intentions, requiring ongoing human oversight and refinement of the techniques as AI capabilities advance. Solving the Trade-Off: A Framework for Engendering Trust in AI To capitalize on the transformative potential of agentic AI while mitigating risks, CIOs must enhance their organization's people, processes, and tools: People Re-skill the workforce to appropriately calibrate AI agency levels Redesign organizational structures and metrics to accommodate an agentic workforce. Agents are capable of more advanced workflows, so human capital can progress to higher-value roles. Identifying this early will save companies time and money. Develop new roles focused on agent oversight and governance Processes Map enterprise functions where AI agents can be deployed, with appropriate agency levels Establish governance controls and risk appetites across departments Implement continuous monitoring protocols with clear escalation paths Create sandbox environments for safe testing of increasingly autonomous systems Tools Deploy "governance agents" that monitor enterprise agents Implement real-time analytics for agent behavior patterns Develop automated circuit breakers that can suspend agent activities Build comprehensive audit trails of agent decisions and actions The Governance Imperative: Why CIOs Must Act Now The shift from suggestion-based AI to agentic AI represents a quantum leap in complexity. Unlike LLMs that merely offer recommendations for human consideration, agents execute workflows in real-time, often without direct oversight. This fundamental difference demands an evolution in governance strategies. If AI governance doesn't evolve at the speed of AI capabilities, organizations risk creating systems that operate beyond their ability to control. Governance solutions for the agentic era should have the following capabilities: Visual dashboards: Providing real-time updates on AI systems across the enterprise, their health and status for quick assessments. Health and risk score metrics: Implementing intuitive overall health and risk scores for AI models to simplify monitoring for both availability and assurance purposes. Automated monitoring: Employing systems for automatic detection of bias, drift, performance issues, and anomalies. Performance alerts: Setting up alerts for when models deviate from predefined performance parameters. Custom business metrics: Defining metrics aligned with organizational KPIs, ROI, and other thresholds. Audit trails: Maintaining easily accessible logs for accountability, security, and decision review. Conclusion: Navigating the Agency Frontier As CIOs, your challenge is to harness the transformative potential of AI agents while implementing governance frameworks robust enough to prevent the Fantasia scenario. This requires: A clear understanding of agency levels appropriate for different enterprise functions Governance structures that scale with increasing agent autonomy Technical safeguards that prevent cascading failures Organizational adaptations that enable effective human-agent collaboration The organizations that thrive in the agentic AI era will be those that strike the optimal balance between agency and governance -- empowering AI systems to drive innovation while maintaining appropriate human oversight. Those that ignore this governance imperative may find themselves, like Mickey Mouse, watching helplessly as their creations take on unintended lives of their own.
0 Comments
0 Shares
39 Views