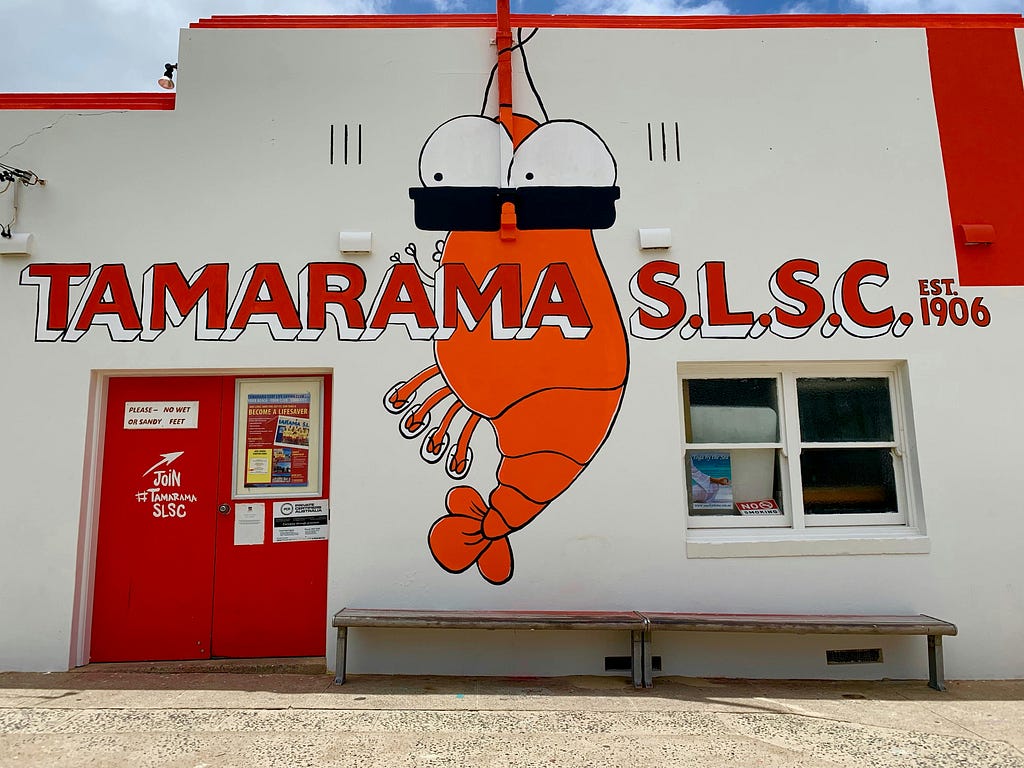
UXDESIGN.CC
How to close the customer-data-gap
Thick or qualitative data (1) helps organizations and their AI-solutions make better decisions by including insights about the context, nuances and influences that leads to customers behaviors and decisions.Photo by G N: https://www.pexels.com/photo/australia-sydney-surf-beachlife-1695310/But thick data sounds expensive, difficult and complicated to collect anduse.It isnt.In fact collecting thick data means doing almost the same as we are already doing. Only asking different questions.First: What is thickdata?According to Gilbert Ryle (2) thick compared to thin datais:thin: includes surface-level observations ofbehaviorthick: adds context to suchbehaviorPut even simpler: thick data is what helps us answer why people are doing what they are doing while thin data only documents what has happened.In an oversimplification (below) we could say thick data is the yellow data on the left helping us understand what can influence people, while channel performance and sales data are thin data on the right documenting what people aredoing.Some organizations use product and sales data (purple) as proxies for human data (yellow). This can lead to a situation where an understanding of the customer gets replaced with a focus on the proxies becoming the goal in themselves.Why should we care about thickdata?AI-engines use both thin and thick data. The former is used to look for patterns and probabilities, while the latter helps the AI better understand context, nuance, culture, ritualsetc.According to a search on Perplexity (3): thick data significantly enhances AIs ability to understand and respond to complex human behaviors and motivations, leading to more nuanced, personalized, and effective performance across various applications.Ethnographer Tricia Wang (4) argues that over-reliance on thin data was part of Nokias downfall. Their own thick data was clearly suggesting that even low-income individuals would invest in smartphones, while Nokias quantitative data was telling it (what it wanted to hear), that the group would keep buying dumbphones.In hindsight its easy to see the fall of Nokia (dumb phones) and the rise of Android (Smartphones) in the Chinese market 20082012. Image by Big Fish Presentations and TriciaWang.How do we get thickdata?Thick data is readily available almost everywhere, especially online. We only need to ask forit.Which is kind of the problem: we dont. For no apparentreason?But the value is there, for anyone who wants it. Right in front ofus.These are just a few examples of questions weve answered by capturing thicker data about the customer:What job [need] are customers trying to solve which leads them tous?How do customers identify themselves?Are customers looking for products or insights? (Where are they in their job-map / journey)?What is the perspective / lens /context through which customers understand youroffer?What else influences customers decisionmaking?What language (terminology) do customers use?How do customers measuresuccess?Are we helping customers achieve thissuccess?This is how you get thick data online (one generalized approach):Collect assumptions by asking your team what has to be true? in relation to what you are trying to achieve (can be anything from strategy to creative concepts or execution).Choose the assumptions that you want to test and articulate them into a clear experimentation statement (if x happens then y) identifying where and how you will run the experiment including how to measure it and what success looks like(5).Create the experiment, run it, analyze andadjust.Now scale this from a novel to a system wideapproachWe talk about thin or quantitative data being at scale, but are we resigned to qualitative data having to be expensive, slow, complicated and not to scale? If so this is only because weve decided to think it likethis.We can ask any question and design any interaction to capture its answers. So why not scale up the thick data production? Breaking from the boring data everyone else has (which we collect and make sense of in the same way and with the same algorithms whats the fun in that?(6))Asking thick questions should be as natural as asking thin questions (e.g. open-rates and conversion funnels). Whynot?We need to establish new design or experience principles that put learning about our customers (not our channels) at the forefront, make available compliant learning modules and objects as part of our design and experience systems. And we need to create demand for them by having our teams ask for better questions, not betteranswers.This would produce a constant flow of thick data from across the ecosystem feeding into the AI models in real-time and atscale.Competitive advantageThin-questions are thinking inside a box inside a box. Why-questions are thicker, they help us understand the customer when they are with us and when they arenot.A box inside a box. Icons by thenounproject.Thin questions are conventional and conformative. They make us the same as everyone else. Everyone collects the same data, asking the same questions using the same algorithms.Thick questions are competitive advantage and the best question is whynot?Sources:(1). Pratibha Kumari J., What is Thick Data?, https://www.linkedin.com/pulse/what-thick-data-pratibha-kumari-jha/(2). Wikipedia, Thick description, https://en.wikipedia.org/wiki/Thick_description(3). Search related to thick on Perplexity, https://www.perplexity.ai/search/in-general-terms-whoch-data-do-4myjkNOXSPWBSNYfyv8iyw#1(4). Tricia Wang, The human insights missing from big data, https://www.youtube.com/watch?v=pk35J2u8KqY(5). Helge Tenn, Business experimentation, https://medium.com/everything-new-is-dangerous/business-experimentation-f5620919f209(6). Helge Tenn, Customer as Competitive Advantage, https://uxdesign.cc/customer-as-competitive-advantage-19a6ede62852How to close the customer-data-gap was originally published in UX Collective on Medium, where people are continuing the conversation by highlighting and responding to this story.
0 Commentarii
0 Distribuiri
3 Views