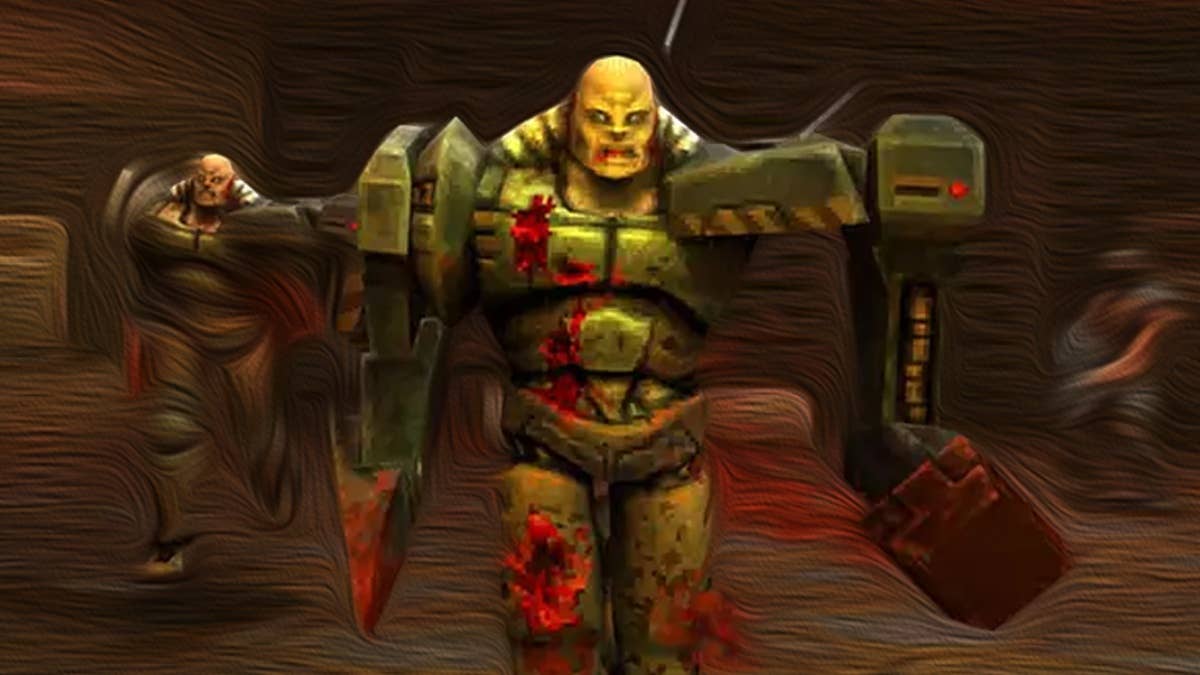
WWW.EUROGAMER.NET
Inside Muse, Microsoft's gen-AI game tool everyone hates - what's really going on and why is it being marketed at Xbox fans?
Inside Muse, Microsoft's gen-AI game tool everyone hates - what's really going on and why is it being marketed at Xbox fans?
If Muse isn't creating games, then what is it creating - and how should we respond?
Image credit: id Software / Eurogamer
Feature
by Chris Tapsell
Deputy Editor
Published on April 16, 2025
Earlier this month, Microsoft set loose upon the world a new, publicly-available demo of its video game-focused generative AI model, Muse. As far as demos go, it was a significant one: a playable, browser-based "AI rendition" of legendary first-person shooter Quake 2.
But rather than landing as, say, an impressive demonstration of Microsoft's bounding progress in artificial intelligence, the Quake 2 "Copilot gaming experience" was widely slammed by the gaming public as, amongst many other things, "shit".
The criticism has been more or less universal, ranging from members of the public describing it as "absolutely fucking disgusting" and "like horrible Nyquil-induced sleep paralysis", to a Forbes "review" of the game that called it "an abomination". Members of the games press condemned reporting in The Verge, which ran the headline "Microsoft has created an AI-generated version of Quake", as overly credulous, labelling any similar coverage as "unpaid PR work".
Will Smith, a tech blogger, offered a more measured response but still concluded that it is ultimately "a gimmick. It's interactive video, and yeah, I guess that's impressive, but it doesn't capture the magic of interlocking systems that still makes playing Quake 2 fun in 2025, despite the fact that it's almost 30 years old and dates to the earliest days of 3D graphics."
All of this comes just a couple of weeks after Microsoft's initial reveal of Muse itself, which that time featured non-interactive but similarly murky footage based on Ninja Theory's Bleeding Edge - and which received a similarly heated response.
