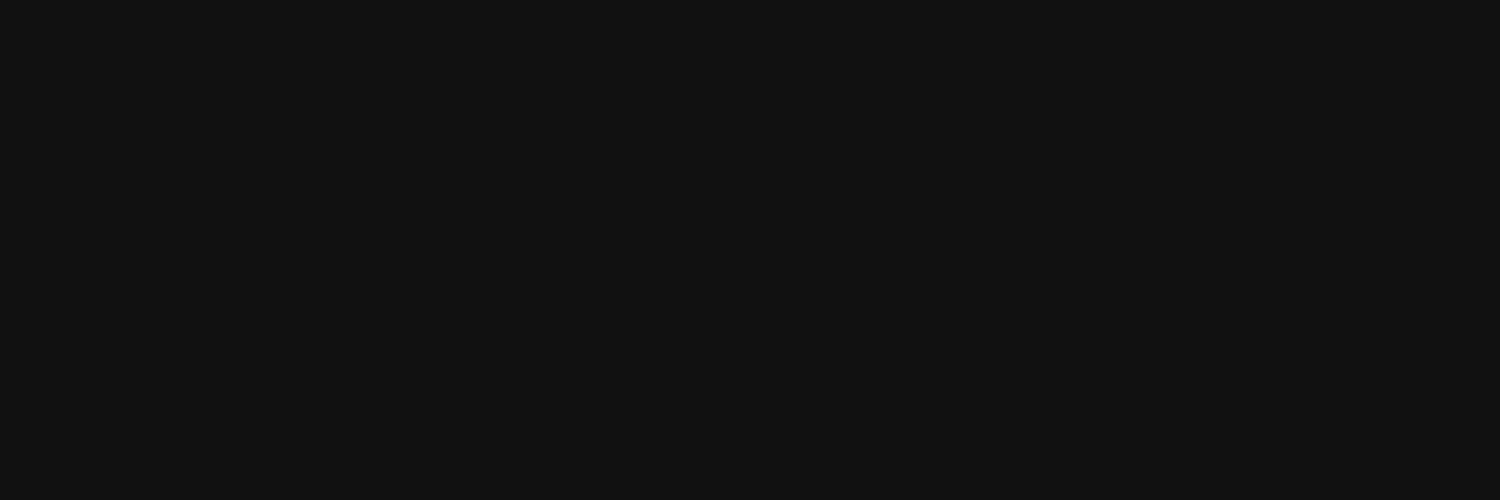
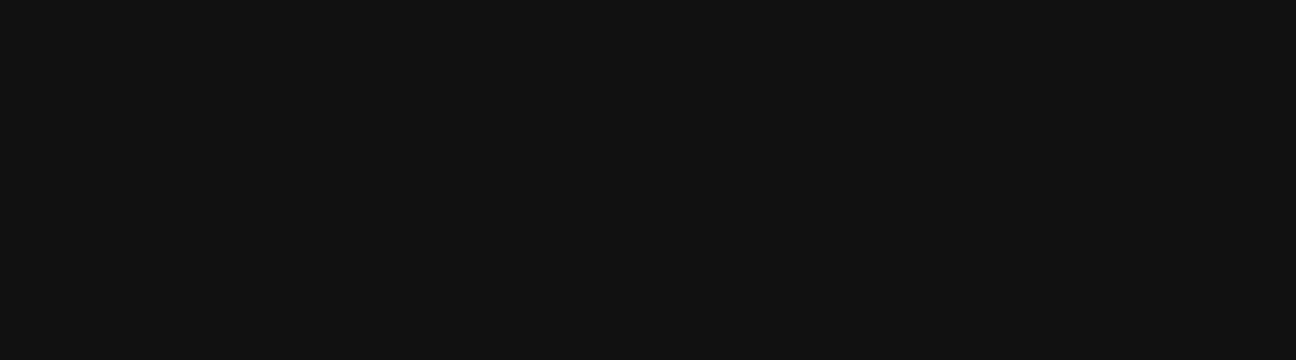
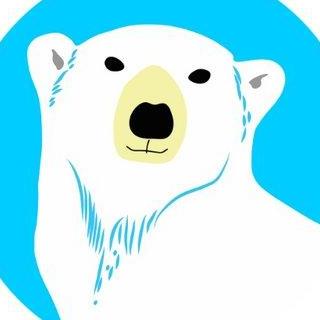
Curated stories on user experience, usability, and product design. By
@fabriciot
and
@caioab
.
@fabriciot
and
@caioab
.
1 people like this
474 Posts
2 Photos
0 Videos
0
Reviews
Share
Share this page
Recent Updates
-
A guide to AI prototyping for product designersuxdesign.ccAI is more than a product, its a platform that will change how and what we designand who gets involved.Continue reading on UX Collective0 Comments ·0 Shares ·13 Views
-
A framework for better UX capacity planninguxdesign.ccMany companies struggle with aligning on level of UX Effort a project needs. This framework canhelp.If theres one pain point Ive seen designers and researchers at every company Ive ever worked at, consulted with, or advised struggle with, its lack of alignment on the capacity & priority of UX Design & Researchwork.Some of the struggles come from designers who expect to do the full UX process (whatever that means) on every project. Other designers have anxiety letting developers implement anything theyve designed without first usability testing theirwork.Even more common is a lack of alignment with Product Management on the level of research & design effort a particular project should justify. Often, this is because no actual capacity planning for UX work takes place. Effort, Estimates, Roadmaps, and Capacity are all terms most commonly assigned to engineering work. Commitments are made, and planning is done for the delivery phaseonly.To be fair, Ive also observed that its not so much that teams dont want to include UX in their estimates. They often just dont know how. Theres no standard framework for level setting across the board around Design & Research Effort. Untilnow.Problems tosolveThere were 4 main problems I set out tosolve:Improve UX Capacity planning and improve alignment between UX, Product, & Engineering on level of UX effort needed in varied circumstances.Set realistic expectations on the kind of UX activities that can realistically be expected in the timeframeHelp UX team determine level of discovery, iteration, and executionEmpower UX team to spend more time on projects where they can add the mostvalue.Pendo Research Effort FrameworkOthers have taken a stab at similar problems. In August of 2021, Jeanette Fuccella published a research prioritization framework on the Pendo blog attempting to solve the problem of determining how much research todo.She created a quadrant across two dimensions: Problem Clarity &Risk.Credit: Jeanette Fuccella 2021 https://www.pendo.io/pendo-blog/blog-authors/jeanette-fuccella/Problem Clarity: High and Risk: Low was Ship it and Measure.Problem Clarity: High and Risk: High was DesignHeavy.Problem Clarity: Low and Risk: Low was Research Light.Problem Clarity: Low and Risk: High was Research: Heavy.While very useful at a conceptual level, there were some practical obstacles to overcome:It provided little context on what Research Light vs Research Heavy meant. It illustrated when to do which level of research, but not what that research entailed.Most companies dont have the luxury of having a big enough research team to do all the project-level research. So the question came up of when should a designer do the research and when should it be left to a full time researcher?Attempting to combine both research and design into 1 matrix was overly simplistic. It helped some with determining research effort, but I saw a big opportunity to do a similar thing for Designeffort.UX Effort Framework 2.0My new framework splits out research effort from design effort, included t-shirt level effort guides, a description on what each level of effort is (and when to use it), as well as common activities or attributes perlevel.For Research Effort, I stayed with Problem Clarity vs Risk. Risk also makes sense for Design work, but I replaced Problem Clarity with Complexity (as complexity is often what determines how much iteration we need for designwork).PROBLEM CLARITY:HIGHThis is a problem weve worked on before, have extensive existing qualitative & quantitative research we can leverage, and/or shared understanding of across theteam.PROBLEM CLARITY:LOWThis is a largely new or unknown problem. Lots of assumptions exist. The problem is not well defined and significant alignment needs to happen. This work is likely at the strategic / initiative level.RISK: HIGHWork that is not easily undoable, affects a large % of users key workflows, and/or has a large amount of risk to the business or user experience.RISK: LOWWork that is easily undoable, affects a small % of users, does not affect mission-critical workflows, and where getting it wrong would have only a small impact to the business or user experience.COMPLEXITY: HIGHInvolves many systems/APIs, external dependencies, stakeholders across multiple teams, or is technically complex (in ways that affect design decisions).This is a problem space with many questions or in which the designer has little existing knowledge.COMPLEXITY: LOWIs mostly self-contained in terms of systems and stakeholders, has few if any dependencies, and is straight-forward.Often these are simple usability improvements or additional use cases in well-understood workflows.Research Effort QuadrantsTo help understand each effort level better, lets take a closer look at each of the Research Effortlevels.SHIP &MEASURESometimes we need to prioritize speed over research rigor. This is best done when the problem clarity is high and risk is low. Often this step entails partnering on a post-release strategy and can be done by a designer.RESEARCH LIGHTEven when risk is low, if we have low clarity around the problem were trying to solve it is wise to do some research. Yet, we dont need to spend weeks starting from scratch. Research Light also usually focuses on follow up discovery research. Since the risk is low, usability testing is not usually needed at thislevel.Research Light is most often appropriate when we have prior quantitative or qualitative research we can leverage but still have unanswered questions. This research is typically done by the design team. Freeing dedicated research teams up from doing Research Light projects is a key element of improving the value created by researchteams.RESEARCH MEDIUMWhen risk is high, even if problem clarity is high it is important to invest in research. The key difference between Research Medium and Research Light is that since the risk is high we do need to invest in evaluative testing since its not easily undoable or the cost to the business if we get it wrong ishigh.It is also worth noting that this is the most common level of research that a designer will conduct if a dedicated research team is established.RESEARCH HEAVYFor larger projects spanning multiple projects, teams, or journeys, more rigorous research is needed. Often these projects will require multiple research methods spanning generative & evaluative, qualitative & quantitative, and attitudinal & behavioral.This kind of research typically is more strategic in nature and should be completed by a trained and experienced researcher. It often requires more time than a designer often has to run on theirown.Design EffortLevelsThe level of design effort needed between quadrants detemines:Level of fidelityneededLeveraging existing patterns vs creating newpatternsAmount of iteration requiredWhat stages of UX work are needed (modeling/mapping, wireframes, mockups, prototypes, etc)The intention here isnt to restrict the designer to a particular deliverable, but instead to communicate to partners the type and amount of work needed depending on risk vs complexity.CONSULT ONLYNot every project needs direct UX hands-on work. When the risk and complexity are low, it is 100% acceptable for UX to be consulted rather than assigned work (mockups) to do. This will enable the design team to spend their time on work where they can have the mostimpact.Doing more consultations when risk is low, complexity is low, and we are leveraging existing design patterns is a key to unlocking UXs potential to contribute more strategically to building the business. Too many designers spend far too much time being mockup monkeys for pre-determined solutions.In those cases, it is extremely helpful to set the expectation with your product team that youd love to just have a quick connect to align on the right component to use, flow, etc but that then the engineeering team should be empowered to do that work. This is one of the biggest benefits of having a design system inplace.Ive even worked places where a front end engineer would spin something up quickly with a mocked up back end and then just show it to me. Wed discuss a minor tweak or two and then it would be ready to connect to the real back end and be released.Letting go of needing to do a mockup on every little UI change is a key tactic in being able to spend more of your time on the more complex and risky (and impactful) work for which you are uniquelysuited.DESIGN LIGHTEven if the complexity is high, if the change youre contemplating would be easy to undue if it went awry, theres often no need for a high level of iteration, low fidelity pre-work, etc. Just get it out there and test in thewild.This is work where due to the complexity, were not comfortable just consulting, but we also dont need a month of design work. Another key component here is where the cost to the business or user experience would be low if yourewrong.HINT: work related to personal info, financial transactions, government regulations, or mission critical systems should never fall within DesignLight.One of the biggest mistakes I see teams make is wanting designers to do UX Light work on every project. One of the big value-adds of using a framework like this is to help illustrate that we absolutely can do UX Light work, but only when Risk islow.DESIGN MEDIUMWhen Risk is high, its important to do more iteration and start problem solving at a lower fidelity. If the complexity is low not as much of this is needed, but it still makes sense to spend some time iterating to avoid costly rework and negative businessimpact.Another common reason for Design Medium rather than Design Light has to do with situations where a new pattern / component needs to be introduced. Extra validation and testing are needed and its important to allow capacity forthat.DESIGN HEAVYFinally, we arrive at Design Heavy. These are complex, risky projects where getting it right is essential. Often Design Heavy is paired with Research Medium or Heavy as well unless extensive prior research has beendone.This level is what many designers think of when they talk about the Full UX Process. Modeling, Information Architecture work, Low Fidelity, Mockups, etc. Although deep functional prototyping is not always needed, it is important here.The work needs to be more thorough because the cost of getting it wrong would be significant to the customer and/or business.How to use this frameworkThis sounds great, Jeremy, you say, but how do I actually use this? Thats a great question. Let me share anexample.1. PROJECTPLANNINGWhen a project gets prioritized (and often before), we populate the Project description with the following:Background Info (any context needed to understand theproblem)Problem to Solve (and why this is worth prioritizing now)Business Impact (orvalue)User Outcome (how will someones lifeimprove)Success Criteria (what do we need to observe to know we were successful)Usually these are populated by the Product Manager, but it definitely can and often is a jointeffort.Then, the assigned UX Designer on the team will take a stab at populating:6. Design Effort level (based on the framework criteria)7. Research Effort level (based on the framework criteria)2. PROJECTKICKOFFWe then hold a kickoff with the balanced team and any key stakeholders (such as UX, Product, and Engineering leaders). We align on on the Project Description (including UX effort) before designers start work. Tweaks or additions are oftenmade.3. DESIGNER ADDS UX STORIES TOJIRAOne thing that Ive found invaluable is to ensure UX tracks their work in a project management tool such as Jira. This helps provide clarity & transparency to partners, and allows us to hold ourselves accountable.Once were all aligned on all the above, the designer will break up the project into individual work items (stories, tasks, product backlog items whatever flavor the org prefers). Guideline for level of fidelity is that each backlog item should be tied to some sort of deliverable (research plan, wireframes, mockups v1, research readout, etc). The Effort Framework operates as a guide on what backlog items should bedone.Each backlog item gets a specific estimate and is prioritized according to PMs direction on which project should be worked on first, etc. Backlog items then get dragged into Sprints based on the designers capacity.4. PLANNINGAt this point, UX stories are reviewed with Product & Engineering and adjustments are made according to priority, business needs, etc. Our goal is to do this for all projects on the roadmap for a particular quarter. We recognize changes will happen but having something written up and prioritized for all projects in a given quarter enables UX Capacity to be taken into account when doing Quarterly Planning which results in more realistic expectations being communicated to executive leaders.NOTE: Im a firm believer in prioritizing problems & outcomes rather than features. Thats an ideal state I always work towards. I have found, however, that isnt the way most teams realistically work. So ensuring a problem and outcome is defined for projects being prioritized and letting UX Effort and capacity guide release schedules (instead of the other way around) has been a useful & realistic compromise.Im Jeremy. I help orgs build better design teams and designers build better careers. Need help? Justask.Works Cited / FurtherReading:A Tried and True Framework for Prioritizing User Research - Pendo BlogWhen to Use Which User-Experience Research MethodsA framework for better UX capacity planning was originally published in UX Collective on Medium, where people are continuing the conversation by highlighting and responding to this story.0 Comments ·0 Shares ·13 Views
-
