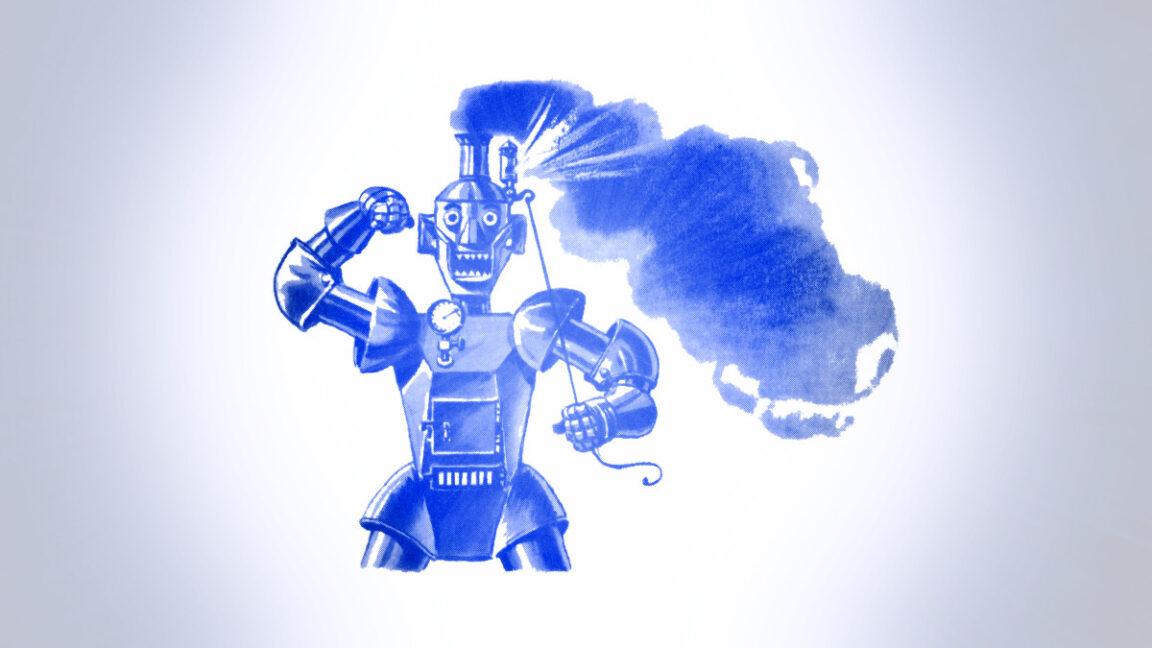
New secret math benchmark stumps AI models and PhDs alike
arstechnica.com
secret math problems dept. New secret math benchmark stumps AI models and PhDs alike FrontierMath's difficult questions remain unpublished so that AI companies can't train against it. Benj Edwards Nov 12, 2024 5:49 pm | 31 Credit: Getty Images Credit: Getty Images Story textSizeSmallStandardLargeWidth *StandardWideLinksStandardOrange* Subscribers only Learn moreOn Friday, research organization Epoch AI released FrontierMath, a new mathematics benchmark that has been turning heads in the AI world because it contains hundreds of expert-level problems that leading AI models solve less than 2 percent of the time, according to Epoch AI. The benchmark tests AI language models (such as GPT-4o, which powers ChatGPT) against original mathematics problems that typically require hours or days for specialist mathematicians to complete.FrontierMath's performance results, revealed in a preprint research paper, paint a stark picture of current AI model limitations. Even with access to Python environments for testing and verification, top models like Claude 3.5 Sonnet, GPT-4o, o1-preview, and Gemini 1.5 Pro scored extremely poorly. This contrasts with their high performance on simpler math benchmarksmany models now score above 90 percent on tests like GSM8K and MATH.The design of FrontierMath differs from many existing AI benchmarks because the problem set remains private and unpublished to prevent data contamination. Many existing AI models are trained on other test problem datasets, allowing the AI models to easily solve the problems and appear more generally capable than they actually are. Many experts cite this as evidence that current large language models (LLMs) are poor generalist learners.Problems spanning multiple disciplinesEpoch AI says it developed FrontierMath through collaboration with over 60 mathematicians from leading institutions. The problems underwent peer review to verify correctness and check for ambiguities. About 1 in 20 problems needed corrections during the review process, a rate comparable to other major machine learning benchmarks.The problems in the new set span multiple mathematical disciplines, from computational number theory to abstract algebraic geometry. And they are reportedly difficult to solve. Really, really difficult.Epoch AI allowed Fields Medal winners Terence Tao and Timothy Gowers to review portions of the benchmark. "These are extremely challenging," Tao said in feedback provided to Epoch. "I think that in the near term basically the only way to solve them, short of having a real domain expert in the area, is by a combination of a semi-expert like a graduate student in a related field, maybe paired with some combination of a modern AI and lots of other algebra packages." A chart showing AI models' limited success on the FrontierMath problems, taken from Epoch AI's research paper. Credit: Epoch AI To aid in the verification of correct answers during testing, the FrontierMath problems must have answers that can be automatically checked through computation, either as exact integers or mathematical objects. The designers made problems "guessproof" by requiring large numerical answers or complex mathematical solutions, with less than a 1 percent chance of correct random guesses.Mathematician Evan Chen, writing on his blog, explained how he thinks that FrontierMath differs from traditional math competitions like the International Mathematical Olympiad (IMO). Problems in that competition typically require creative insight while avoiding complex implementation and specialized knowledge, he says. But for FrontierMath, "they keep the first requirement, but outright invert the second and third requirement," Chen wrote.While IMO problems avoid specialized knowledge and complex calculations, FrontierMath embraces them. "Because an AI system has vastly greater computational power, it's actually possible to design problems with easily verifiable solutions using the same idea that IOI or Project Euler doesbasically, 'write a proof' is replaced by 'implement an algorithm in code,'" Chen explained.The organization plans regular evaluations of AI models against the benchmark while expanding its problem set. They say they will release additional sample problems in the coming months to help the research community test their systems.Benj EdwardsSenior AI ReporterBenj EdwardsSenior AI Reporter Benj Edwards is Ars Technica's Senior AI Reporter and founder of the site's dedicated AI beat in 2022. He's also a widely-cited tech historian. In his free time, he writes and records music, collects vintage computers, and enjoys nature. He lives in Raleigh, NC. 31 Comments
0 Commenti
·0 condivisioni
·136 Views