That first unveiling also came with further issues of its own. Reactions zeroed in on the notion of Muse being used for "gameplay ideation" in particular, as well as Xbox chief Phil Spencer's seemingly outlandish claim, in an accompanying video, that Muse could "radically change how we preserve and experience classic games in the future," making older games compatible with "any device".
There is intense scepticism towards what Muse AI does, what it could do, and whether it could ever be useful for anything.
It was a messy reveal. At the time, we clarified - with the help of AI expert Dr Michael Cook - that Muse very much was not "creating games". As Cook laid out in his blog, the research paper Microsoft had published in Nature alongside the reveal of this model "is not really about 'generating gameplay' or 'ideas'," but is instead, "about these researchers thinking about the implications of how people will work with these tools." But that didn't mean there weren't plenty of limitations and concerns. On Spencer's comments on preservation, for instance, Cook noted: "I could ask my friend's five-year-old son to draw a crayon picture of what he thinks the ending cutscene of Final Fantasy 8 looks like and that would still count as game preservation of a certain sort."
All in all, it leads to what is on the surface an utterly bizarre situation. Microsoft, one of the world's wealthiest businesses, is committing presumably vast sums of money towards the work of its Muse AI research team. The research team features a number of experts, such as Dr Sam Devlin and research lead Dr Katja Hofmann, that are well-known and widely cited in their field. And the early results of this research are something that almost everyone in the industry seems to totally despise. There is intense scepticism towards what Muse AI does, what it could do, and whether it could ever be useful for anything.
But what is actually going on? Can the research team behind it offer more credible answers? And how, exactly, ought we react to all of it?
What is Muse?
To help understand, I spoke to two of the people who have been at the heart of the conversation around Muse since its reveal: Drs Mike Cook and Katja Hofmann.
Cook is a senior lecturer in computer science at King's College London and specialises in artificial intelligence, specifically computational creativity, automated game design, and design and analysis of generative software. We've covered his work on Eurogamer a few times over the years, including an AI program he built, ANGELINA, which was capable of creating video games of its own back in 2013, and his quest to see if it could win a game jam the following year. He talks with the soft directness of a fast-moving lecturer who doesn't mind if you really can't keep up with the source material, as long as you're at least willing to keep trying.
Speaking to me after the first Muse reveal, but before the second featuring Quake 2, I asked Cook to explain what was going on in the Bleeding Edge examples we were first given. "I suppose you could think of it as: if you showed ChatGPT a screenshot of a video game, and then you said, "Could you show me a video of what would happen if there was an enemy here", and it generates a video showing you the player playing from that screenshot, but with an enemy on the screen," he says. That's a "very clumsy" summary, he adds, "but basically that's what they're trying to do."
Bleeding Edge failed to find an audience, but it's played a large part in the Muse project at Microsoft. | Image credit: Ninja Theory / Xbox
A key point to emphasise here is, as Cook says, that "it's generating images. It's not generating code or anything like that." It's a series of images forming a video, and that video is what's known as being "conditioned", in AI research terms. "It's conditioned on inputs," Cook explains, in the same way that an image generation tool such as Midjourney "is image generation conditioned by text." If you tell an AI image generator you want an image of a cat, for instance, "it generates an image and then it looks at the text and it's like, this can't be any image; it has to be an image of a cat."
With Muse, that conditioning is "on not just the video of people playing the game, but actually the buttons they were pressing." This means that when we talk about the 'playable' models, such as this latest version of Muse, and Google's similarly controversial Genie, "it is doing video generation, but you can also say "Generate the next frame of the video if the jump button was being pressed," and it will take that into account when it generates the next frame." The key point here is that this all remains fundamentally predictive, in the same way that large language models (LLMs) such as ChatGPT are ultimately predicting sequences of words based on your text prompts.
Muse is a model that generates simulated video footage of the video game it's been trained on, and simulates interactivity with that footage
I put the same explain-this-to-me-like-the-layperson-I-am question to Hofmann, who is senior principal researcher and the lead of Microsoft's game intelligence research team. Softly spoken, with a slight German accent, Hofmann is a precise talker, measuring words in her combination of seasoned public spokesperson familiar with the conference trail, and excitable research grad who still believes in the work. "In simple terms," she says, the first model "shows we can train an AI simulation of an existing video game. So that means if we train a model on the game visuals and the control actions, we learn a model that is then able to simulate this."
So, she continues, "you create a small, playable scene, that's only backed then at inference time by the model," inference time being the duration it takes for the model to process new, unseen data and make predictions on it. In the "real time" version of this model, then, this actually "allows players to connect the controller and play within the imagination - or play within the model."