The effort paradox in AI designuxdesign.ccWhy making things too easy canbackfireIn our rush to automate daily tasks with AI, we risk reliving product design mistakes from longago.We can learn a lot from Betty Crocker andIKEA.Product design historylessonsBetty Crockers stumble andrecoveryWhen General Mills introduced the worlds first instant cake mixes in the 1940s, they seemed perfect: Add water to a Betty Crocker mix, bake, and enjoy a delicious cake.But sales disappointed and market research revealed a surprising truth: target audiences overwhelmingly found the process too easy. Betty Crockers customers didnt invest enough effort to feel proud. They didnt feel like they were properly caring for their families, and the product made them feel undervalued.The solution? The recipe was modified to require people to add anegg.This tiny, targeted addition of more work made all the difference. Ads highlighted the egg step, sales soared, and Betty Crocker cake mix wasfamous.IKEA, Legos andorigamiA set of 2011 Harvard Business School studies tested consumers value perceptions of products they assembled, vs. those that experts assembled. The effect was the same for utilitarian products (IKEA boxes) and fun products (Legos and origami animals): participants who constructed products valued them higher than preconstructed versions of the same products.Modern designers and businesses often focus on minimizing effort and saving customers time. And that oftenworks.But in many contexts and moments, subtracting effort backfires. Cognitive scientists call this the effortparadox.The AIparallelTodays AI product designers face a similar challenge. In our enthusiasm to automate everything, we risk leaving users feeling disconnected and unrewarded. Just as 1940s homemakers wanted to feel invested in their baking, todays users often want to feeland bemeaningfully involved in AI-assisted work.Thats good because strong collaboration between humans and AI assistants often yields better outcomes than tasks performed by AI models that go it alone. (Soon well dive into how you can leverage that, in an upcoming Mindful AI Design article.)But outcomes aside, removing too much customer effort from AI product interactions can rob customers of psychological ownership and satisfaction.Real-world AIexamplesAI writing assistantsIn a 2024 University of Waterloo study, participants wrote short or long text prompts, which were fed into an AI service that generated stories.People who created more detailed and extensive prompts reported notably stronger feelings of psychological ownership over the final stories, compared to those who provided shorter, simpler prompts. (To a point. The beneficial effects plateaued as the input neared the output story lengtharound 150words.)Notably, the perceived quality of the resulting stories didnt vary based on addedeffort.Reimagining the car ownersmanualThe principle played into my work on Smart Manual, an AI-powered conversational car manual and repair assistant. Early concept testing revealed a consistent theme: While respondents appreciated faster answers to car problems, the were uncomfortable relying solely on the AIs advice and instructions. Two users explicitly requested the ability to cross-reference the original car owners manual to verify the AIs troubleshooting reasoning.Early sketches of Smart Manual concept. In interviews, drivers expressed reservations and distrust about this version of the AI concept. They wanted more direct views into actual ownersmanuals.We updated the Smart Manual interface to surface relevant diagrams and excerpts from the car manual where appropriate, and to always link clearly and directly from AI summaries to the original manual material.That encourages drivers to verify the agents information. And it helps them dig deeper, and learnmore.Feedback drove us to integrate content from the original car owners manuals directly and frequently throughout Smart Manual interactions. This makes it easier for drivers to double-check the AIs accuracy, and it helps them feel involved in maintaining, repairing, and learning about theircars.This approach requires a bit more user efforta bit more taps, more scrolling, more thinking, and more deciding. But it builds trust and human involvement, and we hope it will boost the tools credibility. We hope to amplify drivers capabilities by framing the AI as an aid to making informed decisions, rather than as a magical cure-all.Finding the SweetSpotAs these examples show, setting the stage for meaningful human engagement in AI design requires the right balance between over-automation (excluding people from work where they bring, and derive, value) and human drudgery (bogging people down with work they dont enjoy and that AI is well suited to deliver).Its about identifying those places where a bit of extra effort can boost users sense of accomplishment, control, and investmentand foster a sense of ownership andmastery.That sweet spot will vary for different users and contexts. Some will call for more hands-on involvement, while others will need more automation.Guidelines for AI Product Designers1. Find the optimal human touchpoints. Distinguish between genuine friction and moments of meaningful effortwhere human involvement boosts value and enjoyment. Consider:What parts of this process give people a sense of accomplishment?Where does human judgment add genuinevalue?When is the effort the pointwhen people want to grapple with a problem ortopic?How can we augment, rather than replace, human capabilities?2. Preserve human agency. Give people clear control over key decisions.3. Show the work. Make AI processes transparent enough to keep people informed and involved, and design clear means to verify AI outputs. (This requires a nuanced balance. Too much explanation can bog down usersand AI toolstoo.)4. Help people learn, and help them refine explorations based on their learnings. That often beats flat, one-shotanswers.5. Consider customization. For more advanced users and contexts, controls for people to adjust levels of automation can be appropriate.Remember: effort isnt ourenemy.WALL-Es world of over-automation. It was a beautiful movie. Lets not make it areality.Looking AheadTheres a lot more to dig into here with research around human effort, perceptions of effort, and how this can impact the design of AI products.Studies already suggest that: Human perceptions of effort arent fixed and can be shaped dramatically by learning and experience. Different people judge levels of effort differently. Rewarding mental labor now can boost peoples willingness to expend effort in thefuture.As AI capabilities expand, the temptation to over-automate will grow stronger. Thats not an AI thing, its a human thing. We repeat that story with every new wave of technology. (Remember The Tragic Life ofClippy?)Its our job as mindful designers to steer past that tendency.Its time to set aside the false binary of manual vs. automated to ask more nuanced questions.This will be key to designing AI products that respect human agency while amplifying human potential.What do youthink?Have you felt unsatisfied with an AI tool that made something tooeasy?Have you encountered examples of the effort paradox in customers reactions to an AI product or service youre workingon?Have you had any success finding the sweet spot between overautomation and too much hassle, or finding those optimal human touchpoints and moments when injecting a bit of human effort can boost enjoyment or engagement?Please tell us about it in the comments.Satisfaction lies in the effort, not in the attainment MahatmaGandhiPart of the Mindful AI Design series. Alsosee:Do mosquitoes bite leeches? Keys to calibrating trust in AI productdesignBlack Mirror: Override. Dystopian storytelling for humane AIdesignRelatedThe Effort Paradox: Effort Is Both Costly and ValuedPMCWriting with AI Lowers Psychological Ownership, but Longer Prompts Can Help | AI Research PaperDetailsThe IKEA Effect: When Labor Leads to LoveMichael I. Norton Daniel Mochon DanArielyRewarding cognitive effort increases the intrinsic value of mental labor |PNASOn the specifics of valuing effort: a developmental and a formalized perspective on preferences for cognitive and physicaleffort15 Times to use AI, and 5 Not toby EthanMollickThe effort paradox in AI design was originally published in UX Collective on Medium, where people are continuing the conversation by highlighting and responding to this story.0 Comments ·0 Shares ·34 Views
-
AI is reshaping UIhave you noticed the biggest change yet?uxdesign.ccAI is reshaping UIhave you noticed the biggest changeyet?AI is reshaping interactions as we know them, driving a new UI paradigm. Lets break downhow.Goodbye commands, hellointentThe way we interact with software is anything but static. Sometimes its a gentle evolution, other times a jarring leap. Today, a growing wave of design pioneers, including Vitaly Friedman, Emily Campbell and Greg Nudelman are dissecting emerging patterns within AI applications, mapping out the landscape that refuses to stand still. At first glance, this might seem like yet another hype cycle, the kind of breathless enthusiasm that surrounds every new tech trend. But take a step back, and a deeper transformation becomes apparent: our interactions with digital systems are not just changing; they are shifting in their veryessence.Imagine the transition from film cameras to digital photographysuddenly, users no longer had to understand exposure times or carefully ration film. They simply clicked a button, and the device handled therest.AI is bringing a similar shift to UI design, moving us away from rigid, step-by-step processes and toward fluid, intuitive workflows. The very nature of the interaction is shifting, and as Jakob Nielsen recently stressed in his article, this evolution demands our full attention. He articulates a crucialinsight:With the new AI systems, the user no longer tells the computer what to do. Rather, the user tells the computer what outcome theywant.This isnt just a technological evolutionits a philosophical one. It challenges long-held assumptions about control, agency, and human-machine collaboration. Where once we meticulously dictated every step, we now define intentions and let AI determine the best path forward. This transformation is as profound as the move from command-line interfaces to graphical user interfaces, and for UI designers, it represents both an opportunity and a challenge.Tapping, swiping, asking: How interaction isevolvingBut before we dive into how AI is reshaping interaction, its important to reflect on what has defined our most intuitive interfaces so far. In 1985, Edwin Hutchins, James Hollan, and Don Norman published a seminal paper on direct manipulation interfaces. Norman later defined some of the most widely accepted design principles in The Design of Everyday Things, while Hutchins pioneered the concept of Distributed Cognition. But in 1985, they, along with Hollan, captured a pivotal moment in design history when direct manipulation was emerging as a dominant strategy.Direct manipulation is an interaction style in which users act on displayed objects of interest using physical, incremental, and reversible actions whose effects are immediately visible on the screen.NN/gBut what does this mean in simple terms? Say, you need to move a file from one folder to anotherthis is a classic example of direct manipulationyou see the file, grab it, and move it exactly where you want it togo.You start by recognizing your goal (1). Then, you locate the file in its current folder and decide to drag it to the new location (2). You click and hold the file, move it across the screen, and drop it into the target folder(3).If you accidentally drop it in the wrong place, you immediately see the result, adjust your approach, and drag it again until it lands where you intended. This kind of interaction feels intuitive because it minimizes cognitive effortthe system responds in real-time to your actions, reinforcing a sense of direct engagement andcontrol.The smoother this process, the more natural and satisfying the interaction feels.Moving a file on MacOS using direct manipulation involves dragging that file from the source folder and moving it into the destination folder.SourceWhile reducing distance improves usability, what truly defines direct manipulation is engagement. The authorswrite:The systems that best exemplify direct manipulation all give the qualitative feeling that one is directly engaged with control of the objectsnot with the programs, not with the computer, but with the semantic objects of our goals and intentions.Direct manipulation has remained a foundational design principle for decades. However, as we transition into AI-driven systems, we must consider how these principles evolveand when they give way to goal-oriented interactions.Now, think of Windows Photos AI-powered Erase feature. Say you take a picture of your dog, but theres an unwanted leash in the shot. Instead of manually selecting pixels and meticulously editing them out, as you would have done a decade ago, you simply select the leash and let the AI handle the rest. The system understands your goalremove the leashand executes the best possible solution.Windows Photos,sourceThis interaction still involves some level of manipulation, as you must indicate the object to be erased, but the difference is that you are refining a request rather than directly altering pixels. You are no longer meticulously editing every detail; you are collaborating with the system to achieve a desired outcome. This shift marks a fundamental evolution in UIdesign.Desolda, along with fellow researchers, captured this dynamic in a model based on Normans Gulf of Execution and Gulf of Evaluation. Unlike straightforward direct manipulationsuch as dragging a file between folders, where actions unfold step by stepAI interactions demand a more fluid, iterative process. Users articulate their goals, but instead of executing every step manually, they collaborate with the system, refining inputs and guiding the AI as it interprets, adjusts, and responds dynamically.SourceThe continued relevance of direct manipulationAI may be reshaping the way we interact with technology, but direct manipulation isnt going anywhere. Even in an era of intent-based interfaces, users will still need to engage with AI systems, guiding them with the right inputs to translate human goals into machine-readable instructions. Designing AI experiences isnt about replacing direct manipulationits about enhancing it, layering new interaction models on top of well-established patterns to make interactions smoother, more intuitive, and ultimately, more powerful.To design seamless AI experiences, we need to recognize and build on familiar patterns.For instance, in many AI applications, an open-ended prompt field acts as an icebreaker, helping users get the conversation started. Built upon the familiar input field pattern, which has been a standard UI component for decades, this method now serves a new role. Whether its typing a question into ChatGPT or instructing a design tool to generate a layout, this approach provides flexibility while guiding user intent in an intuitive and approachable way.Open input pattern examples, SourceThis approach isnt limited to interaction patternsit extends into UX frameworks aswell.For example, Evan Sunwall introduced Promptframes as a way to complement traditional wireframes by integrating prompt writing and generative AI into the design process. The goal is to increase content fidelity and accelerate user testing by incorporating AI-powered content generation earlier in the workflow. Yet, this concept is built upon the foundation of wireframes, reinforcing the importance of understanding traditional UX structures to effectively design for AI-driven experiences.Final thoughtsThe best interface experiences are the ones users dont notice. They dont demand your attention or make you think about how to use themthey just work, letting users focus on what they came to do. AI, when done right, follows this same principle. It doesnt need neon powered by AI labels; it should weave itself so seamlessly into the user journey that it feels like a natural extension ofintent.Take Netflixs recommender system. It doesnt interrupt your experience to remind you its using advanced algorithms. It doesnt ask you to configure a dozen settings. Instead, it quietly learns, adapts, and presents suggestions that feel effortlessso much so that you rarely stop to think about the system behind it. Thats what AI-driven interaction should be: not a feature you have to wrestle with, but an invisible assistant that refines itself around yourneeds.As we move toward intent-driven systems, this is the bar designers should aim for. AI should reduce friction, not add complexity. It should empower users, not overwhelm them with unnecessary choices. The best AI isnt the one that demands attentionits the one that disappears into the flow of what you were trying to accomplish in the firstplace.AI is reshaping UIhave you noticed the biggest change yet? was originally published in UX Collective on Medium, where people are continuing the conversation by highlighting and responding to this story.0 Comments ·0 Shares ·27 Views
