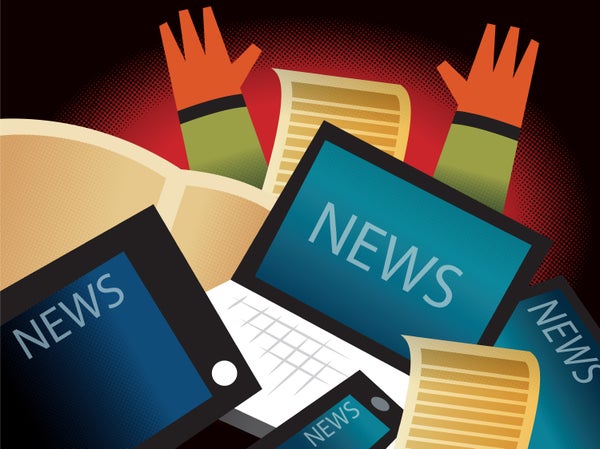
WWW.SCIENTIFICAMERICAN.COM
Misinformation Really Does Spread like a Virus, Epidemiology Shows
November 6, 20245 min readMisinformation Really Does Spread like a Virus, Epidemiology ShowsGoing viral appears to be more than just a catchphrase when it comes to the rampant spread of misinformationMisinformation really does spread like a virus, suggest mathematical models drawn from epidemiology. Paulo Buchinho/Getty ImagesThe following essay is reprinted with permission from The Conversation, an online publication covering the latest research.Were increasingly aware of how misinformation can influence elections. About 73% of Americans report seeing misleading election news, and about half struggle to discern what is true or false.When it comes to misinformation, going viral appears to be more than a simple catchphrase. Scientists have found a close analogy between the spread of misinformation and the spread of viruses. In fact, how misinformation gets around can be effectively described using mathematical models designed to simulate the spread of pathogens.On supporting science journalismIf you're enjoying this article, consider supporting our award-winning journalism by subscribing. By purchasing a subscription you are helping to ensure the future of impactful stories about the discoveries and ideas shaping our world today.Concerns about misinformation are widely held, with a recent UN survey suggesting that 85% of people worldwide are worried about it.These concerns are well founded. Foreign disinformation has grown in sophistication and scope since the 2016 US election. The 2024 election cycle has seen dangerous conspiracy theories about weather manipulation undermining proper management of hurricanes, fake news about immigrants eating pets inciting violence against the Haitian community, and misleading election conspiracy theories amplified by the worlds richest man, Elon Musk.Recent studies have employed mathematical models drawn from epidemiology (the study of how diseases occur in the population and why). These models were originally developed to study the spread of viruses, but can be effectively used to study the diffusion of misinformation across social networks.One class of epidemiological models that works for misinformation is known as the susceptible-infectious-recovered (SIR) model. These simulate the dynamics between susceptible (S), infected (I), and recovered or resistant individuals (R).These models are generated from a series of differential equations (which help mathematicians understand rates of change) and readily apply to the spread of misinformation. For instance, on social media, false information is propagated from individual to individual, some of whom become infected, some of whom remain immune. Others serve as asymptomatic vectors (carriers of disease), spreading misinformation without knowing or being adversely affected by it.These models are incredibly useful because they allow us to predict and simulate population dynamics and to come up with measures such as the basic reproduction (R0) number the average number of cases generated by an infected individual.As a result, there has been growing interest in applying such epidemiological approaches to our information ecosystem. Most social media platforms have an estimated R0 greater than 1, indicating that the platforms have potential for the epidemic-like spread of misinformation.Looking for solutionsMathematical modelling typically either involves whats called phenomenological research (where researchers describe observed patterns) or mechanistic work (which involves making predictions based on known relationships). These models are especially useful because they allow us to explore how possible interventions may help reduce the spread of misinformation on social networks.We can illustrate this basic process with a simple illustrative model shown in the graph below, which allows us to explore how a system might evolve under a variety of hypothetical assumptions, which can then be verified.Prominent social media figures with large followings can become superspreaders of election disinformation, blasting falsehoods to potentially hundreds of millions of people. This reflects the current situation where election officials report being outmatched in their attempts to fact-check minformation.In our model, if we conservatively assume that people just have a 10% chance of infection after exposure, debunking misinformation only has a small effect, according to studies. Under the 10% chance of infection scenario, the population infected by election misinformation grows rapidly (orange line, left panel).A compartment model of disinformation spread over a week in a cohort of users, where disinformation has a 10% chance of infecting a susceptible unvaccinated individual upon exposure. Debunking is assumed to be 5% effective. If prebunking is introduced and is about twice as effective as debunking, the dynamics of disinformation infection change markedly.Sander van der Linden/Robert David GrimesPsychological vaccinationThe viral spread analogy for misinformation is fitting precisely because it allows scientists to simulate ways to counter its spread. These interventions include an approach called psychological inoculation, also known as prebunking.This is where researchers preemptively introduce, and then refute, a falsehood so that people gain future immunity to misinformation. Its similar to vaccination, where people are introduced to a (weakened) dose of the virus to prime their immune systems to future exposure.For example, a recent study used AI chatbots to come up with prebunks against common election fraud myths. This involved warning people in advance that political actors might manipulate their opinion with sensational stories, such as the false claim that massive overnight vote dumps are flipping the election, along with key tips on how to spot such misleading rumours. These inoculations can be integrated into population models of the spread of misinformation.You can see in our graph that if prebunking is not employed, it takes much longer for people to build up immunity to misinformation (left panel, orange line). The right panel illustrates how, if prebunking is deployed at scale, it can contain the number of people who are disinformed (orange line).The point of these models is not to make the problem sound scary or suggest that people are gullible disease vectors. But there is clear evidence that some fake news stories do spread like a simple contagion, infecting users immediately.Meanwhile, other stories behave more like a complex contagion, where people require repeated exposure to misleading sources of information before they become infected.The fact that individual susceptibility to misinformation can vary does not detract from the usefulness of approaches drawn from epidemiology. For example, the models can be adjusted depending on how hard or difficult it is for misinformation to infect different sub-populations.Although thinking of people in this way might be psychologically uncomfortable for some, most misinformation is diffused by small numbers of influential superspreaders, just as happens with viruses.Taking an epidemiological approach to the study of fake news allows us to predict its spread and model the effectiveness of interventions such as prebunking.Some recent work validated the viral approach using social media dynamics from the 2020 US presidential election. The study found that a combination of interventions can be effective in reducing the spread of misinformation.Models are never perfect. But if we want to stop the spread of misinformation, we need to understand it in order to effectively counter its societal harms.This article was originally published on The Conversation. Read the original article.
0 Commentarii
0 Distribuiri
9 Views