In even more simple terms, think of it like this: Muse is a model that generates simulated video footage of the video game it's been trained on, and simulates interactivity with that footage by predicting what your button presses would cause that video footage to do, and then replicating those. So if, for example, all of the Bleeding Edge footage used as training data shows that when players push the right analogue stick upwards in Bleeding Edge, the camera looks upwards, then when "playing the model", pushing the analogue stick upwards likewise causes the simulated video feed to tilt upwards towards the sky.
Is Muse generating video games?
All this notion of "playing" Muse raises one particularly important question: is Muse generating video games? The emphatic answer from both Hofmann and Cook is, essentially: no.
For Cook, there's a question about whether it's even accurate to describe Muse, and similar models such as Genie or Oasis, as "playable" at all. "On YouTube you can watch panoramic video and turn your head around, but that's not "playable" video. That's just a video that has some interaction with it." At the same time, he says, "if you think about those streaming services where you were playing at home and your keyboard inputs were being sent to a server," such as say, Xbox Cloud Gaming, Nvidia GeForce Now, Amazon Luna or the doomed Google Stadia, "your computer was just showing you video. And the video was kind of conditioned on keyboard inputs."
It's best to think of it instead as "video of a 3D world, but the 3D world doesn't really exist." Whether that does or doesn't disqualify it from actually being a game is up for debate. As Cook himself says, "it gets a bit philosophical at some point." (An example of a natural follow-on question that's either fascinating or incredibly boring to you, depending on your persuasion: does a video game world defined by fixed code "exist"? Does it exist on your hardware more than a streamed one from a server? Or, as in Muse's case, a very murky, inconsistent one conjured on the spot from no code at all?)
This is Quake 2 as created by Muse. It clearly suffers in comparison to the original game, but is that really the point? | Image credit: Microsoft
During our initial conversation, Hofmann extended an invitation to visit the Microsoft AI research team's offices in Cambridge in order to try out a demo of Muse in person. There, she elaborated further on this notion of playing the model, rather than its attempt at recreating a game.
"We're trying not to refer to it as a game, because it is not a game," Hofmann tells me during the in-person demo, which this time takes place after the reveal of the Quake 2 simulation. "We're thinking of "playing the model", so that exploratory kind of interaction of figuring out: What are the limitations? Where does it break? What doesn't work, what works?" To Hofmann, it's a process she feels is "fascinating," albeit joking that said fascination might be reserved for a very specific subset of researchers who share her enthusiasm.
In fact to Hofmann, testing the boundaries of where the model breaks is in many ways part of the point of publishing the Quake 2 demo, and indeed creating it in the first place. She compares it to other, non-interactive generative AI favourably in that sense, in that with attempting to replicate video games you can simply find the flaws much faster. "If you interact with a chatbot, you never get that immediate experience of: I can essentially see where data is missing, or where it's making mistakes. Here, because we're so wired for 3D interaction and visual interaction, we get that immediate experience of: you can literally debug the model by going in and playing within this environment," she says. "And to me, that is the key fascinating aspect of what we're able to show here."
Having "played the model" myself both via the online, browser-based Quake 2 Copilot demo and again in person, via an Xbox controller connected to Hofmann's laptop, this notion does have an element of truth to it. I played an updated version of the Bleeding Edge-based simulation alongside the Quake 2 one - both based on WHAMM (note the second M), which is the more recent version that makes the Quake 2 demo possible - and also tested out the drag-and-drop functionality of adding interactable items, jump pads, and enemies in real time.
Quake 2 was remastered recently. This handy footage serves as a good comparison for what the game ought to be like to play.Watch on YouTube
It's worth making very clear up front that both of them are, as video games, utterly terrible, for all the many reasons already detailed at length and with far more satisfying bite elsewhere than I could ever conjure: they're fuzzy, laggy, nightmarish non-places, chugging along at nine frames per second, crucially without much in the way of consistent internal logic, let alone meaning or intent. They are, by every measure of playability, unplayable.
However, treated as a kind of meta-game, where the challenge isn't within the game but in just attempting to successfully play it at all, there is something at least novel and interesting here. In one moment of quasi-Quake 2, for instance, I found myself stuck in a dark, underwater secret area with no apparent way out, not helped by the vagueness and inconsistency of the visuals and controls. Then I realised I could "play the model" to escape, effectively gaming its 0.9 second memory by looking at a dark wall for a moment, then turning back around to discover the world had totally changed and a route was now clear.
These are, in their own ways, the kinds of consistent rules we need to make something a game, learned in the same way we learn any other mechanic or moment of internal game logic: if I do X, Y happens. That, technically, is a video game. And arguably an interesting one, if only given its novelty and context, much the same way as an out-there art gallery installation can be when you're only interacting with it once or twice. But also still not a particularly good one.