-
Watch out: Gemini is coming for your Google Workspaceuxdesign.ccThe race for AI-powered work tools heats upContinue reading on UX Collective0 Comments ·0 Shares ·37 Views
-
The end of design certaintyuxdesign.ccWhy AI forces us to embrace emergence instead of clinging to control and understandingMade by Patrick Morgan with MidjourneyAnthropic CEO Dario Amodeis recent podcast with Lex Fridman caught my attention with an observation that keeps replaying in my mind. Even as his team works to understand and interpret AI models, he acknowledged a surprising truth:Theres no reason why [AI models] should be designed for us to understand them, right? Theyre designed to operate, theyre designed to work. Just like the human brain or human biochemistry. Theyre not designed for a human to open up the hatch, look inside and understand them.This statement gave me pause. It challenges something deeply ingrained from the last generation of software design: the idea that we must fully understand how something works in order to consider it a valid solution. For years, weve doubled down on the belief that data-driven design can eliminate uncertainty. The idea is that to make something effective, we first have to deconstruct it, grasp every nuance, and then carefully engineer it into existence.But theres an irony here: as weve become more obsessed with data-driven certainty, weve invented systems that operate beyond our ability to fully analyze or predict. AI models demonstrate that sometimes the most powerful solutions emerge from patterns we can observe but not fullyexplain.AI flips the script. It wasnt designed for human comprehensionit was designed to work. Understanding, if it comes at all, is often after the fact, something we piece together after we see that the system works. And that realization has forced me to rethink my assumptions about design and invention for this newera.Rethinking solutions in search of problemsTraditional design wisdom views solutions in search of problems as a criticism. It runs counter to everything were taught about being problem-focused and user-centered. But as Anthropics head of product design Joel Lewenstein observed in a recent Dive Club podcast with Michael Riddering: Ive come to see solutions in search of a problem as not a dirty word at all as long as you just lean into it and state your assumptions, saying look, theres the germ of something here and were going to exploreit.This isnt about abandoning user-centered designits about recognizing that with AI, understanding often emerges through exploration. The technologys capabilities are so novel that even those working on the frontier dont know whats possible until they seeit.This shift isnt limited to Anthropic, its happening across leading AI companies. Inspired by a recent conversation with Perplexitys head of design Henry Modisett, Linear CEO Karri Saarinen commented, At Perplexity they start projects by exploring LLM capabilities with very simple prototypes, even with just a command-line implementation. Only once theres proof that the idea can work consistently, and that they can bend it to do what they want, do they start designing the experience. Normally, you start with design to explore possibilities, and the tech follows. But in this domain, or this new era of software, LLM/AI is the tool for exploration, and design comesafter.Invention comes before understandingHistorically, software design has followed a structured, step-by-step approachone where every phase is carefully planned to produce a predictable outcome.Understand the problemdeeply.Define a precise solution.Craft an experience that is intentional and predictable.Ship a finished product that behaves exactly as expected.But this isnt how many of the most transformative inventions have come about. If you look at breakthroughs across history, the process is always messy and often reversed:Have an intentan idea of what youre trying toachieve.Experiment, iterate, and push forward without muchclarity.Uncover an unexpected breakthroughit works, but not how youthought.You study the breakthrough, refine it, and later figure out why itworks.This pattern of discovery before understanding runs deep. Alexander Fleming didnt intend to discover penicillinhe noticed something unexpected in his experiment and followed the thread. The steam engine was a product of tinkering; Newcomen and Watt refined working models decades before scientists understood the laws of thermodynamics that made them possible. Early radio pioneers transmitted signals across great distances without fully understanding the physics of electromagnetic waves. And the use of anesthesia in surgery revolutionized medicine long before scientists figured out its precise mechanism ofaction.AI amplifies this historical patternAI doesnt just follow this patternit speeds itup.Traditional software is deterministic; AI is probabilistic. It doesnt follow rigid rulesit generates outputs based on patterns and likelihoods we can observe but not fully predict. The technology itself resists complete upfront understanding.The challenge is that many designers, engineers, and product teams are still trying to apply legacy design methodologies to a technology that simply doesnt work that way. AI doesnt respect our craving for certainty. It doesnt wait for us to fully understand it before showing results. And the more we try to force it into rigid, explainable, deterministic workflows, the more we suffocate its potential.Why design struggles to let gobut why itshouldThis has forced me to confront my own biases. Ive spent a decade designing software, and the instinct to make things fully understood before they exist is deeply ingrained. Its particularly rooted in the culture of UX designthis idea that we cant build effectively unless we first have a full grasp of what weremaking.AI challenges this instinct and asks us to revise our beliefs. It requires us to lean into the ambiguity, to design before we fully understand, and to shape the raw materials of generative outputs as useful options emerge. As Lewenstein notes, You can talk about AI and you can write about AI, but theres something just so powerful about seeing a working prototype and feeling the dynamic, stochastic nature of it seeing a website get rendered in real time, iterating on it and seeing it change in front of youits just magical. Understanding comes through doing, through making something tangible that we can respond to andrefine.Confronting this tension reminds me of the Daoist concept of Wu Wei, often translated as effortless action or without force. Its the idea that instead of rigidly trying to control every element of a process, we should move with the natural flow of thingsguiding and shaping, rather than imposing. Wu Wei isnt passivity; its about working with forces rather than against them. In AI design, this means crafting interactions where users guide and shape outcomes, rather than micromanaging every detail. Its like how a surfer harnesses a waves energy rather than trying to control theocean.Design must guide, notcontrolSo what does it look like to design without force? Instead of suppressing the unknown, we embrace it as part of theprocess.We create affordances, not strict controls: building interfaces that guide behavior rather than dictate it. Instead of trying to expose every parameter, we need interfaces that let users navigate AI while embracing its variability.We prioritize steerability over explainability: giving users meaningful, intuitive ways to shape AIs behavior without needing to understand its internals. The goal isnt to make the black box transparent, but to make it controllable at the right level of abstraction.We embrace emergence: designing systems that adapt and evolve, rather than ones that are locked into rigid, pre-defined behaviors. This means creating spaces where unexpected capabilities can surface and be refined throughuse.This doesnt mean that understanding is unimportant. But it does mean we should be wary of overprioritizing upfront understanding at the cost of progress. AI is teaching us that function canand often mustprecede full comprehension. And as designers, builders, and creative thinkers, we need to get more comfortable working in thatspace.If this makes you uncomfortablegood. It means youre seeing the shift. But if it excites youwell, my friend, youre right where you need tobe.What might become possible if you embrace emergence instead of clinging tocontrol?Embracing theunknownThis shift is much bigger than a throwaway line on a podcast. Its a reframe for how we approach design and invention in this new age. For decades, the software business has trained us to believe that predictability, explainability, and control are the highest ideals. But many of the most powerful things in the worldour brains, ecosystems, markets, and now AI modelsdont operate thatway.If youre designing with AI, start experimenting before you demand clarity. Try tinkering with the raw materials first, then layering on design afterwardlike Perplexity does. Embrace the unknown as a creativetool.As we build in this new era, we need to ask ourselves: what happens when we stop forcing things to fit our desire for immediate understanding? What becomes possible when we embrace discovery as a design principle? And how do we shape these new, emergent systems in ways that are powerful, safe, and genuinely creative?We may not fully understand AI yet. But if history tells us anything, that might be exactly where we need tobe.Patrick Morgan is the founder of Unknown Arts. If you enjoyed this post, subscribe to his newsletter or follow him on social media: X, LinkedIn, Bluesky.The end of design certainty was originally published in UX Collective on Medium, where people are continuing the conversation by highlighting and responding to this story.0 Comments ·0 Shares ·20 Views
-
How I review design workuxdesign.ccDefining what good design actually lookslike.This article is a lightly edited note I wrote for my team of product designers atDialpad.Good design can be tricky. Whats obvious to one person might not make sense to someone else. Design might feel subjective, but there are things I (and the rest of design leadership) consistently look for when reviewing designs.Good design is the result of early and good collaboration. Early designs usually wont check every box, but we can anticipate some stuff and avoid spending too much time on designs that dont quiteland.This document attempts to define what good actually means for design at Dialpad. It gives us a shared target so that when we get to leadership reviews, weve anticipated much of their feedback and they trust that the direction isgood.Have a NarrativeIts important to start with why were doing a project in the first place. Is it a metric were trying to improve? Is it an unmet user need were solving for? A sale were trying to close? Before I look at any screens, I need to understand why were doing something in the first place. It helps us all stick to first principles when reviewing designs.You know how we say that storytelling is important? This is what wemean.Only after I have this context can I give you feedback thats actually helpful and not just skin-deep increase the spacing here type feedback.You dont always need a slide deck, 12 paragraphs or a 30 second spiel is usually good enough. I dont appreciate being dropped in the middle of a Figma file with no notes, nor do I appreciate being linked to a large Jira epic or 15-page PRD just to know what a projectis.Design leadership covers a lot of ground and Im relying on you to tell me what a project is and why itmatters.Be strategic about what you share and what feedback youre after. You shouldnt show and explain everything in the design file. To this end, its helpful to organize your thoughts and Figma file ahead of time. Its incredibly hard to follow someone rambling while zipping around a Figma artboard over screenshare. You likely wont get the feedback youre looking for thisway.Your OwnIdeasOften a PM or engineer will introduce a project with a solution already in mind. Itll be included in the Jira ticket, described in a PRD, or mentioned in a meeting. And thats great. We should definitely explore and draw out other peoples ideas. But I want to see you thinking of your own ideastoo.Figuring out what to do is a big part of senior designroles.I want to see that youre not just executing someone elses ideas. I want to see that youve explored an idea from at least two or three different angles (read: not the same idea with two or three minor variations).Coming up with different ways to solve a problem shows that youre not just doing what youre told, but really using your own judgment. Thats what makes someone great in a senior role. Dont be afraid to ask questions and suggest bold ideas. The way you come up with and improve ideas is just as important as making themhappen.Consistency vs. InnovationDialpad has been around for a while, which means weve built up a lot of design patterns that are quick and easy to use. But lets not settle for quick and easy if theres room for improvement. Sometimes a new approach could make thingsbetter.When reviewing designs, I ask myself the following questions about consistency and innovation:Are we proposing a new solution to an old problem? Is there a goodreason?Do we have examples across the product that do something similar, while noting the pros andcons?Does the design use patterns customers are used to, or would it be something new theyd have tolearn?If there is an existing pattern that accomplishes a task, I want to see it. At the same time if you think a new pattern is warranted, I want to see that too. Bonus points if you have a reasoned preference towards one or theother.While fixing inconsistencies is important, we should also think about the users context. Sometimes its important to push for a better experience even if it means more work. Other times its better to stick with what we have for the sake of consistency and speed. Having multiple designs drawn out helps facilitate this discussion.Masking ComplexityDialpad is an incredibly complex product that contains a lot of technical workflows and generates a ton ofdata.Our customers shouldnt have to knowthat.Our aim should be to make communication instantly and easily understood, like a web browser does for connecting to and displaying websites.When reviewing designs, I ask myself the following questions about scaling afeature:Is the information presented in a screen easy to understand at a glance? Can the user go deeper if theywant?Does it involve too many clicks? Are we asking users to do things we could automate?How might a new user perceive this feature? What about a poweruser?Do we need to build something new? Or can we change something we alreadyhave?The design should be easily understood but robust enough to handle complexity andscale.UX CopyRemember design is mostly words. I want to see you hold UX copy to the same standard as user flows, rectangles, andcolor.When reviewing designs, I ask myself the following questions about UXcopy:Can there be less words? Whether its a blog post or a single form field, my first reaction when writing anything is to removewords.Is the language clear? Can we simplify or remove technical jargon, acronyms, and internalspeak?Is the language consistent? Will I understand this based on what I already know about Dialpad? Are we using verbs consistently? What about date and time formats? Even things like capitalization are