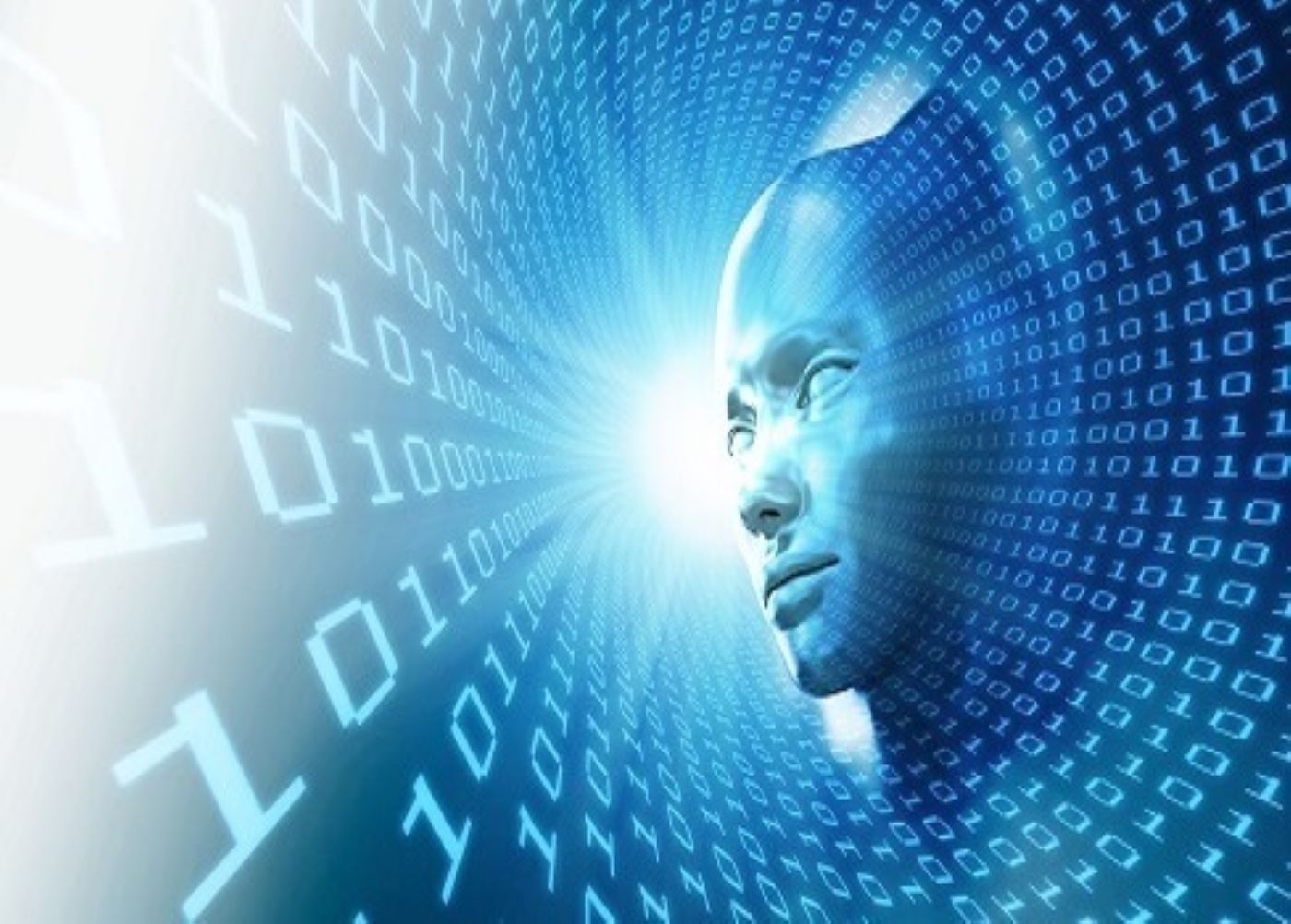
WWW.INFORMATIONWEEK.COM
How Can Decision Makers Trust Hallucinating AI?