That also ties into the natural follow-on question here, which asks what the actual point of this model is, and what its uses might or might not eventually be.
"Gameplay ideation", preservation, or something else: who and what is Muse for?
If the question of whether or not Muse's synthetic creations are video games seems a tad murky, this next one's about as clear as mud. Who, or what, is Muse actually for? Again, this question rapidly takes on a philosophical form as soon as you begin to prod at it, but before we get to the real chin-stroking it makes sense to run through the proposed uses that've already been suggested so far.
One of the prominent, and most easy to dismiss, is this notion of preservation touted by Phil Spencer. Hofmann, it's worth noting, was on that slightly odd, video call-style reveal video with Spencer when he raised this as a possibility. I asked her whether she felt that was a genuinely feasible use for Muse, now or at any point in the future.
"What I see at this point in time is that we're having so many conversations on what could be the potential branch-out points into real applications," she said, "and game preservation has been one of them that multiple people have brought up. And one that Phil, for example, was particularly excited about."
The famous Halo 2 E3 demo has actually been preserved and is playable on PC. If Muse is ever capable of doing similar, it's many years away. | Image credit: Microsoft
However, she continued, "I see this as one of the capabilities of not something that is, in any full form, feasible today. I like to ground it in: what's feasible today is I can create a simulation of a level in a game, that gives you a sense of playing a version of that game, that is not exactly like the real game."
"My sense is that over the next decade, this technology will mature," she added, noting that she felt it "perfectly feasible" to believe it could eventually be "a very general way" of preserving a video game in some form. "I'm certain it won't be the only one. But I see that. I do see it as something that could be exciting to explore along with other application areas."
Cook is unequivocal here, meanwhile. "Think about your favourite game, and think about the footage of it that's on YouTube," he said. "If we were going to learn the game based on that footage, what stuff isn't seen very often? What stuff is maybe not seen at all? Maybe there's secrets no one's ever found. Bugs, glitches.
"And also on maybe a stupider kind of level - but still important to think about - is things like when dataminers find cut content from Elden Ring. Or how if you look in very old games, you can find comments in the codebase from developers who are working at 4:00am and writing messages in the code. That stuff obviously can't be locked, can't be recovered, because the computer can't see it."
An analogy he offers here: "The Globe Theatre that I walk past is not the real Globe Theatre. It's still useful that we built it. But it would be great if we also had the original." Hypothetically, he adds, even if this method got "99 percent of the way there, ultimately it isn't the same as actually recording the code base, for a million and one reasons."
The next suggestion here feels like less a piece of executive ad-libbing, and more a central to Microsoft's core pitch for Muse: "gameplay ideation" - a rather corporate term for, essentially, thinking up stuff you can do in a game. Muse is explicitly being touted as a potential tool for helping with this, but crucially, and as we've already clarified, it's not coming up with ideas or inventing gameplay itself in any way.
"There are philosophical questions around: can models be creative?" Hofmann said, when I asked her to clarify. "And I'm quite firmly on the side that they are not. I've seen a lot of confusion around that in the literature. But by framing Muse as: it is a simulation of an existing video game, I think that quite firmly emphasises that the model in itself is not creative."
"I can't tell you specifically which innovation is going to come after the other, but it's basically opened up this huge, huge space… I cannot see the end of how far we're going to be able to get with that."
What the research team was instead aiming to achieve, after conversations with developers internally at Microsoft, "was exactly to tease out the model capabilities that could unlock human creativity." In other words, the team is attempting to figure out how models like this, if they were to become faster, more efficient, more accurate and so on, might be useful to human developers in the future.
In this case, it comes back to the initial demonstration of Muse with Bleeding Edge, where you could drag and drop something into the game - a jump pad, an explosive barrel, an enemy - and then quickly see how that plays out.
Is that actually feasible as a method of seriously prototyping game ideas, and even if it was, would it actually be helpful? With the first question, Hofmann describes the output of the current version of the model as "not-fully-working" and for now only an example of "signs of life", but despite plenty of scepticism from the watching public, her confidence in its ability to improve seems ironclad.
That confidence is based on what Hofmann believes to be genuine discoveries in how these models can operate, she explains, such as gaining an understanding of "how we can curate data to craft - or to train - models that capture those structural relationships in the data." By "structural relationships", she adds, what she specifically means is "the understanding of how this model is able to translate an image into numbers, and then learn how those numbers relate to each other." In the simplest possible terms, Hofmann feels the team has learned how being specific with training data in certain ways can give you much more reliable outputs, and with less data required.