important.We have copy guidelines and a ChatGPT bot trained in Dialpads voice and tone. Usethem!When writing copy, I usually put something like this intoChatGPT:Rewrite the following sentence 10 times. Keep it short, 10-15 words max.Use simple language and the rules you know for our UX copy."A poorly written sentence that gets my idea across."Usually the best copy is a combination of these examples. I used this technique when writing thisarticle.How Does ItFeel?When reviewing workflows, a prototype is worth a thousand static screens. I love seeing prototypes early, like even at the wireframe stage. It helps me preview what it would feel like to actually use thedesign.When reviewing prototypes, I ask myself the following questions:How discoverable is a feature? If I was new to the product, would I understand where to go and what todo?How does it feel? Is it a lot of clicks? How are the transitions betweensteps?How does it make me feel compared to other apps I use? How would I feel if I had to use this design every day? Everyweek?Its not always practical to prototype in code, so things like Figma prototypes offer the next best thing to how a design would actually feel in the product. From new user onboarding through to task completion, an interactive prototype gives me a decent idea of what the user journey would feel like from start tofinish.How Could ThisFail?Most design reviews focus on selling an idea, convincing the audience why a design is good. But thats only part of the equation. I want to know about the downsides too. We should be our own worstcritic.What are the pros and the cons of each design direction. How could a design fail? When reviewing a design, Ill be looking for reasons why it wont work or how it could be abused or exploited, so it helps if youve thought about this too. Thinking about this ahead of time helps facilitate the discussion amongst theteam.While not an exhaustive list of things that will make design good, thinking about these things ahead of time helps focus the discussion with leadership around around deep, meatytopics.When we embrace different definitions of good, lean on clear frameworks, and create a supportive space for critique, we can deliver design work that hits both creative and businessgoals.Subscribe to my newsletter to get more bit-sized thoughts on product design and leadership.How I review design work was originally published in UX Collective on Medium, where people are continuing the conversation by highlighting and responding to this story.0 Comments ·0 Shares ·69 Views
-
Decolonising AI: A UX approach to cultural biases and power dynamicsuxdesign.ccAn AI system is only as good as the stories it learns, but what happens when those stories are incomplete or one-sided?CunhatainAntropofagia musical, 2018Denilson BaniwaPicture by:DuHarteAI systems, trained on data reflecting historical power imbalances, often encode and reinforce inequalities, leading to discriminatory outcomes for marginalised communities.There is an urgent need to explore ideas on how to Decolonise AI, examining how UX can play an essential role in addressing cultural biases and power dynamics. deeply ethical and political one that requires interdisciplinary collaboration and a fundamental shift in perspective.The colonial echo in algorithmic designAI systems are not neutral arbiters; they are reflections of the data they are trained on and the values of their creators. Too often, this data is sourced from Western-centric datasets, embedding cultural assumptions and biases that disadvantage non-Western populations. This phenomenon echoes Boaventura de Sousa Santoss concept of epistemicide, where Western knowledge systems systematically suppress alternative forms of knowledge.Consider, for example, image recognition algorithms that struggle to accurately identify faces from diverse ethnic backgrounds, or natural language processing models that perform poorly on non-English languages or dialects. These failures are not simply technical glitches; they are manifestations of a biased system that prioritises certain cultural perspectives while marginalising others.Source: Humans Are Biased. Generative AI Is Even WorseBy Leonardo Nicoletti and Dina Bass Technology + EqualityJune,2023.The subaltern and the algorithm: Who gets tospeak?The issue of algorithmic bias is further complicated by the fact that marginalised communities often lack the power to shape the development and deployment of AI systems that affect their lives. Gayatri Chakravorty Spivaks seminal essay, Can the Subaltern Speak? (1988), highlights the challenges faced by marginalised groups in having their voices heard within dominant power structures. This resonates strongly with the issue of algorithmic bias, where certain voices are systematically excluded from the data used to train AIsystems.The consequences of this exclusion can be shocking. AI systems used in law enforcement, for example, have been shown to disproportionately target communities of colour. Similarly, AI-powered hiring tools can perpetuate existing inequalities by penalising candidates from non-Western backgrounds. Achille Mbembes Necropolitics (2003) examines how power operates through the control of life and death. AI systems, used in areas like law enforcement and border control, can become instruments of necropolitics, disproportionately impacting marginalised communities.Frantz Fanons work, particularly Black Skin, White Masks (1952), explores the psychological effects of colonialism and the ways in which it internalises feelings of inferiority and alienation. Similarly, Walter Mignolos, The Darker Side of Modernity: Global Futures, Decolonial Options (2011) exposes how coloniality is inherent to modernity, and remainsunseen.These insights can inform our understanding of how AI systems can perpetuate colonial patterns, even when they are not explicitly biased. To truly decolonise AI, we must challenge the concentration of power in the hands of a few tech companies and governments.We must promote the development of AI systems that are democratically controlled and accountable to the communities theyserve.AI Decolonial Manyfesto, pushing for a re-evaluation of AI through diverse cultural viewpoints, and Tierra Comn, which campaigns for interventions against data colonialism.UX as a tool for decolonisationUX, with its focus on people and empathy, can be a powerful tool for decolonising AI. By centring the experiences and perspectives of marginalised communities, UX teams can help identifying and mitigating biases in AI systems before it causes realharm.Questioning who AI truly serves and whose voices are missing from the design process, AI risks being shaped by a narrow set of experiences, reinforcing existing power structures rather than challenging them.The UX for Decolonial AI frameworkSome of the key strategies that UX practitioners can add to their frameworks to identify and prevent harmful experiences could relyon:Diversifying data sets: Actively seek out and incorporate data & feedback from diverse cultural backgrounds to ensure that AI systems are trained on a representative sample of the population.Engaging in participatory design: Involve members of marginalised communities in the design process, giving them a voice in shaping the development of AI systems that affect theirlives.Promoting transparency and explainability: Make AI systems more transparent and explainable, so that users can understand how they work and challenge potentially biased outcomes.Embracing cultural sensitivity: Design interfaces that are culturally sensitive and inclusive, taking into account the diverse needs and preferences of users from different backgrounds. Silvia Rivera Cusicanqui, a Bolivian sociologist, emphasizes the importance of chixi thinkingembracing contradiction and ambiguityin challenging colonial power structures. In AI, this could mean designing systems that acknowledge and celebrate cultural differences rather than striving for a single, universal standard.The pathforwardDecolonising human-AI interactions is a complex and ongoing process that demands a collective effort. By embracing a decolonial lens, we can design AI systems that are not only accurate and reliable but also truly inclusive.This requires us to recognise the colonial legacy embedded in AI by acknowledging the historical power imbalances that have shaped its development and deployment. It also means centring the voices of marginalised communities, prioritising their experiences and perspectives as those most affected by algorithmic bias.But notonly.How can we all commit to building an environment where AI empowers rather than marginalises?Decolonising AI: A UX approach to cultural biases and power dynamics was originally published in UX Collective on Medium, where people are continuing the conversation by highlighting and responding to this story.0 Comments ·0 Shares ·68 Views
-
My kids and I used AI to build a gameuxdesign.ccWe found the limits of the current generation of AItooling.Can modern AI dev tools enable kids (and an zany father) to build a mobile game on a snowday?SummaryTools like Replit and Vercel v0 can deliver impressive resultsThe tools struggle with accuracy, humans are better at finedetailsRight now, tools like the ones tested are best for getting to 80%doneTwo years ago, my kids and I built Simple Kid Games. As the name implies, the website has a few very simple web-based desktop HTML games designed bykids.The goal then was to teach my kids about technology and how to write HTML, JavaScript, and CSS. You can read about that adventure here.In what seems like a blink of an eye, AI has completely revolutionized the way we build technology. So, we thought wed expand Simple Kid Games by using AI to build a new mobilegame.Heres how it went building BirdDash.Presidents Day 2025 was a surprise snow day. We got to work in ourpajamas.Getting startedAll good projects start with a plan. Armed with markers and paper, the kids sketched ideas for a side-scrolling runner. They worked on level and character designs while I spun up Vercel v0 andReplit.I started a doc to capture our initial prompt as well as some reflections along theway.After a little trial and error, we settled on a collaborative approach to crafting the prompts based on real-time collaboration. The kids initially struggled to describe the mechanics of ourgame.Once we got rolling, and after a little help, they got more excited and skilled at suggesting prompts to make improvements to theapp.Like stealing candy from anLLMReplit and Vercel v0 are similar tools with similar capabilities. Both turn text prompts and files into functioning apps, all written in modern front-end code. Each tool also offers deployment and hostingoptions.The same initial prompt was used for each tool. We started withReplit.Replits Agent turned our initial prompt into a neat summary and offered new ideas (although it never did add sound effects).Replit took about 4 minutes to build the first version of the app from this initial prompt, asking us if we see the game and are able to useit:Replit: Can you see the infinite runner game with a black canvas and a white rectangle (player)? Are you able to make the player jump by pressing spacebar or touching thescreen?Humans: No theres nothing on the screen except the words distance: 0m and coins: 0. And nothing isworkingReplit: Ill check why the game canvas isnt showing up and fix the display and functionality issues.After that, we were actually amazed that there was a functioning game! It was rudimentary but functional and fun. The kids were delighted with the initialresults.The initial version of Bird Dash (then called Bird Runner) was functional and fun. There was one problem, the obstacles (red squares) were not at the same level as the character (white square) so you couldntfail.At this point, we decided to head to Vercel v0 and see what it could do. With the same prompt, we received a somewhat more transactional, though useful, response telling us the steps itd take to develop ourgame.Vercel v0 was a bit more straight to the point, telling us the steps it would take to build theapp.With a comical shrug from the kids (who cares, looks good), we hit enter and Vercel did its thing and returned an error. After telling v0 that we were kids and didnt understand what was happening, it fixed theboo-boo.We told Vercel v0 to treat us like kids and it fixed the scaryerror.Now, to Vercels credit, v0 was creating this app to run on a server with a database and probably some sophisticated routing and whatnot, but we were here to see how far we could get withoutcoding.Unfortunately, that was as far as we were able to get with v0. After a few tries to get a functional version of Bird Dash, we abandoned ship and headed back toReplit.After several attempts at getting something working, Vercel v0 delivered this gem. With more time, I suspect we could have made this functional, but these new tools are raising the standards of expectation prettyquickly.We did like a splash screen that Vercel decided to add to the game, so we took that idea and headed back to Replit. After instructing Replit to add said screen, the game was starting to lookgood.There was one problem, the character (represented by a white square) was not aligned with the obstacles on the stage (represented by red squares) so it was impossible to run into an obstacle.The white square, our character placeholder, would slide under the red obstacles.This became the most frustrating part of creating our game. We tried 7 different prompts to get the objects to align and appear on the same x-axis. We eventually got it good enough and movedon.After 7 different attempts, the purple bird and the spikey obstacle were aligned, but are rendered floating above the x-axis of thestage.Ready fortakeoffAfter a few more failed tweaksincluding trying our best to change the black background to white and adjust the stage placementwe decided good enough was good enough and deployed.Interestingly, out of this whole experiment, the most difficult thing was remembering my hosting account and resetting the SSL certificate for SimpleKidGames.com on Namecheap.After getting accustomed to Vercel and Replit, I dont know that Ill ever go back to traditional hosting solutionsa surprising outcome I hadnt expected out of this experiment.Both Vercel and Replit have built-in hosting and deployment options with cloud scaling and other features.Not great, but actually prettygreatIts amazing that Im dissatisfied with the results at all. The quality is not great, but then again, it would have taken me multiple days to get the game to this point with my ownhands.In 1.5 hours on a snow day, my kids and I were able to think of an idea for a game, experiment with a few different tools for building it, and ship a functioning version of the idea to ourwebsite.Give Bird Dash (the AI called it Bird Runner in the UI) a try at SimpleKidsGames.com and let us know what youthink.If dad was building this game, it would be much more accurate but take a lot longer time.MysonMy kids and I used AI to build a game was originally published in UX Collective on Medium, where people are continuing the conversation by highlighting and responding to this story.0 Comments ·0 Shares ·68 Views
-
Creative briefs: the best way to track design projects if youre short on timeuxdesign.ccHow to provide just enough context for your projectsContinue reading on UX Collective0 Comments ·0 Shares ·72 Views
-
