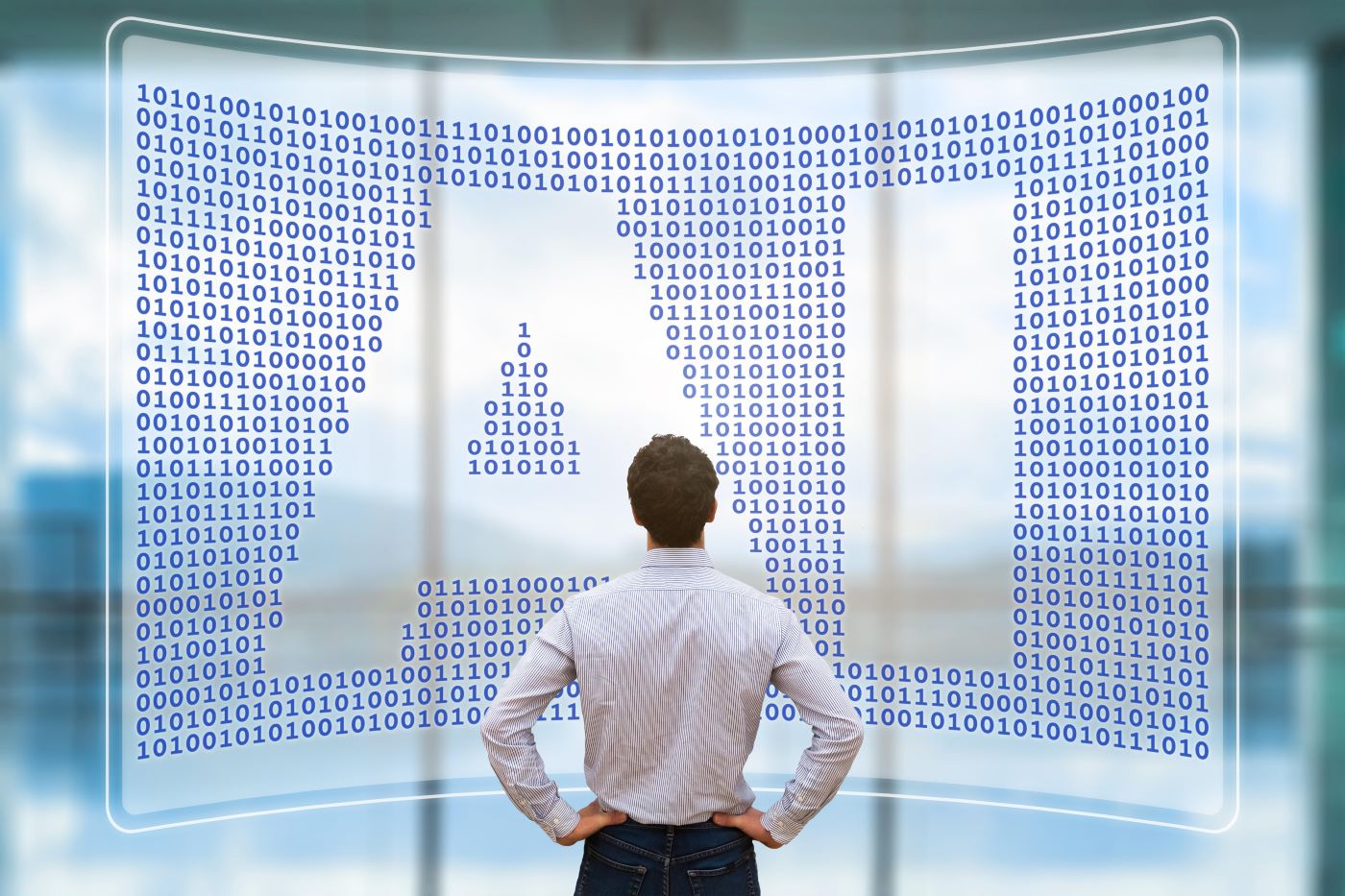
AI Risk Management: Is There an Easy Way?