That newfound understanding has "opened up this really big space around how specifically curated data sets and multi-modal models interact with each other, and the kinds of structures they're able to learn." When does that potential become actual, usable reality for some kind of developer-friendly jump pad-testing tool? There's "a lot of runway", she says, though "I can't give you a timeline. I can't tell you specifically which innovation is going to come after the other, but it's basically opened up this huge, huge space… I cannot see the end of how far we're going to be able to get with that."
Xbox has actually done more for preservation than most platform holders, with some great results on Xbox One and Xbox Series consoles.Watch on YouTube
Whether or not this might actually be useful to developers is another issue, however. Cook, for his part, is sceptical again, suggesting more immediately helpful (and efficient) avenues for this kind of tool might be found in quickly adding a splash of a game's visuals and other details to standard whiteboxing, or adding new forms of immediate, quickfire automatic playtesting that don't currently exist. "Human playtesters exist," he says, "but there are some techniques that require you to have an automatic playtester."
Nevertheless, Cook is keen to praise Microsoft for having actually sought input from real game developers, something that Hofmann also repeatedly points to. "One of my favourite things about the paper is that they actually sat down with developers and talked to them," Cook says, "and often they talked of developers that were already very positive about the technology - but they still actually said to them: What would a workflow that looks useful for you be? I thought that was really important and we need more of that stuff."
One other, important question comes up here, when it comes to the practical implementation of this kind of hypothetical tool. How could a developer use Muse to simulate adding a new mechanic to an in-development game, when doing so currently requires training Muse on, as in Bleeding Edge's case, about 7000 hours of the already-finished game's footage?
Hofmann argues the team has indeed thought of this, and it came up in the conversations the research team had with developers. "We look at: assume a scenario where let's say someone has built out a first level of a new game that they're working on - how little data might they need to train a model like this?" The team is "nowhere near the full version of making that real," she said, "but we now know that we can get away with as little as about 50 hours of gameplay from a given game level to create a really nice, consistent representation in that."
The very fact that there is so much uncertainty over possible uses for Muse, however, leads on to yet another, regularly posed query. Isn't this all being done back to front?
Finding solutions before problems - and the problem with doing research in public
"A solution looking for a problem" is a criticism regularly levied at all kinds of AI ventures, particularly generative AI ones. In many cases it feels entirely justified. AI-generated video and images, such as those produced by Midjourney and Sora, have thus far failed to find any real purpose beyond generating low-effort memes, objectionable propaganda and disinformation, or unanimous criticism whenever used in relation to a video game.
Generative text, such as ChatGPT, has fared better in terms of gaining weekly users, which have landed in the hundreds of millions, but poorly in terms of actually making any money from that (the vast majority of those users use it for free, and using it costs OpenAI a huge amount of money). Text-based gen-AI's main uses seem to remain helping Instagram influencers and spammers to rapidly fill out inessential image captions to game the algorithm, meanwhile, or disrupting more trustworthy Google search results with such valuable advice as eating rocks for good health.
Naturally, that same criticism was levied again at Muse after its reveal of the Quake 2 demo. Sos Sosowski, an indie developer, issued one of the most widely-shared putdowns on BlueSky with exactly this. "That's very on-track with [the] AI trend," he wrote. "A solution looking for a problem. It's yet another in a series of "reveals" that is bug-ridden and broken."
AI, when used in media or video games, is generally pushed back on heavily by fans and consumers. The trailer for Ark Aquatica found this out in March. | Image credit: Snail
In talking to Cook and Hofmann, who both work in very different areas of AI-based academic research, I was particularly curious about this. How does the actual timeline of research unfold here? Whose idea was Muse? How did it begin, or change over time? And is it normal to do things this way round, in the academic world, in researching technology first and worry about finding a use for it later?
"What used to happen at Microsoft Research Cambridge - I used to know someone who worked there," Cook says, "and they used to joke that they felt like Microsoft didn't know they existed, and in a good way. Because they didn't ask what they were doing with their money, and so the researchers there would just do whatever they were interested in and had a great time doing it."
The one exception to that? "The Xbox team that was there - and I think over time, there's been more scrutiny on what the AI teams are doing."
He has a few suggestions as to how this initial research might have come to be. Sometimes, he explains, "ideas like this are born out of a single researcher or a hackathon day, or a conversation over coffee." Likewise, "it might have been born out of something more specific," like say, if there's a large data source that's already available. "So it's like: listen, we've got 400,000 hours of people playing this game, there must be something we can do with it."