Design yourself out of a job so youre ready for the next oneuxdesign.ccBefore taking your next role, figure out how and when you plan toleave.illustration by StorytaleEarly in my career, before I became a people manager, I would say that I knew it was time to leave a job when I was no longer learning. Learning my craft, new techniques or processes, how to manage clients or projects. As I grow in my career, I still want to learn, its a driving factor in each new role I take, but now Im much better at finding new things to learn without leaving a job. Now my focus is on teaching, what will I bring to a company, to my team in order to feel like Ive reached a level of success there, and know it's time to move on, from the role, the team, or thecompany.When I start a new job I immediately start the process of designing myself out of the role as soon as possible.Every company is different and what they need from you is as well. Sometimes you start, thinking youll spend your time building the team, or shepherding the product forward, sometimes you end up doing something entirely different What might those other things looklike?Establishing role standards for what is expected at each level of seniority, articulating the deltas between them to create a clear plan for growth and set expectations for performance reviews and promotionGrowing beyond legacy business metrics (funnels, basic click-through-rates, sales, cart value) into measuring actual user success, happiness, confidence, and frustration, atscale.Building links between functions, even successful products may still have chasms in the knowledge between the functional teams. Do engineers really know the design process? Do the designers understand how to read and interpret analytics dashboards. Respect, and eagerness to collaborate starts with understanding.What got you here wont get you there, when you join a team at an established company things could looks great, meetings may run smoothly, people get along, and its business as usual. Will that get you where you need to be as a company? If not, you may need to be the forcing function for process or organizational change.But upon learning more you realize the biggest impact you can have it something completely different, sometimes things that dont even feel likedesignillustration by StorytaleEarly in your career what are your goals? For many, its a specific title, a salary with a certain number of commas, a recognizable company name on your resume. These arent wrong, but youll get tired of them, I promise. One of the questions I ask when Im interviewing for a role or onboarding in a company is, what change in this organization would cause you to start looking for something new? Going into a role it's good to know what people consider core to their company and its culture, it's not that those things are unchangeable, but you have to be sensitive and intentful when planning changes in thoseareas.Challenge yourself now, what are your personal goal as a manager? For me its a team that wants me around but doesnt feel they need me. Its getting my top performers promoted. Its getting my most insightful team members to write, give talks, teach classes, and share their knowledge. Getting my most quiet and introverted to understand they can be leaders (and if they want, managers) as well, leadership isnt about speaking loudly in front of groups but in cultivating trust and influence.Further ReadingWhat Maslows Hierarchy Wont Tell You About Motivation by SusanFowlerHow to Motivate Your Problem People by Nigel Nicholsonillustration by StorytaleFor Individual ContributorsI would say to my team members, design yourself out of a job and well find you a better one. It can be scary for some people to take steps that could eliminate their own role, or make it no longer level-appropriate through process improvements or automation, they may be concerned about job security, of overstepping bounds, or simply not being recognized for the work outside the scope of their current assignedrole.Do the job of your manager (but ask first!), look at what takes up your managers time and figure out what parts of that feel like a step up in responsibility, visibility, or complexity for you, it could be logistical things like running a reoccurring crit, stand-up, or cross-functional sync meeting. Handling an intern programme or on-boarding process for new team members, taking the lead in planning an offsite, sprint, or other strategic team event. Take a more active role in one of these things, and then approach your manager in a 1+1 and ask if theyd be open to you taking it overcompletely. Let them know why you want to and how youll make sure they know the outcomes of it later. Focus on the win-win of them getting their time back to focus on something more appropriate to their skills and goals while you have the opportunity to work toward a stretch goal of yourown.Early in my career, especially at smaller companies you end up taking on every role; no Writer / Content Designer? That's you now. No Prototyper? also you. At bigger companies things tend to get more specialized, that doesn't mean you still cant step into the shoes of another function, find someone whos doing something interesting and ask to shadow them, see what they do, what tools they use, how they work with other function, and what their deliverables look like. Make sure to not miss any of the core requirements of your day-to-day job, and even if you dont decide to go down that path, youll always be better at working with that person and their function in thefuture.Real leadership has little to do with seniority, no matter what your role is, identifying something the company should be doing and isnt (or is doing and shouldnt) is a way that you can show leadership in your role, no matter how early in your career youare.Further ReadingArticulating your value as an individual contributor by CaioBragaMaintaining a growth mindset by EdOrozcoFor PeopleManagersWhat do manager goals look like? Shepherding a major project from concept to launch, growing a team in size, skill, or creating structure that helps get work done more effectively. Creating a safe and supportive space. These are just a few examples. For me, these tend to make the top of my list over and overagain:Identifying the big bets (and the critical small ones): As a manager, you need to be able to spot the opportunities that have the potential to significantly impact the company or team. But, just as importantly, its about recognizing those small, often overlooked projects that can provide immediate value, spark innovation, or refine processes. Both the big and small bets require careful thought, resources, and attention to execution.Finding who is bored and needs something new and challenging: Keeping your team engaged is one of your core responsibilities. People dont thrive in stagnation. Its important to stay attuned to the energy and enthusiasm of your team members. If someone feels uninspired or stagnant, its up to you to identify those individuals and work with them to find new challenges or opportunities for growth. This not only increases motivation but also helps retain toptalent.Identifying what parts of your job are appropriate to delegate: Effective delegation is a hallmark of strong leadership. As a manager, you cant (and shouldnt) do everything. The key is understanding which tasks can be handed off to others, whether they are operational or more strategic in nature. Delegating work helps empower your team, build trust, and also frees you up to focus on bigger priorities. Delegation doesnt mean abdicating responsibility, but rather giving others the space to grow while you concentrate on shaping the biggerpicture.Creating an environment where people feel safe to fail, and take on big challenges: A big part of your role is fostering a culture where risk-taking is encouraged, and failure is seen as an opportunity to learn. People are more likely to innovate and take on big challenges when they know its okay to fail and grow from their mistakes. You must lead by exampleshowing vulnerability and resilience in your own work, admitting when you dont have all the answers, and making it clear that trying and failing is a normal part of thejourney.Being okay with handing off some scary stuff: Often, as a manager, youll feel the instinct to protect your team or shelter them from high-stakes or difficult tasks. However, giving team members the chance to tackle challenging, sometimes intimidating, responsibilities helps them grow in confidence and skill. Stepping back and allowing them to take the reins builds trust, empowers them, and develops their leadership potential.Cultivating successors: As a manager, its your responsibility to not only build and strengthen your current team but also to prepare for the future. This means actively identifying high-potential team members and mentoring them into leadership roles. A good manager works themselves out of a job by cultivating successors who can step in and take over key responsibilities, ensuring that the team and the organization continue to thrive even after you moveon.Doing Your Managers JobEvery one-on-one you have with your manager is an opportunity to talk about what takes up their time and mental bandwidth. Its important to manage up and down. While youre focused on your own leadership responsibilities, dont forget that your manager likely has their own set of challenges and priorities. Understanding how you can help alleviate their burden or take on certain tasks can strengthen your relationship and demonstrate initiative. Ask them questions about what theyre struggling with, what projects need attention, and how you can help them make more strategic decisions. This transparency and collaboration make you more effective in your role and show that youre a valuable partner, not just a directreport.By understanding the nuances of your managers role and the pressure theyre under, youll become better at supporting them while also excelling at your own leadership tasks. A reciprocal relationship where both parties understand each others challenges and needs allows both of you to be more successful andaligned.illustration by StorytaleFind your motivation, here and elsewhereWork is a lot like dating. Each role, each company teaches you something new about yourselfwhat you like, what you dont, and how you collaborate with others. You get better at recognizing patterns in your own motivation, communication, and work style. Just like relationships evolve, so does yourcareer.When youre just starting out, motivation may come from tangible markers: promotions, titles, recognition. Over time, it shifts. The excitement of proving yourself fades, and what becomes more important is the impact youre making, the people youre mentoring, and the larger picture youre contributing to.You may notice patterns in the roles and companies that excite you. Some people stay in their first job or with their first employer for years, often out of comfort. But like any long-term relationship, it can lose its spark if growth isnt there. The trick is knowing when to move forwardwhen the job no longer challenges you or aligns with your evolvinggoals.Each job is just a chapter in your career story, and youre the one writing it. The key is to keep evolving, to stay connected to what drives you, and to be open to new opportunities that align with that. Whether its finding new challenges within your current role or seeking something entirely different, remember: motivation is not static. You get to define what success looks like as yougo.illustration by StorytaleHand off the scary stuff, not just the boringstuffI once heard being a Manager likened to being a janitor It's your job to do the things that need to be done, but that no one necessarily wants to do. Preparing for emergencies that may never happen, ensuring the mental space the team occupies is ready for anything, and doing the cleanup (backlog, documentation libraries, onboarding materials, processes, and contact methods, are always up-to-date and relevant) that keeps a team running smoothly.Its not always about success, sometimes its about trying! Its ok to fail, its ok to take the wrong path, its ok to waste time as long as youre learning from your mistakes. As a manager it can be tempting to be the one in the front of the room, representing your teams achievements, being the one who answers the hard questions from executive leaders, after all you have the most training, know these stakeholder better than anyone on your team, and you trust yourself. Dont do it! Step back, and shine the light on your team, be the note taker sitting at the edge of the room, sit in the eyeline of the people on your team presenting their work, there to offer a calming smile, a reminder to pause, breathe, and take theirtime.Role playing, and rehearsing presentations can be helpful mentally preparing your team members for difficult or challenging things they need to in the course of their work, but the reality is they may never get over their nerves (you might not either) and thats ok. Dont protect your team from every opportunity to get hurt or scared, instead, prepare them as best you can, and be there for them when they needyou.illustration by StorytaleLeave a legacy, but also leave someroomWhen choosing to leave a team or a company it can feel like you need to spell out everything for your successor, but being too detailed and too prescriptive can cause patterns to ossify. Its always a bad sign when you ask why things are done a certain way at a company and the only thing people can answer is its just the way its alwaysbeenWhen I worked at Google we had a saying that was a response to someone asking what the Google way of doing something was, wed say, whats the best way to do it? What we meant was that when you hire smart, creative people, making those people conform to some standard way of doing things didnt make any sense. Instead, every hire is an opportunity to improve, learn, and grow from that persons experience and expertise, no matter if theyve been working for 15 years or just graduated the month before. New ideas come from inviting people to try doing things differently, sometimes they work sometimes theydont.So how do you leave just the right amount of guidance? Unfortunately there is no formula, every team is different, but Ill outline some best practices.Make sure the team has evergreen goals and motivations, not just a roadmap and a mission statement. Work with your team to define a plan that is durable enough to weather changes to annual planning cycles, the whims of changing tech landscape, and the comings and goings of teammembers.Set up processes that improve quality, and are self explanatory, no one likes process for process sake. If youre clear about what the goal of a process is then later, people can improve on it, with the intent of getting even closer to the original goal of the processitself!Ensure people feel trusted and empowered, constantly reinforce that you, their manager, are not there to police them, to reprimand them, but to create an environment for their success, their growth, and to be their biggest champion. While it will be a shock, and possibly even hurtful to them when you leave, if they feel empowered, they will continue to create the environment that you fostered even withoutyou.Plan short and inspire long. So much can change in a company and with the product landscape in a very short time, finding the right balance between annual planning (too long!) and quarterly planning (too brief) is critical, work with your team to define longer term vision (multi-year), but plan work towards those goals at a much shorter timespan.Wrapping it allupAt the end of the day, the journey of being a design manager is about evolutionboth for yourself and the teams you lead. Its not just about building processes or hitting milestones; its about creating an environment where your team can thrive without you, where you can step away and see them continue to grow. Your impact is measured not just by what you accomplish, but by how you set others up forsuccess.Remember that the goal isnt to hold onto a job forever but to build a foundation that allows you to pass the torch smoothly, knowing that youve created something sustainable. Embrace the messiness of it allprocesses will fail, things wont go as planned, and there will be moments of doubt. But thats where growth happens. Both for you and yourteam.So design yourself out of a job, but also design in a way that leaves room for the next chapterone where you can look back and feel proud of what youve built and excited for whats tocome.Design yourself out of a job so youre ready for the next one was originally published in UX Collective on Medium, where people are continuing the conversation by highlighting and responding to this story.0 Comments ·0 Shares ·79 Views
