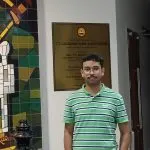
Google AI Introduces Parfait: A Privacy-First AI System for Secure Data Aggregation and Analytics
www.marktechpost.com
Protecting user data while enabling advanced analytics and machine learning is a critical challenge. Organizations must process and analyze data without compromising privacy, but existing solutions often struggle to balance security with functionality. This creates barriers to innovation, limiting collaboration and the development of privacy-conscious technologies. A solution that ensures transparency minimizes data exposure, preserves anonymity, and allows external verification is needed. Addressing these challenges makes it possible to unlock new opportunities for secure and privacy-first computing, enabling businesses and researchers to collaborate effectively while maintaining strict data protection standards.Recent research has explored various privacy-preserving techniques for data aggregation, model training, and analytics. Differential privacy has been widely adopted to add noise to datasets, ensuring individual data points remain unidentifiable. Federated learning allows models to be trained across decentralized devices without sharing raw data, enhancing security. Additionally, trusted execution environments (TEEs) provide hardware-based security for private computations. Despite these advancements, existing methods often involve trade-offs between accuracy, efficiency, and privacy, highlighting the need for more robust, scalable, and verifiable privacy-first solutions.Researchers from Google introduced a new approach, Parfait, designed to enhance privacy-first computing by integrating multiple privacy-preserving techniques into a unified framework. It prioritizes transparency by offering clear insights into data usage and processing methods. It incorporates federated learning, federated analytics, and secure aggregation to minimize data exposure, allowing computations to occur locally without transferring raw data. Additionally, it employs differential privacy algorithms for tasks like model training and analytics, ensuring sensitive information remains anonymized. By combining these techniques, Parfait enables secure data handling while maintaining accuracy and efficiency.Another key aspect of Parfait is external verifiability, which ensures that privacy claims can be independently verified. TEEs are utilized to create secure workflows where computations can be audited without compromising confidentiality. This enhances trust among users and organizations by ensuring that privacy protocols are upheld. Parfait fosters a collaborative space and enables businesses and open-source projects to innovate securely while adhering to strict privacy principles. Its comprehensive design aims to address existing challenges in privacy-preserving computation, striking a balance between data security, accessibility, and performance.The results demonstrate that Parfait effectively enhances privacy-preserving computing by ensuring secure data aggregation, retrieval, and analysis. It successfully maintains data confidentiality while enabling collaborative innovation across various domains. Using federated learning and differential privacy techniques minimizes the risk of privacy breaches. Additionally, trusted execution environments provide verifiability, reinforcing user trust. The framework balances privacy and efficiency, proving its capability to handle tasks like model training, analytics, and secure computation. These findings highlight Parfaits potential to set a new standard for privacy-first computing, making it a valuable tool for businesses and open-source projects.In conclusion, Parfait introduces a robust framework for privacy-preserving computing, enabling secure data aggregation, retrieval, and analytics without compromising confidentiality. Integrating advanced privacy techniques such as federated learning, differential privacy, and trusted execution environments ensures transparency, minimizes data exposure, and enhances security. The results highlight its effectiveness in balancing privacy with computational efficiency, making it a tool for businesses and open-source communities. Parfait sets the stage for future innovations in privacy-first computing, paving the way for more secure, verifiable, and collaborative AI applications that respect user data while enabling meaningful insights and advancements.Check out the Technical Details and GitHub Page. All credit for this research goes to the researchers of this project. Also,dont forget to follow us onTwitter and join ourTelegram Channel andLinkedIn Group. Dont Forget to join our75k+ ML SubReddit.(Promoted) Sana HassanSana Hassan, a consulting intern at Marktechpost and dual-degree student at IIT Madras, is passionate about applying technology and AI to address real-world challenges. With a keen interest in solving practical problems, he brings a fresh perspective to the intersection of AI and real-life solutions.Sana Hassanhttps://www.marktechpost.com/author/sana-hassan/Exploration Challenges in LLMs: Balancing Uncertainty and Empowerment in Open-Ended TasksSana Hassanhttps://www.marktechpost.com/author/sana-hassan/Creating an AI-Powered Tutor Using Vector Database and Groq for Retrieval-Augmented Generation (RAG): Step by Step GuideSana Hassanhttps://www.marktechpost.com/author/sana-hassan/Mistral AI Releases the Mistral-Small-24B-Instruct-2501: A Latency-Optimized 24B-Parameter Model Released Under the Apache 2.0 LicenseSana Hassanhttps://www.marktechpost.com/author/sana-hassan/Agentic AI: The Foundations Based on Perception Layer, Knowledge Representation and Memory Systems [Recommended] Join Our Telegram Channel
0 Reacties
·0 aandelen
·20 Views