I put the same question to Hoffman, who for her part offered a detailed explanation which seems to quite closely echo some of Cook's best guesses. "Microsoft Research is quite unique, I would say, in that it's a very bottom-up research organisation," she explains.
"Our remit is to drive innovation, to drive the start of the art in our respective areas." In practical terms that means combining their research into machine learning with, for instance, "leveraging the rich data that we can in many cases responsibly obtain in video games, where we might be able to get to a scale or a variety that might be very, very difficult to collect in any other application area." That'll be the readily-available data theory, then, and in this case that's down to the End User License Agreement (EULA) that all players agree to when playing games on Xbox. Under that often-ignored agreement, Xbox was able to gather the video data of, for instance, Bleeding Edge gameplay that it used to train its model.
This combination of the research area and the available data then melds with the "luxury" of Microsoft being a large, multidisciplinary company. That grants the research team the "opportunity of engaging with the rest of the company and seeing what's on people's minds," as Hofmann puts it, "and so we have those regular conversations with people in the gaming space to understand what limitations, what challenges they're facing. Where do they see things going?" The research team itself then decides, "within the team", how to direct its research. In this case, Hofmann says the notion of something to help with "ideation" was one that came more or less directly from conversations with developers.
"Many of them felt that because game development is so expensive, and prototyping is expensive, everyone who mentioned it felt like they did not have the luxury of doing enough ideation and prototyping - which they felt limited the creativity of what ultimately got built," she explained.
There were in fact "multiple points" during the project where the team almost stopped work on it, with any further progress "in the balance"
As for the specific timeline, Hofmann says the research team started discussions with Ninja Theory as far back as 2018, when Microsoft first purchased the developer, in part because the two are simply both based in Cambridge. The team looked at various options, "very much with an open-ended, exploration point of view - so there was never an expectation that any of the things that we do would actually be put into the games." Ninja Theory then offered the gameplay data the studio had collected under the Bleeding Edge EULA to the research team in 2020, the data was anonymised and imported, and the research carried on from there.
The specifics of what the team actually chose to do with this data, meanwhile, wasn't fully settled on until the autumn of 2022, when Hofmann returned from maternity leave to a world where LLMs such as ChatGPT had begun to grow in use and public awareness. "The world had changed in AI," she says, "in the sense of, suddenly, the broad population knew what language models were and what they could do… and so we within the team took that step back and said, well, how does that impact our work? What are the next frontiers that we can explore?" Ultimately, they decided, "Well, we know what works for language, wouldn't it be amazing to figure out what happens when we train on a large amount of actual human gameplay data?"
Notably, Muse is actually one of two research projects being worked on in parallel by the team. The group, of around 10 to 15 researchers, can usually accommodate around "one to three" projects at a time, Hofmann said, with Muse a "sustained effort over the better part of two years," and more than half the team involved for much of that time. There were in fact "multiple points" during the project where the team almost stopped work on it, with any further progress "in the balance" until the "huge public response" to the paper that was published in Nature back in late February allowed the team to continue.
To come back to that core question of whether it's standard practice to work this way round, Hofmann's answer was clear: first that, yes, it is indeed normal. And second, in her view, that her research team actually placed more emphasis on practical uses than most research of this nature normally would.
"In terms of the research process, it's very common to be purely curiosity driven," she said. "And in many ways, I see our project as an example of not doing it this way - which is ironic, because a lot of people don't see that. We started from technical curiosity, but as soon as we saw signs of life - of this is what it's able to do - we put together that interdisciplinary team. We did the user research to understand what the capabilities would be, how this ultimately enabled specific use cases, and then we were especially focusing on ideation and early prototyping.
If the technology is still in such an early stage, why show it to the public now?
While Hofmann is clear about the order in which the research has unfolded, and the level to which developers have been consulted along the way, the elephant in the room is Microsoft itself. Microsoft is one of the world's largest publicly-traded companies, and one which happens to be in an arms race with other, AI-focused tech competitors, as each attempts to justify - and find a way to ultimately profit - from their investments.
OpenAI notably lost $5bn last year and is projected to lose double that in 2025, and triple in 2026. In late February it was revealed that Microsoft had backed out of new datacentre leases with a power capacity equivalent to the entire operational IT load of London, potentially signalling a lack of demand to meet its previously planned generative-AI supply.
There is, obviously, an incentive for Microsoft to reveal new, exciting things that can be done with generative AI as early as possible. As Cook puts it, while Muse "has some really interesting qualities to it" and there are "things in there that could be useful for developers," those things aren't really the focus of how the research was presented by Microsoft in the first place. "A lot of the positioning does seem to be: positioning yourself as a company that uses AI and that invests in AI and that is on top of AI advances. It does seem to be a shareholders thing."