-
A really (really) long but authentic history of design evolution in Indiauxdesign.ccTracing the journey of Indian design: from ancient traditions to contemporary innovations.Photo by Ankush Rathi: https://www.pexels.com/photo/brown-and-multicolored-concrete-building-925069/A few days back I was traveling to a city and noticed the political banners hosted in the city. I noticed something: each banners color pattern was different, and every banner represented a religion. If you live in India you know there are different colors for different religions like orange/saffron for Hindu, Green for Islam, etc. But as designer; I dont see religion in colors, but justcolors.Back home, I looked over the internet to understand this depictions origin. I came to know that- orange in Hinduism is used as fire, the color of the saints as most Hindus were saints back then. Similarly Green in Islam was represented as Jannah (a place with fertility after birth). These colors have been used so much in India that they have made the viewers perspective somewhat religious. Although, this usually does not affect any designeras designers are always playing with colors, they know the psychological effects of all colorsThis keeps them unbiased.Looking for these two colors took me to the origin of Indian color philosophy and I was now curious to understand more about Indian Design. What role does design play in India? How has design influenced India? How design is evolving in India? Then, I was thinking about-What is the design identity of India?When we say Indian designwhat people from other country see? What do they think about Indian design? Is it historical art, ornamental decorations and vibrant colors, or beautiful intricate architecture and carvings or any new tech? All these questions occupied my mind and I decided towrite.If you are a designer: when I say Japan, you suddenly transport in the world of Calligraphy Shodo (Japanese calligraphy, also known as shodo which means the way of writing, is an art form that combines brush and ink writing with poetry, literature, and painting. It is a revered part of Japanese culture and is used in everyday life and artistic pursuits) and minimal design on banners, lot of color and complex designs in urbanarea.https://dribbble.com/shots/7142386-Tokyo-Blends-posterLikewise, what is Indian design? This will be longish article with few details on evolution of design in India and I promise, by end of this article you will be definitely surprised and gather more insight about IndianDesign.The RootsThe oldest things in India are truly ancient. People have had to dig into the earth to find them!temples, caves, and rock carvings. These were built much earlier; in fact it is also known as prehistoric era. This Era was in 2500 BCE and earlier. India is one of the oldest country, on Internet it says its 500010,000 yearsold!We do see the paintings in caves, rock carvings made by early humans in India (According to Farnaz Broushaky (2016), evidence indicates that the Neolithic farmers form the main ancestry of many modern South Asians. They migrated from the fertile crescent, most likely from a region near the Zagros Mountains in modern day Iran, to South Asia some 10,000 yearsago.)We can still visit a few Rock shelters in India, the most popular one that you can visit is the Bhimbetka Rock Shelters in Madhya Pradesh. These were not made or constructed by humans; they are natural formations in the Vindhyan Hills of Madhya Pradesh, India. However, they became significant due to the early hominins and humans who used these shelters as habitation sites and canvases for their artwork. Evidence of early human ancestors living here date back to the Paleolithic period (around 100,000 years ago). The name Bhimbetka is derived from Bhim Baitka (Bhims sitting place) in local folklore, connecting it to the epic Mahabharata. This connection is mythological, but the shelters significance is primarily archaeological and anthropological. We cannot trace down who actually created this designs but we surely know that this was start of Graphic Design. Graphic: Derived from the Greek word graphiks (), meaning pertaining to drawing or writing, which itself stems from graphein (), meaning to write or todraw.SourceGoogleImagesSource: GoogleImagesThe emergence of TemplesTemples in India began to emerge much later, during the early historic period, around the Mauryan dynasty (322185 BCE) and more prominently during the Gupta period (4th6th century CE). These were influenced by evolving religious practices, particularly Hinduism, Buddhism, and Jainism. Few of early temple builders inIndia:Mauryan Period (322185 BCE)Emperor Ashoka was active in constructing stupas and rock-cut architecture, like the Barabar Caves. These were Buddhist structures, not temples in the classical sense but precursors to temple architecture.Satavahanas and Kushans (1st Century BCE3rd Century CE)Early rock-cut temples dedicated to Hindu deities started appearing, as seen in Ellora Caves and AjantaCaves.Gupta Period (4th6th Century CE)Known as the Golden Age of Indian Art, this era saw the first free-standing temples, such as the Dashavatara Temple at Deogarh. Temples in this period were made of stone and brick, with intricate carvings.Pallavas (4th9th Century CE)The Pallavas pioneered South Indian temple architecture with rock-cut temples like the Mahabalipuram ShoreTemple.Impression from the AjantaCavesThe Pallavas Art and ArchitectureOkay, so from History we got caves, temples, rock shelters, etc.Lets now talk a bit about Civilisation Design.The Indus Valley Civilization (Harappan Civilization)The Indus Valley Civilization, also known as the Harappan Civilization, was one of the worlds earliest urban cultures, thriving around 33001300 BCE in modern-day India, Pakistan, and Afghanistan. Its cities, like Harappa, Mohenjo-daro, Dholavira, and Lothal, were marvels of urban planning, featuring grid-like streets, advanced drainage systems, and well-defined zones for residential and administrative purposes. They used standardized baked bricks for construction, a practice that ensured durability and uniformity. The civilizations infrastructure, such as massive granaries and the Great Bath of Mohenjo-daro, highlights its focus on public utility and organization.Great Bath in Mohen Jo DaroSourceThe artistic achievements of the Indus Valley are equally impressive. Painted pottery with intricate geometric and natural motifs, expressive terracotta figurines, and the famous Dancing Girl bronze statuette reflect their creativity and craftsmanship. They also created exquisite jewellery using gold, silver, and semi-precious stones and carved intricate seals featuring animals, symbols, and script, likely serving both functional and cultural purposes. Though textiles have not survived, evidence suggests they used cotton and other natural fibers. Their designs blended practicality with aesthetics, often carrying religious or symbolic significance, such as motifs of bulls, peepal trees, and mythical creatures, underscoring their deep connection to culture and spirituality.Indus Valley CivilisationSourceAfter the Historian period, we began to see design patterns emerging in the form of cave art (like Warli art) or more ornamental motifs in architecture, featuring elements such as leaves, flowers, andbirds.Voila! First icons set of India! These were the symbols used while creating the Indus valley, I found no exact information on the internet about why these were made, who made them, and what purpose theyserved.Icons used in IndusValley.Recently The Hindu Published a very interesting news about this above icon set. Tamil Nadu Chief Minister M.K. Stalin announced a $1 million prize for experts or organisations that succeed in deciphering the scripts of the Indus Valley Civilisation for everyone to understand.The Colonial Influence (15th-19th Century)The colonial influence on design in India left a significant and lasting impact, blending traditional Indian aesthetics with European sensibilities.This period saw the introduction of new materials, techniques, and styles, which reshaped various aspects of Indian art, architecture, anddesign.If youve traveled to India, you may have observed buildings that blend traditional Indian architecture with European or British influences. However, throughout the country, there are also structures that deviate entirely from Indian architectural styles. The reason for this is colonialism.Several nations ruled over India and left a lasting impact on its architectural design. Here are a few ofthem.Portuguese Rule in India (15thCentury)The Portuguese were the first Europeans to establish a presence in India, arriving in 1505, following Vasco da Gamas historic voyage in 1498. They remained the last to depart, with their rule concluding in 1961. Although Portuguese colonial rule outlasted that of the British, its impact was relatively limited. Noteworthy remnants of their legacy include architectural landmarks like the Cathedral of Goa and other structures representing Portuguese India.Cathedral of Goa.SourceFrench Rule in India 17thCenturyThe French colonies in India were the smallest among the European possessions in terms of territory. The French first ventured into India in the 17th century, primarily for trade. They established their presence by acquiring Pondicherry from the Sultan of Bijapur and Chandannagar from the Mughal Governor of Bengal. These settlements became key hubs for French commercial activities in India. The most characteristic element of French architecture in India is the use of columns to support the roof and divide the building into sections. Dormers, Shutter windows, rounded towers or gables, sloping roofs, and chimneys were prominent features of the Indo-French architectural style.https://theholidaysdestination.com/puducherry-museum/The British Raj (18th-19th Century)Coronation of George V. Delhi Durbar and the royals.dailymail.co.ukThe British ruled the Indian subcontinent for nearly 200 years, culminating in Indias independence in 1947. During their administration, they blended Indian design elements with Western architectural styles, reflecting the regimes influence. Their rule began with the construction of extensive road and railway networks, primarily to serve their interests, and later included the establishment of significant landmarks such as monuments, railway stations, rest houses, and government buildings. Prominent examples of British colonial architecture include the Rajabai Clock Tower, CSMT (Victoria Terminus at that time), and the Bombay High Court inMumbai.Victoria Terminus is now known as Chtrapati Shivaji MaharajTerminusThe Portuguese, French, and British colonial powers had a profound influence on graphic design, typography, and print culture in India. Each brought unique aesthetics, techniques, and practices that shaped Indias design language and print traditions.Heres how their influence unfolded:The Portuguese were the first Europeans to establish a strong foothold in India (Goa in 1510), and their contributions laid the groundwork for print and typography.Introduction of the Printing PressThe Portuguese introduced the first printing press in India in 1556 in Goa, primarily for religious purposes. Early printed works were in Konkani and Marathi, using Roman script, as well as Portuguese.The press was used for publishing Christian texts, catechisms, and religious literature, making print culture accessible in local languages.TypographyEarly typographic designs reflected a fusion of Roman lettering and local Indian scripts.The Jesuit missionaries in Goa developed movable type for Indian scripts like Tamil and Malayalam to aid in spreading Christianity, influencing Indian language typography.Visual DesignThe graphic style of Portuguese print leaned heavily on religious iconographysaints, crosses, and baroque ornamental patternsreflecting Catholic art traditions.Left:The Christ Child as Good Shepherd (Good Shepherd Rockery), Indo-Portuguese, ivory, seventeenth century, 17.4 6.8 5 cm, place of origin: India (Goa) (The Walters Art Museum); right: Virgin of the Immaculate Conception (Our Lady of the Immaculate Conception), Singhalo-Portuguese, ivory, seventeenth century, 25.7 cm high, place of origin: Sri Lanka (The Walters ArtMuseum)First printed book in Tamil in 1579,CochinImage SourceFrench InfluenceThe French influence on Indian typography and graphic design was less widespread but concentrated in areas like Pondicherry.Typography and DesignFrench colonial administrators brought a neo-classical aesthetic, characterized by elegant, serif-based typography and restrained graphic design. They influenced the development of Tamil typography, especially for religious texts and educational materials, creating a refined typographic tradition.Transliteration of vowels and consonants from Tamil to French (Lap Reference Lap1904,7)Printing PressesFrench presses in Pondicherry produced bilingual works (French and Tamil), fostering cross-cultural exchange.The design of printed materials often combined French minimalism with Indian motifs, creating a hybrid aesthetic.Visual IdentityFrench influence can still be seen in signage, graphic styles, and architectural typography in Pondicherry, where colonial design sensibilities merged with localculture.The British had the most extensive impact on graphic design, typography, and print in India, driven by industrialization, commerce, and education.The British established printing presses across India to produce newspapers, books, and official documents.Pioneering typographers like John Gilchrist and William Carey worked on adapting Indian scripts like Devanagari, Bengali, and Urdu into movabletype.John GilchristWilliam CareyIndian scripts were standardized and streamlined for mass printing, creating the foundation of modern Indian typography.The first Indian newspaper, Hickeys Bengal Gazette (1780), marked the start of print journalism. English newspapers and bilingual publications brought European typographic styles (serif fonts, grid layouts) into Indian printculture.Hickys Bengal Gazette March,1781During colonial times, lithography played a significant role in shaping art, culture, and communication in India. Introduced by the British in the early 19th century, this printing technique was initially used for administrative purposes like producing maps, official documents, and educational materials. Over time, Indian artisans and publishers adopted it, transforming it into a medium for printing books, newspapers, and journals in regional languages, which helped promote literacy and cultural identity.Lithography also revolutionized Indian art, making religious and traditional imagery more accessible to the masses. Artists like