www.informationweek.com
When ChatGPT commercially launched in 2022, governments, industry sectors, regulators and consumer advocacy groups began to discuss the need to regulate AI, as well as to use it, and it is likely that new regulatory requirements will emerge for AI in the coming months.The quandary for CIOs is that no one really knows what these new requirements will be. However, two things are clear: It makes sense to do some of your own thinking about what your companys internal guardrails should be for AI; and there is too much at stake for organizations to ignore thinking about AI risk.The annals of AI deployments are rife with examples of AI gone wrong, resulting in damage to corporate images and revenues. No CIO wants to be on the receiving end of such a gaffe.Thats why PWC says, Businesses should also ask specific questions about what data will be used to design a particular piece of technology, what data the tech will consume, how it will be maintained and what impact this technology will have on others It is important to consider not just the users, but also anyone else who could potentially be impacted by the technology. Can we determine how individuals, communities and environments might be negatively affected? What metrics can be tracked? Related:Identify a Short List of AI RisksAs AI grows and individuals and organizations of all stripes begin using it, new risks will develop, but these are the current AI risks that companies should consider as they embark on AI development and deployment:Un-vetted data.Companies arent likely to obtain all of the data for their AI projects from internal sources. They will need to source data from third parties.A molecular design research team in Europe used AI to scan and digest all of the worldwide information available from sources such as research papers, articles, and experiments on that molecule. A healthcare institution wanted to use an AI system for cancer diagnosis, so it went out to procure data on a wide range of patients from many different countries.In both cases, data needed to be vetted.In the first case, the research team narrowed the lens of the data it was choosing to admit into its molecular data repository, opting to use only information that directly referred to the molecule they were studying. In the second case, the healthcare institution made sure that any data it procured from third parties was properly anonymized so that the privacy of individual patients was protected.By properly vetting internal and external data that AI would be using, both organizations significantly reduced the risk of admitting bad data into their AI data repositories.Related:Imperfect algorithms.Humans are imperfect, and so are the products they produce. The faulty Amazon recruitment tool, powered by AI and outputting results that favored males over females in recruitment efforts, is an oft-cited example -- but its not the only one.Imperfect algorithms pose risks because they tend to produce imperfect results that can lead businesses down the wrong strategic paths. Thats why its imperative to have a diverse AI team working on algorithm and query development. This staff diversity should be defined by a diverse set of business areas (along with IT and data scientists) working on the algorithmic premises that will drive the data. An equal amount of diversity should be used as it applies to the demographics of age, gender and ethnic background. To the degree that a full range of diverse perspectives are incorporated into algorithmic development and data collection, organizations lower their risk, because fewer stones are left unturned. Poor user and business process training.AI system users, as well as AI data and algorithms, should be vetted during AI development and deployment. For example, a radiologist or a cancer specialist might have the chops to use an AI system designed specifically for cancer diagnosis, but a podiatrist might not.Related:Equally important is ensuring that users of a new AI system understand where and how the system is to be used in their daily business processes. For instance, a loan underwriter in a bank might take a loan application, interview the applicant, and make an initial determination as to the kind of loan the applicant could qualify for, but the next step might be to run the application through an AI-powered loan decisioning system to see if the system agrees. If there is disagreement, the next step might be to take the application to the lending manager for review.The keys here, from both the AI development and deployment perspectives, are that the AI system must be easy to use, and that the users know how and when to use it.Accuracy over time.AI systems are initially developed and tested until they acquire a degree of accuracy that meets or exceeds the accuracy of subject matter experts (SMEs). The gold standard for AI system accuracy is that the system is 95% accurate when compared against the conclusions of SMEs. However, over time, business conditions can change, or the machine learning that the system does on its own might begin to produce results that yield reduced levels of accuracy when compared to what is transpiring in the real world. Inaccuracy creates risk.The solution is to establish a metric for accuracy (e.g., 95%), and to measure this metric on a regular basis. As soon as AI results begin losing accuracy, data and algorithms should be reviewed, tuned and tested until accuracy is restored.Intellectual property risk.Earlier, we discussed how AI users should be vetted for their skill levels and job needs before using an AI system. An additional level of vetting should be applied to those individuals who use the companys AI to develop proprietary intellectual property for the company.If you are an aerospace company, you dont want your chief engineer walking out the door with the AI-driven research for a new jet propulsion system.Intellectual property risks like this are usually handled by the legal staff and HR. Non-compete and non-disclosure agreements prerequisite to employment are agreed to. However, if an AI system is being deployed for intellectual property purposes, it should be a bulleted check point on the project list that everyone authorized to use the new system has the necessary clearance.
0 التعليقات
·0 المشاركات
·57 مشاهدة