The Muse reveal on Xbox Wire suggested that people who play video games should care, but it caused a backlash and offered little in the way of concrete benefits to look forward to. | Image credit: Xbox
To Cook, the way Muse has been presented in general is a concern. To him, "there's a difference between what the researchers were trying to achieve, and how Microsoft presented it - and in this case, I thought it was very stark. I really felt like they were kind of hung out to dry in some ways."
I put this to Hofmann - both the question of why this was being revealed to the public so early, given its "not-fully-working" nature, as Hofmann herself put it, and of whether she felt the team had struggled to communicate what their research actually was.
"I think there's as many opinions and drivers of this as we have people in the team, and potentially a lot more," Hofmann laughed, on the topic of going public early. "Part of it comes from the academic research community. Within academic research, there is a drive and incentive and realisation that it's important to be as open as possible," she explains. "A lot of research does not get into the hands of people where they can try something this quickly… we said, 'Okay: there is something here that even has that possibility; a lot of research is not very accessible by definition; so here is something that could be accessible. Let's put it out.'"
At the same time, Hofmann agrees it would be "largely accurate" to say there's been some difficulty in properly communicating what Muse is all about. "Our learnings are very much around: how do we explain what's happening here, what the technical capability is? How do we make that very clear? And with anything we explain, we'll never be able to reach everyone, but I'm certainly looking at the social media activity and I'm taking notes of, okay, here's where the confusion is."
Hofmann maintains, however, that even though there's still "a lot of confusion around what it actually is and what is the purpose, and a lot of debate around that," there has been positive feedback for the team. "Interestingly, everyone who commented on the technical achievement called it impressive, or [were] even more hyperbolic. So on the technical level I'm really satisfied, because people appreciate the achievement, which is fantastic."
"We're very explicit - trying to be very explicit - that this is a technical demo where we intend to show what is becoming possible, and how the field is moving."
That said, Hofmann concedes that the downside of going this early is the impact on the conversation. "It's not something that happens that commonly, even in an age where AI research does move quickly and people do push out an archive white paper or a video demonstration," she says. Typically, when researchers publish something usable, such as an app, members of the public "expect a certain level of product polish, and we're between those. The technology is not ready. We're very explicit - trying to be very explicit - that this is a technical demo where we intend to show what is becoming possible, and how the field is moving."
The goal for that, she says, is to at least "give people that background" to be able to then compare progress down the line. "I'm hoping that ultimately, all those steps will lead to both us understanding how to communicate this more clearly, and then also to the public having built up some of that understanding that helps them interpret what's going on there."
The many ethical concerns, from energy consumption to advanced robotics
For all the big questions and elephants-in-the-room we've worked through so far, there is one more cluster to go that is without doubt the biggest: the many questions of ethics.
As anyone loosely familiar with AI, particularly generative AI, will already know, there are a number of ethical issues that very justifiably arise with each discussion. The first of those is the matter of plagiarism - or intellectual property rights - which in the case of Muse is slightly easier to put aside. Microsoft owns Bleeding Edge developer Ninja Theory and also Quake 2, via Zenimax, and under the EULA therefore has rights to the gameplay footage used as training data here. (That said, there are of course ethical questions about whether it's right for Microsoft, or any entity, to impose EULAs which collect user data at will on anyone wanting to play their games. And indeed about whether it's right for Microsoft to own such a large share of the games industry today - but these are questions for another time.)
More applicable here is the second essential question with generative AI ethics, which is the environmental impact from the sheer amount of energy used to both train the model, and then to use it in practice.
The use of AI impacts people inside and outside of the video game industry, but its affect on the world we live in is potentially catastrophic.
"The energy cost is, I would say, more than you would want for an actual player-facing experience," Hofmann said regarding the Quake 2 demo, when I asked her about this. That public demo uses Nvidia H100 GPUs for the inference, which Hofmann describes as "very similar to an LLM, or a chat bot," in terms of energy cost, and similarly for the training process as well.
"What's making me optimistic about where we can push this is, one, the insights from those last two months around how little data, how little training, and how small a model we could get away with," she adds, which she claims is "nowhere near the end" right now. "We haven't really optimised this for inference cost, for example, or for more efficient training."
"I can't tell you when, but I'm confident this will run on a consumer GPU, or an NPU [neural processing unit] on a mobile phone," she says, giving a rough estimate of "less than two years" until that's possible in terms of inference cost. As for training cost, "the more we learn about how to do this data efficiently, the more we pave the way to something that is truly democratising access to this." I asked Hofmann if she knew the specific numbers involved in terms of current energy cost, either to train or to "play" the model, to which she directed me to Microsoft PR. Microsoft PR told us they did not have anything additional to share beyond what had been published via the company's blog.