Raja Ravi Varma used lithographic presses to reproduce their work, blending Indian themes with European styles and reaching a wider audience.Beyond art and literature, lithography became a powerful tool in the independence movement, allowing the production of pamphlets, posters, and newspapers that spread nationalist ideas. The technique reflected a unique cultural fusion, combining European methods with Indian creativity, and its legacy continues to influence Indias visual and print heritagetoday.To know exactly, what is lithography checkout this quick video by TheoLotzI remember when I was kid I used to see the Raja Ravi Vermas painting on our calendar, every month had a different painting. And then later I saw the same paintings during my drawing elementary and intermediate exams. Raja Ravi Verma and various artists have leverage their art while using the lithographic drawings.Lithography Print by Raja RaviVermaVisual communication played a key role in spreading the message of boycotting British goods and supporting native industries. Designs from this era prominently featured traditional Indian symbols like the spinning wheel (charkha), the lotus, and Bharat Mata (Mother India), which evoked pride in Indias cultural heritage. Posters, handbills, and advertisements promoting locally made products used bold imagery and vibrant colors to capture attention, while typography blended traditional Indian styles with modern techniques to represent the fusion of tradition and progress.Impressions from the SwadeshiMovementPublications, calendars, and packaging design also embraced Swadeshi themes, with nationalist imagery appearing in newspapers, magazines, and khadi products to reinforce the movements message. Artists like Abanindranath Tagore, associated with the Bengal School of Art, rejected European aesthetics in favor of traditional Indian techniques, creating a distinct visual language aligned with Swadeshi ideals. The graphic design of this period not only supported the independence movement but also laid the foundation for Indias modern visual identity, showcasing the power of design as a means of cultural and political expression.The Swadeshi Movement of the early 20th century had a significant influence on Indian graphic design, which became a powerful tool for promoting the movements ideals of self-reliance and national identity.Popular 1930s poster depicting Gandhi using a charkha to spin cotton and weave cloth, captioned Concentrate on Charkha and SwadeshiPost-Independence Period (19471980s)After gaining independence in 1947, India concentrated on establishing its identity as a sovereign nation. This aspiration shaped various design domains, including architecture, graphic design, and crafts. The Nehruvian focus on industrial growth and modernization significantly influenced the design landscape of the era. Alongside modernization efforts, there was a strong drive to renew traditional crafts and indigenous practices, creating a balance between preserving heritage and embracing progress.Post-independence Development in Architecture designLe Corbusier and Jawaharlal Nehru (18891964), Prime Minister of India (19471964) at the initiative of the founding of Chandigarh.Pandit Jawahar lal Nehru and Le Corbusier planning the design of Chandigarh City.Modernism: Influenced by Le Corbusier and Bauhaus, modernist architecture became prominent. Le Corbusiers designs for Chandigarh (1950s) set a benchmark for modernist urban planning.Public Institutions: Institutions like the Indian Institute of Technology, Kharagpur and government buildings reflected functional, minimalist design with an emphasis on concrete and openspaces.Housing for All: Low-cost housing projects were developed to address the needs of a growing population, showcasing utilitarian designs.Post-independence Development in Industrial designDesign in industries began focusing on affordable, functional products for everyday use. ForinstanceHINDUSTAN MOTORS LTD Ambassador (1958) Indias first locally manufactured car. Godrej Consumer Products Limited Steel Cupboards: Durable and cost-effective storage solutions.White Goods: Companies like Bajaj Electricals Ltd and Godrej Consumer Products Limited produced refrigerators, fans, and scooters designed for the Indian middleclass.Post-independence Development in GraphicdesignThere was a tremendous growth seen in the Graphic design sector, Institutions were establised and the people were more eager to learn new this that the nation is providing. During this time in 1961, National Institute of Design was establised to formalise the design education.One cool thing about NID is its logo! The NID logo was designed by Adrian Frutiger, the same person who designed the fonts like Univers, Frutiger andAvenirAdrian Frutiger and the NIDlogoAlong with the Institutions, there were various Projects were going on including the Indian Railways Logo designDuring this point the government of India recognized the potential of professional design to elevate the public sector branding. Designers, possibly involving collaborations with or inspiration form NID alumni, were tasked with creating the Indian Railways logo IndianRailwaysAnother interesting project was the Tricolor flag of India. The demand for national flag emerged during Indias freedom struggle as a unifying symbol against British colonial rule. Various flags were used in protests and rallies, each representing culture and religious Identities.Its believed the the first national flag was hosted in 1906 in Calcutta (Now Kolkata). It featured horizontal stripes of green, yellow and red with symbols like the sun, crescent moon andlotus.In 1916, The Home Rule Movement was initiated by Bal Gangadhar Tilak and Annie Besant, this moment sought a greater self-governance within the British Empire. Thus we see red (Hindu) and green (Muslim) stripes with Union Jack on top left. There were seven stars in the flag that represent the Saptrishi, a constellation deeply rooted in Indian mythology and culture, signifying Indias spiritual heritage.In 1921, Mahatma Gandhi suggested a flag to represent Indias diversity during the 1921 Congress session. Pingali Venkayya, a freedom fighter and agricultural scientist, presented a design featuring a red stripe for Hindus, a green stripe for Muslims, and a white stripe for other communities, with a spinning wheel (Charkha) at the center symbolizing self-reliance.In 1947, The Charkha in the 1931 design was replaced by the Ashoka Chakra, a 24-spoked wheel from the Lion Capital of Ashoka (Sarnath), representing dharma (righteousness), law, andmotion.Digital Revolution aka IT Boom (1990sEarly2000)This was a transformation period for India, being an independent country and in a race for growth, everyone knew that digital transformation is the next big thing, we made rapid advancements in technologies, government policies and emerged our digitaleconomy.This was a phase that laid the foundation of IT in India. In 1991, India liberalized its economyintroducing a new economic policy that drastically reduced government controls, opened up the market to foreign investment, and allowed for greater private sector participation. The rise of IT tech giants like Infosys, Wipro, Tata Consultancy Services provided a stage for Indias software and outsourcing.InfosysCompany History & Defining Milestones | AboutUsDuring this time a roadmap for IT was created by the National Task Force on Information Technology and Software Development. It was established by the Indian government to formulate a national policy aimed at transforming India into an IT superpower by creating a comprehensive strategy to integrate Information Technology across all sectors of the economy, building necessary infrastructure, and promoting the use of IT for national growth; essentially, to position India as a leader in the global ITmarket.The Government has entrusted the task force with a job to excite and energise the people of India, creating the faith in them that information technology vitally aids personal growth and nationalgrowth.Spread of Internet inIndiaThe first public internet service in India was launched by VSNL (Videsh Sanchar Nigam Limited) in 1995, marking the introduction of internet access to the general public, although it was initially very expensive and slow due to dial-up technology; essentially, this meant that most people could only access the internet for a limited time at a highcost.If you want explore internet journey of India in detail, please read refer to this article by GayatriVinayakFirst call inIndiaIn 1995, Jyoti Basu, the Chief Minister of West Bengal, and Sukh Ram, the Union Communications Minister, made history by becoming the first official cellphone users in India. They inaugurated the countrys first cellular service, Modi Telstra, with a phone call between Calcutta andDelhiDialling up: Mobile telephony started with then West Bengal CM Jyoti Basu saying the first hello to then Union communications minister Sukh Ram. Also seen in the picture is industrialist BK Modi (filephoto)New Telecom Policy marked a turning point in Indias telecom sector. By opening the industry to private competition, it significantly reduced costs, making mobile phones more accessible to a wider population and transforming the way people connected and communicated. With increasing access to internet and making the it affordable for everyone attracted the people of India, slowly people started learning to use technologies, implemented them in work and India became hub for IT services, benefiting from English proficiency and skilledlabor.The Y2K bug, or the Millennium Bug, was a computer programming issue that arose because many older software systems stored years using only the last two digits (e.g., 99 for 1999). As the year 2000 approached, there was widespread concern that computers would interpret 00 as 1900 instead of 2000, leading to errors in date-based calculations, financial transactions, and critical infrastructure systems.Who Solved It? The Y2K problem was a global challenge, and multiple countries played a role in addressing it. However, India played a particularly significant role in fixing the Y2Kbug.Contemporary Era (2000sPresent) of IndianDesignThe 21st century has witness a very dynamic transformation in design, blending the traditional aesthetics with modern digital technology, with rise of globalisation and growing creative sector, India has expanded beyond print and advertising to include UX/UI, motion graphics, AR VRetc.The 2000s marked the shift with companies focusing on startups and user experience, with increasing number of mobile phones and internet.UX/UI design became the central to product development. Apps like Swiggy, Paytm, Zomato and Flipkart has really pushed Indian designers to adopt global standards while catering to local user behaviour.Branding and VisualIdentityContemporary Indian design has moved towards bold, minimalistic and cultural branding. Brands like Paperboat, FABINDIA LIMITED, Royal Enfield have successfully merged the nostalgia with the modern aesthetics. While the new age start ups like CRED, Dunzo and boAt Lifestyle focuses on future and experimental visual styles. Bira 91 Bira has seamlessly blended Indian cultural motifs with contemporary aesthetics, incorporating vibrant colors, folk art-inspired illustrations, and playful typography to create a distinct and youthful brand identity that resonates with modern India.This fusion of Indian motifs with global design trends has given rise to unique brand identities.The introduction of regional typography and multilingual interface became important in making digital products accessible for everyIndian.Bira 91The brand with IndianEditionCred app design2022If you want to read more about the process of Bira design, checkout the Packaging design journey page by NH1DesignIf you want to read more about CRED design, there is relaly good article you can read, Thoughts on Creds UI revampNeoPOP (Apr 2022) written by Shaunak BhanarkarThanks for reading (it was alot!)Having explored the origins and evolution of design, we can see that it is fundamentally a visual transition.Design influences every Indian in profound waysour surroundings, culture, and history shape how we perceive and remember visual elements. Just as hearing an old song evokes nostalgia and transports us to moments from the past, design has a similar impact. It is s also science. If youre a designer, its essential to understand that whatever you create doesnt just impact individualsit contributes to the design identity of an entirecountry.How do you want your designs to represent India? Should they express professionalism, playfulness, or a balance ofboth?Indias design language is unique because it doesnt adhere to a singular genre. Instead, it embraces a diverse and vibrant aesthetic, reflecting the countrys rich culture. This variety makes Indian design more inclusive, adaptable, and deeply connected to itspeople.In my next article, Ill explore how even the smallest design choices can have a huge impact, eventually shaping the visual identity of a nation. Until then, keep designing with purpose andpassion!Sourceshttps://www.britannica.com/topic/Hindu-templehttps://www.incredibleindia.gov.in/en/maharashtra/chhatrapati-sambhaji-nagar/ajanta-caveshttps://en.wikipedia.org/wiki/Pallava_dynastyBuilt to Last: The Enduring Legacy of Pallava Art and Architecture | Asia ResearchNewshttps://www.thetalentedindian.com/the-indus-valley-civilisation-an-artistic-cultural-legacy/https://www.novatr.com/blog/architectural-influence-of-colonization-in-indiahttps://sundayguardianlive.com/art/4421-how-ravi-varma-s-popular-litho-prints-changed-indian-art-foreverhttps://www.visvabharati.ac.in/abanindranathtagore.htmlhttps://chandigarh.gov.in/know-chandigarh/general-information#:~:text=Chandigarh%2C%20the%20dream%20city%20of,the%20twentieth%20century%20in%20Indiahttps://www.thehansindia.com/life-style/history-of-tiranga--the-evolution-of-indian-tricolor-600061A really (really) long but authentic history of design evolution in India was originally published in UX Collective on Medium, where people are continuing the conversation by highlighting and responding to this story.0 Comments ·0 Shares ·77 Views
-