Max Belov, Chief Technology Officer, Coherent SolutionsNovember 25, 20244 Min ReadMopic via Alamy StockEvery breakthrough has its share of mistakes. Artificial intelligence is disrupting routine tasks and is quickly establishing itself as a very powerful personal assistant. For example, AI helps medical researchers find and evaluate available donors for cell treatments, giving patients hope where there was none -- and the list of AI uses goes on. Yet, this same technology generates misleading financial forecasts based on non-existent data or creates references to fictitious scientific articles.AI models are only as trustworthy as the data they are trained on. However, even with a solid data foundation, the results of AI predictions are not 100% accurate. The impact of their occasional hallucinations may range from causing slight user embarrassment to billions of dollars worth of financial losses and legal repercussions for organizations. The question is how organizations can look beyond hallucinations and rely on AI in decision-making when the models are partially transparent.AI Confidence Mislead Decision-MakersOver half of Fortune 500 companies note AI as a potential risk factor. They fear AI inconsistencies and potential ethical risks that might lead to negative brand publicity and financial and reputational losses.It is impossible to fix AI hallucinations with a wave of a hand. So far, hallucinations are a common challenge in AI solutions. While the explainability of traditional ML methods and neural networks is well understood by now, many researchers are working on methods to explain GenAI and LLMs. Significant advancements will come in the near future. Meanwhile, AI certainly shouldnt be dismissed because it's not entirely reliable: It has already become a must-have tool for organizations across various industries. Decision-makers should rely on human intelligence and supervision to effectively integrate AI models into business processes.Related:Black Box Trust IssuesAI models are black boxes that lack transparency and are only partially explainable. Hallucinations are common in complex language models and deep learning systems. Such systems are affected since they hinge on patterns derived from vast datasets rather than on a fundamental deterministic understanding of the content.The good news is that taking an insightful look into the black boxes is possible, to a certain extent. Organizations can use specific methods to address one of the major trust issues with AI.Explaining the UnexplainableIn many business applications, especially those influencing critical decision-making, the ability to explain how an AI model reaches its conclusions is more important than achieving the highest possible models accuracy.Related:Not all AI models are black boxes. For example, decision trees or linear regressions are common in predictive analytics, financial forecasting, and business intelligence applications. These types of AI models are interpretable.For non-transparent models, SHAP (shapley additive explanations) helps explain how much each input affects an LLMs prediction. For example, users can ask an LLM to highlight key points in the input data and explain the logical chain behind the output. The answers can help improve system prompts and input data. However, SHAP has limited effectiveness for pre-trained LLMs due to their complexity, which requires different methods to explain their results. This is still a very rapidly developing field, with new emerging approaches for the interpretability of LLMs, such as using attention mechanisms to trace back how a model reaches its conclusion or using LLMs with memory functions to reduce inconsistencies over time.How Can Organizations Rely on AI Models?Organizations should carefully manage and contextualize the reliability of the models they use. Decision-makers can apply guardrails like regular audits and protocols for human oversight. They can consider creating a domain-specific knowledge base, which, for example, will be paramount for medical professionals, as their decisions often impact people's lives. They can also apply theRAGapproach (retrieval augmented generation) to mitigate associated risks. For example, a customer support chatbot can retrieve past interactions with a client, augment that data with product updates, and generate highly relevant responses to resolve a query.Related:Generative AI works best by augmenting human decision-making rather than entirely replacing it. It is important to keep humans in the loop, as they are competent to monitor a models accuracy and ethical compliance. As a rule of thumb, implement GenAI solutions that provide insights while putting human employees in charge of making the final decisions. They can correct and refine the outputs before an AI-driven error grows into a problem.AI models should be dynamic. Feedback loops where humans report issues and introduce changes play a key role in maintaining and enhancing the accuracy and reliability of AI. The next step in aligning AI with organizational processes is in fostering collaboration between data scientists, domain experts, and leaders.Lastly, before investing in GenAI, organizations should conduct a maturity assessment to make sure they have the necessary data infrastructure and robust governance policies in place. They need these to enhance the quality and accessibility of data used to train AI models.AI has great potential to enhance decision-making, but organizations must acknowledge the risks of hallucinations. When they implement consistent measures addressing this issue, they build trust in AI and maximize the benefits of AI solutions.About the AuthorMax BelovChief Technology Officer, Coherent SolutionsMax Belov joined Coherent Solutions in 1998 and assumed the role of CTO two years later. He is a seasoned software architect with deep expertise in designing and implementing distributed systems, cybersecurity, cloud technology, and AI. He also leads Coherents R&D Lab, focusing on IoT, blockchain, and AI innovations. His commentary and bylines appeared in CIO, Silicon UK Tech News, Business Reporter, and TechRadar Pro.See more from Max BelovNever Miss a Beat: Get a snapshot of the issues affecting the IT industry straight to your inbox.SIGN-UPYou May Also LikeReportsMore Reports
0 Commentarii
0 Distribuiri
26 Views