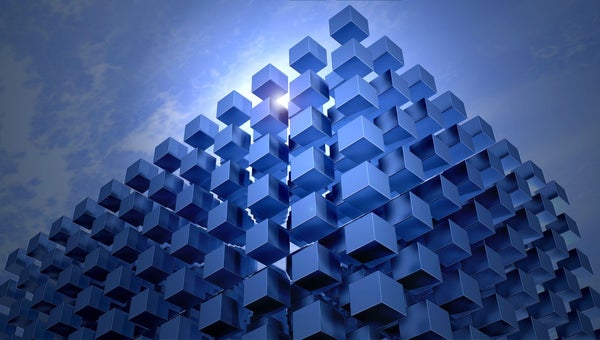
Googles DeepMind AI Can Solve Math Problems on Par with Top Human Solvers
www.scientificamerican.com
February 10, 20253 min readGoogles AI Can Beat the Smartest High Schoolers in MathGoogles AlphaGeometry2 AI reaches the level of gold-medal students in the International Mathematical OlympiadBy Davide Castelvecchi & Nature magazine Google DeepMinds AI AlphaGeometry2 aced problems set at the International Mathematical Olympiad. Wirestock, Inc./Alamy Stock PhotoA year ago AlphaGeometry, an artificial-intelligence (AI) problem solver created by Google DeepMind, surprised the world by performing at the level of silver medallists in the International Mathematical Olympiad (IMO), a prestigious competition that sets tough maths problems for gifted high-school students.The DeepMind team now says the performance of its upgraded system, AlphaGeometry2, has surpassed the level of the average gold medallist. The results are described in a preprint on the arXiv.I imagine it wont be long before computers are getting full marks on the IMO, says Kevin Buzzard, a mathematician at Imperial College London.On supporting science journalismIf you're enjoying this article, consider supporting our award-winning journalism by subscribing. By purchasing a subscription you are helping to ensure the future of impactful stories about the discoveries and ideas shaping our world today.Solving problems in Euclidean geometry is one of the four topics covered in IMO problems the others cover the branches of number theory, algebra and combinatorics. Geometry demands specific skills of an AI, because competitors must provide a rigorous proof for a statement about geometric objects on the plane. In July, AlphaGeometry2 made its public debut alongside a newly unveiled system, AlphaProof, which DeepMind developed for solving the non-geometry questions in the IMO problem sets.Mathematical languageAlphaGeometry is a combination of components that include a specialized language model and a neuro-symbolic system one that does not train by learning from data like a neural network but has abstract reasoning coded in by humans. The team trained the language model to speak a formal mathematical language, which makes it possible to automatically check its output for logical rigour and to weed out the hallucinations, the incoherent or false statements that AI chatbots are prone to making.For AlphaGeometry2, the team made several improvements, including the integration of Googles state-of-the-art large language model, Gemini. The team also introduced the ability to reason by moving geometric objects around the plane such as moving a point along a line to change the height of a triangle and solving linear equations.The system was able to solve 84% of all geometry problems given in IMOs in the past 25 years, compared with 54% for the first AlphaGeometry. (Teams in India and China used different approaches last year to achieve gold-medal-level performance in geometry, but on a smaller subset of IMO geometry problems.)The authors of the DeepMind paper write that future improvements of AlphaGeometry will include dealing with maths problems that involve inequalities and non-linear equations, which will be required to to fully solve geometry.Rapid progressThe first AI system to achieve a gold-medal score for the overall test could win a US$5-million award called the AI Mathematical Olympiad Prize although that competition requires systems to be open-source, which is not the case for DeepMind.Buzzard says he is not surprised by the rapid progress made both by DeepMind and by the Indian and Chinese teams. But, he adds, although the problems are hard, the subject is still conceptually simple, and there are many more challenges to overcome before AI is able to solve problems at the level of research mathematics.AI researchers will be eagerly awaiting the next iteration of the IMO in Sunshine Coast, Australia, in July. Once its problems are made public for human participants to solve, AI-based systems get to solve them, too. (AI agents are not allowed to take part in the competition, and are therefore not eligible to win medals.) Fresh problems are seen as the most reliable test for machine-learning-based systems, because there is no risk that the problems or their solution existed online and may have leaked into training data sets, skewing the results.This article is reproduced with permission and was first published on February 7, 2025.
0 Commentarios
·0 Acciones
·56 Views