As far as broader ethical concerns go, this is far from the last of it. Cook, for instance, suggests one less-cited worry with 3D video game gen-AI more broadly: "It feels like it's sort of a long play for moving toward robotics," he says. "Obviously a lot of these researchers are interested in this stuff for their own sake," he notes, but large tech companies - including those beyond Microsoft, such as Alphabet and Meta - are at least partially looking into AI as it corresponds to video games specifically, he says, because "these projects have two features: they work on video data; and they need to have some model of the world when an action is taken. And these two things are also really important for robots."
He qualifies this, adding, "I know the Muse team, they're interested in games, they're really excited by this stuff. But I think one of the reasons why we're seeing big tech companies invest more in this kind of game-modelling stuff, and why they often talk about it as "worlds" and things like that, is because games have always been a research platform for other purposes."
Less speculatively, there's the more immediate fact that, as Cook puts it, "for lots of the proposals for tools that are powered by AI, their use case seems to be that they would reduce employment. There doesn't seem to be a way around this." Coupled with a growing concern that video game bosses may be implementing AI in their workplaces with a mixture of ignorance and artlessness - one recent report based on a handful of accounts quoted anonymous developers, with one describing it as "an overwhelmingly negative and demoralising force" - there is plenty of reason for those concerns.
All combined, it makes for an almighty mixture of ethical, philosophical, scientific and financial questions - which perhaps is to be expected, given the broader state of AI and the public's natural response to it right now. All those issues are only heightened and complicated by this practice of essentially performing scientific research in front of a live audience, be that out of the pure idealism of accessibility or, I can't help but suspect, the business incentive to do so.
"So with generative AI, the question should just be: do we want this? We don't have to do anything. We don't even have to make games if we don't want to. Sometimes we forget that we do have this power."
For Hofmann, it remains a project that, at least to her, is as personally worthwhile as it is anything else. Her hope is that regardless of the ultimate use case, the research may "add to the wealth and breadth of interactive experiences that are available to us," be that through adding these dream-like (or maybe more accurately for the foreseeable: somewhat nightmarish) elements of an AI-generated game simulation directly, as some kind of additional medium for artistic expression, or in just helping developers think up something else entirely.
"I love video games; I recently got back into Quake 2," she laughs, "I played Cocoon recently… I don't even define myself as a gamer, but there aren't enough games to satisfy the time I would like to spend playing." For her, she concludes, "if people are able to use this to create something that is meaningful to them, and there are people who enjoy it - if I could play a role in that, I'd be happy."
A fellow researcher, I get the sense that Cook remains sympathetic to Hofmann and her team, even if the goals and natures of their research are notably different. I ask him somewhat bluntly, towards the end of our conversation, if he feels people are right in reacting so viscerally to what Microsoft revealed, even with all the communication mishaps, the well-meaning intentions, and the public learning-on-the-fly in mind.
"I think it's justified for a number of reasons," he says. "One of the reasons is just simply that people are so burned out and hurt by all of the things that are happening in the space, that I think there's just a general sense of anger and despair. And I think that's completely understandable." The industry has, of course, been going through a prolonged period of unprecedented layoffs and general uncertainty. The people staying in the industry after all this, as Cook puts it, "they're doing it because they truly love it and they want to do it, and so seeing this creative practice that they love treated in a sort of disrespectful way, it hurts them as well. It's not just about the morals or the ethics or anything like that. This is a thing they care about."
When it comes to this practice of doing science in public, and how these findings are presented, he adds, "I see that there's a responsibility. The public are not stupid, but they can only work with the information they're given, and often they're given really bad information."
"And so we have to think that going with these gut reactions makes sense right now; it's all we've got to work with. And then hopefully over time, in the future, we can slightly start to build trust again and figure out the bits of AI that we want to keep and that we like."
A final, forgotten question for Cook is also the most important. "Not even: does it make games better; but do people want it? And they don't have to want it - technology is rejected all the time, for all sorts of reasons," he says.
"So with generative AI, the question should just be: do we want this? We don't have to do anything. We don't even have to make games if we don't want to. Sometimes we forget that we do have this power; we can just not have something if we don't want to. And so players should think more about: what do they really want from the future of games? Because they can want anything. They don't just have to want the things that they see in tech demos, at E3. They can build whatever future industry they want."
0 Комментарии
0 Поделились
61 Просмотры