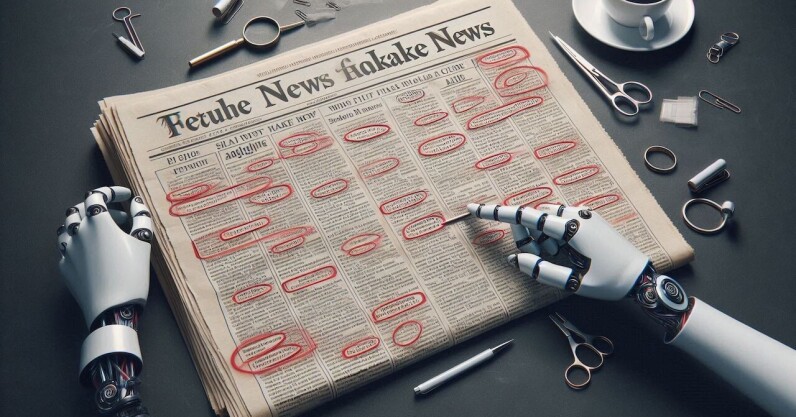
THENEXTWEB.COM
How close are we to an accurate AI fake newsdetector?
In the ambitious pursuit to tackle the harms from false content on social media and news websites, data scientists are getting creative.While still in their training wheels, the large language models (LLMs) used to create chatbots like ChatGPT are being recruited to spot fake news. With better detection, AI fake news checking systems may be able to warn of, and ultimately counteract, serious harms from deepfakes, propaganda, conspiracy theories and misinformation.The next level AI tools will personalise detection of false content as well as protecting us against it. For this ultimate leap into user-centered AI, data science needs to look to behavioural and neuroscience.Recent work suggests we might not always consciously know that we are encountering fake news. Neuroscience is helping to discover what is going on unconsciously. Biomarkers such as heart rate, eye movements and brain activity) appear to subtly change in response to fake and real content. In other words, these biomarkers may be tells that indicate if we have been taken in or not.For instance, when humans look at faces, eye-tracking data shows that we scan for rates of blinking and changes in skin colour caused by blood flow. If such elements seem unnatural, it can help us decide that were looking at a deepfake. This knowledge can give AI an edge we can train it to mimic what humans look for, among other things.The personalisation of an AI fake news checker takes shape by using findings from human eye movement data and electrical brain activity that shows what types of false content has the greatest impact neurally, psychologically and emotionally, and for whom.Knowing our specific interests, personality and emotional reactions, an AI fact-checking system could detect and anticipate which content would trigger the most severe reaction in us. This could help establish when people are taken in and what sort of material fools people the easiest.Counteracting harmsWhat comes next is customising the safeguards. Protecting us from the harms of fake news also requires building systems that could intervene some sort of digital countermeasure to fake news. There are several ways to do this such as warning labels, links to expert-validated credible content and even asking people to try to consider different perspectives when they read something.Our own personalised AI fake news checker could be designed to give each of us one of these countermeasures to cancel out the harms from false content online.Such technology is already being trialled. Researchers in the US have studied how people interact with a personalised AI fake news checker of social media posts. It learned to reduce the number of posts in a news feed to those it deemed true. As a proof of concept, another study using social media posts tailored additional news content to each media post to encourage users to view alternative perspectives.Accurate detection of fake newsBut whether this all sounds impressive or dystopian, before we get carried away it might be worth asking some basic questions.Much, if not all, of the work on fake news, deepfakes, disinformation and misinformation highlights the same problem that any lie detector would face.There are many types of lie detectors, not just the polygraph test. Some exclusively depend on linguistic analysis. Others are systems designed to read peoples faces to detect if they are leaking micro-emotions that give away that they are lying. By the same token, there are AI systems that are designed to detect if a face is genuine or a deep fake.Before the detection begins, we all need to agree on what a lie looks like if we are to spot it. In fact, in deception research shows it can be easier because you can instruct people when to lie and when tell the truth. And so you have some way of knowing the ground truth before you train a human or a machine to tell the difference, because they are provided with examples on which to base their judgements.Knowing how good an expert lie detector is depends on how often they call out a lie when there was one (hit). But also, that they dont frequently mistake someone as telling the truth when they were in fact lying (miss). This means they need to know what the truth is when they see it (correct rejection) and dont accuse someone of lying when they were telling the truth (false alarm). What this refers to is signal detection, and the same logic applies to fake news detection which you can see in the diagram below.For an AI system detecting fake news, to be super accurate, the hits need to be really high (say 90%) and so the misses will be very low (say 10%), and the false alarms need to stay low (say 10%) which means real news isnt called fake. If an AI fact-checking system, or a human one is recommended to us, based on signal detection, we can better understand how good it is.There are likely to be cases, as has been reported in a recent survey, where the news content may not be completely false or completely true, but partially accurate. We know this because the speed of news cycles means that what is considered accurate at one time, may later be found to be inaccurate, or vice versa. So, a fake news checking system has its work cut out.If we knew in advance what was faked and what was real news, how accurate are biomarkers at indicating unconsciously which is which? The answer is not very. Neural activity is most often the same when we come across real and fake news articles.When it comes to eye-tracking studies, it is worth knowing that there are different types of data collected from eye-tracking techniques (for example the length of time our eye fix on an object, the frequency that our eye moves across a visual scene).So depending on what is analysed, some studies show that we direct more attention when viewing false content, while others show the opposite.Are we there yet?AI fake news detection systems on the market are already using insights from behavioural science to help flag and warn us against fake news content. So it wont be a stretch for the same AI systems to start appearing in our news feeds with customised protections for our unique user profile. The problem with all this is we still have a lot of basic ground to cover in knowing what is working, but also checking whether we want this.In the worst case scenario, we only see fake news as a problem online as an excuse to solve it using AI. But false and inaccurate content is everywhere, and gets discussed offline. Not only that, we dont by default believe all fake news, some times we use it in discussions to illustrate bad ideas.In an imagined best case scenario, data science and behavioural science is confident about the scale of the various harms fake news might cause. But, even here, AI applications combined with scientific wizardry might still be very poor substitutes for less sophisticated but more effective solutions.Magda Osman, Professor of Policy Impact, University of LeedsThis article is republished from The Conversation under a Creative Commons license. Read the original article. Story by The Conversation An independent news and commentary website produced by academics and journalists. An independent news and commentary website produced by academics and journalists. Get the TNW newsletterGet the most important tech news in your inbox each week.Also tagged with
0 التعليقات
0 المشاركات
133 مشاهدة