AI needs designers, accessibility affordances, I dont know where I fit anymoreuxdesign.ccWeekly curated resources for designersthinkers andmakers.Its easy to feel overwhelmed by the urgent need for AI ethics. AI is a versatile and dynamic field, capable of performing a wide range of tasks that can help solve real-world problems. However, algorithmic systems often reflect and amplify existing societal biases. Until interfaces clearly communicate the logic behind these algorithmic decisions, users wont be able to hold these systems accountable.Why AI (desperately) needs designers By BettinaD'vilaMoving away from order-taking culture [Sponsored] Join leading experts as we challenge the order-taking stereotype head-on and examine how to transcend it, positioning UX researchers as strategic advisors. Well also look into how research visibility can act as a catalyst to drive thischange.Editor picksDesign has changed and I dont know where I fit anymore After two decades in design Im now questioning my place.By PascalPotvinWere all going to need accessibility affordances one day UX probably needs to think more about aging.By DaleyWilhelmMedium is hiring a Principal Product Designer An immense, rare opportunity to shape the future of Medium.By CassieMcDanielThe UX Collective is an independent design publication that elevates unheard design voices and helps designers think more critically about theirwork.A genealogy of technology and power since 1500Make methinkSocial media is a trap Some people believe that social media offers some semblance of permanence. They become attached to their body of work, their content. Their profiletheir personabecomes their identity, and the place where they can make or remake themselves.AIs and robots should sound robotic But there is something fundamentally different about talking with a bot as opposed to a person. A person can be a friend. An AI cannot be a friend, despite how people might treat it or react to it. AI is at best a tool, and at worst a means of manipulation.The future belongs to idea guys who can just do things There, I said it. I seriously cant see a path forward where the majority of software engineers are doing artisanal hand-crafted commits by as soon as the end of 2026. If you are a software engineer and were considering taking a gap year/holiday this year it would be an incredibly bad decision/time to doit.Little gems thisweekA designers dilemma: how to deal with disruptive tech like Deepseek? By DarrenYeoOpen AI Operator: when AI takes the drivers seat By PatrickMorganAre Duolingos AI video calls just a gimmick or a valid way to study? By MaryBorysovaTools and resourcesYour brain has been tricked, and its loving it Is design a game of manipulation and lies?By Himanshu BharadwajHow Im using AI to streamline persona and journey creation Unlocking efficiency and creativity.By KyleSoucyDesigning for AI engineers UI patterns you need to know.By EveWeinbergSupport the newsletterIf you find our content helpful, heres how you can supportus:Check out this weeks sponsor to support their worktooForward this email to a friend and invite them to subscribeSponsor aneditionAI needs designers, accessibility affordances, I dont know where I fit anymore was originally published in UX Collective on Medium, where people are continuing the conversation by highlighting and responding to this story.0 Comments ·0 Shares ·51 Views
-
Interaction design demands more than visual creativityuxdesign.ccOur field has changed; update yourself.Continue reading on UX Collective0 Comments ·0 Shares ·64 Views
-
What the British Government Can Teach You About UX Writinguxdesign.ccSober, self-conscious, clear copy at its finestexploring GOV.UK.Continue reading on UX Collective0 Comments ·0 Shares ·88 Views
-
The utility-over-usability effect explains why bad UX persistsuxdesign.ccThe more essential a product is, the more users tolerate poor usability.For years, UX designers have pushed the idea that usability is everything. The common belief is that a smooth, intuitive experience leads to engagement, retention, and conversions, while bad UX drives users away. And in many cases, thatstrue.However, during my 14 years as head of design at an medical publishing company, I learned something that challenged this assumptionwhen a product, service, or content is highly valuable or necessary, users tolerate badUX.I define this concept as a new UX framework called the Utility Over Usability Effectthe idea that utility (the value of what is being offered) sometimes outweighs usability (the friction required to engage withit).Usability vs. Utility Quadrant Diagram | Illustration byauthorThe Usability vs. UtilityQuadrantThe Utility Over Usability Effect becomes clearer when we break products into four categories based on their utility (how necessary they are) and usability (how easy they are touse).High Utility + High Usability IdealThe gold standard. These products are both essential and intuitive, making them a joy to use. Users rely on them daily with little friction.High Utility + Low Usability TolerableUsers struggle with the experience but keep using it out of necessitythink government portals, medical software, legacy enterprise tools, or even innovative platforms like Amazon andAI.Low Utility + High Usability SuperficialThese products may look great and function smoothly, but they dont provide enough value to be indispensable. If a better alternative comes along, theyre easily replacedlike flashy tech gadgets that fade quickly or apps with great UX but no realdemand.Low Utility + Low Usability ObsoleteA complete failure. These products are neither useful nor easy to use, leading to inevitable abandonment. If something isnt needed and its a pain to use, it wont survive forlong.Understanding where a product falls in this quadrant explains why some frustrating experiences persist while some beautifully designed productsfail.How I Discovered This PhenomenonAs part of my role at the medical publishing company, I not only evaluated our own offerings but also conducted in-depth competitor analysisassessing design choices, branding, marketing strategies, and overall user experience. Our primary focus at the time was professional oncology news and education.During my research, a clear pattern emergedmost oncology-related content platforms, including ours, suffered from frustrating UX issues. Overcomplicated sign-in processes, cluttered interfaces, and poor information architecture yet medical professionals continued using them despite theseflaws.Oncologists werent choosing platforms based on the experiencethey were choosing based on necessity. The information was critical to their work, and with so few alternative sources available, they put up with the friction.The same was true for our own products. Our digital oncology content held exceptional value because it was developed in collaboration with leading oncology societies and institutions. Physicians relied on it not for its UX, but for its credibility.This led me to a key realizationusability wasnt a significant factor in driving engagement and retention. The real reason users stuck around was the utility of thecontent.This insight reshaped my understanding of UX. While usability plays a major role in adoption and satisfaction, its not always the deciding factor. If the perceived or actual value of a product is high enough, users will tolerate poor usability rather than abandon something they genuinely need.A New Perspective on Why Users Endure BadUXMy experience in the medical publishing industry helped establish the principles behind the Utility Over Usability Effect. However, the notion that users accept bad UX under certain conditions isnt newconcepts like Jakobs Law and The Network Effect also demonstrate how familiarity or network-driven value can make users overlook usability issues.Jakobs Law states that users expect interfaces to work like the ones theyre already familiar with. This means they often resist changeseven when usability is improvedbecause relearning a system feels harder than sticking with an inefficient one.Similarly, The Network Effect makes usability a secondary concern when a platforms value comes from its ecosystem. Products like LinkedIn or Microsoft Teams retain users despite frustrating UX because leaving means losing access to essential connections, content, or workflows.While these ideas explain specific reasons why users tolerate bad UX, they dont fully capture the broader dynamics of the Utility Over Usability Effecthow the balance between intrinsic value and usability determines whether users endure friction or seek alternatives.The Effect in the RealWorldThe Utility Over Usability Effect isnt a unique phenomenon. Some of the biggest and most widely used platforms in the world have thrived despite usability issues, simply because they provide something people cant get anywhereelse.Amazon, for example, grew into an e-commerce giant while offering an experience that was anything but smooth. Its early design was clunky, its navigation chaotic, and even today, its interface remains overloaded with features. But that never stopped people from shoppingthere.Amazon homepage with 15 tabs (2000)Source: humanfactorsblog.orgThe reason Amazon dominated wasnt because of its user experienceit was because of what it offeredan unmatched selection of products, fast shipping, and competitive pricing. The sheer utility of the platform outweighed its usability flaws.The same principle applies to professional software. Adobe products have been criticized for years due to their complex menus, inconsistent workflows, and feature bloat. Photoshop, Illustrator, and InDesign are all known for their steep learning curves, yet they remained industry standards fordecades.Designers didnt keep using them because they loved the experiencethey used them because, for a long time, there were no viable alternatives. Adobe owned the market, and professionals had no choice but to adapt to its difficult UX. Only in recent years, with the rise of competitors like Figma, has this grip started toloosen.Even today, were seeing this Utility Over Usability Effect play out in the AI space. Have you attempted creating images using MidJourney? Or simply tried navigating OpenAIs platform? The UX of these tools can be frustratingMidJourney requires users to generate images through Discord with cryptic prompts, and OpenAIs interface can feel counterintuitive attimes.Yet, people tolerate the friction because the technology itself is highly valuable and new. When innovation is fresh and groundbreaking, users are willing to endure bad UX because the utility outweighs the experience.When Theres No ChoiceGovernment, Universities, and Bureaucratic SystemsThe Utility Over Usability Effect is also easy to spot in areas where users have no alternative but to push through bad UX. Universities, government websites, and corporate portals are notorious for poor usability, yet people continue to usethem.Applying for a business license, registering for college courses, or submitting a grant application often means navigating confusing and unintuitive systems. But since these services are necessary, users endure the friction because they dont have anotherchoice.When I was pursuing my graduate degree, I was blown away by how awful the usability of the FAFSA website was. The Free Application for Federal Student Aid (FAFSA) is required for most college enrollments and despite its convoluted design, students have no choice but to endureit.The Illusion of UsabilityWhen Utility and Aesthetics Mask PoorUXWhile the Utility Over Usability Effect explains why users tolerate bad UX when the value is high enough, theres another layer to this phenomenonone that designers and product teams oftenexploit.Sometimes, a product isnt truly usable, but it feels usable because it combines high utility with strong aesthetics. This creates the illusion of usability, where users perceive an experience as smoother than it really is simply because it looks polished and provides something theyneed.This ties into the Aesthetic-Usability Effectthe psychological principle that people perceive aesthetically pleasing designs as more functional and user-friendly, even when they arent. In other words, users subconsciously equate good design with good usability, even if the experience is frustrating.Apples early skeuomorphic designs (Source: iphoneincanada.ca)Think about Apples early skeuomorphic designs. They werent necessarily easier to use than competitors, but they felt intuitive because they mimicked real-world objects. Or consider Teslas touch-based controlsthe interface looks sleek and modern, but in practice, replacing physical buttons with a screen often adds friction rather than reducingit.This illusion works because users associate beautiful design with ease of use. If it looks easy, it must be easy. However, this effect has limits. If usability flaws become too apparentlike Tesla drivers struggling to adjust basic settings while drivingthe illusion breaks. But as long as a product remains high-utility and visually refined, many users will tolerate the friction.Why This Effect Matters for UX DesignersMany UX professionals operate under the assumption that improving usability is always the path to better engagement. But The Utility Over Usability Effect challenges thatbelief.When users keep returning despite bad UX, it often means theyre doing so because they need the product, not because they enjoy the experience. In industries where content depth and functionality are more important than visual appeal or seamless interaction, improving usability might not be a primaryconcern.That said, this effect has a natural limit. As soon as an alternative emerges that offers the same level of utility with better usability, users will make the switch. Microsoft Word was once the default for document creation, but Google Docs gained traction by offering a simpler, more collaborative approach.Leveraging This Effect as a Strategic AdvantageFor companies operating in high-utility, low-usability spaces, The Utility Over Usability Effect can act as a buffer for experimentation. When users rely on a system due to its necessity, businesses may feel they have room to test unrefined features, roll out major interface changes, or even deprioritize usability in favor of other businessgoals.When I worked for the medical publishing company, I did this regularlyblasphemy, I know. We had several high-value content platforms, giving us the luxury of rolling out live feature updates and gathering direct feedback and metrics. To be honest, nothing truly replaces real audience feedback andtesting.However, this is a double-edged sword. While platforms like Amazon, Google, and Adobe have managed to make controversial UX decisions without immediate user drop-off, history shows that usability problems can accumulate until they create an opening for disruption.Take Amazon, for examplethey didnt ignore usability forever. Over time, it introduced one-click purchasing, streamlined checkout processes, and personalized recommendations to improve the experience.This means companies can experiment, but they shouldnt assume users will tolerate bad UX indefinitely. The moment a competitor offers equal utility with better usability, the patience of even the most locked-in users will wearthin.Resource Allocation and Market ConsiderationsFor companies with limited resources, the Utility Over Usability Effect suggests that prioritizing usability investments should depend on how vital or unique the product is within itsmarket.If a product operates in a saturated market with strong competition, usability becomes a crucial differentiatorusers will gravitate toward the option that offers both utility and a seamless experience. However, if a product serves a niche, high-necessity market with little competition, companies can often get away with investing less in UX, as users will endure friction out of necessity.This might not be what most UX professionals want to hear, but businesses think in terms of ROI. When usability isnt the primary reason users engage, companies may allocate resources elsewhere, such as expanding features, improving infrastructure, or increasing content depth. However, this is a calculated riskone that works until a competitor proves that usability can be a differentiator.The KeyTakeawayThe Utility Over Usability Effect is a reminder that bad UX isnt always a dealbreakerif the perceived value of a product is high enough, people will endure friction to access it. But that tolerance only lasts as long as theres no better alternative.For companies operating in high-utility spaces, this effect can sometimes provide room for experimentation. When users rely on a product out of necessity, businesses may have more flexibility in testing changes or rolling out unpolished features without immediate drop-off. However, this is only a temporary advantage.A product that thrives despite poor usability is operating on borrowed time. The question isnt whether usability mattersits whether usability is keeping users engaged or whether its simply being tolerated.If users are putting up with a frustrating UX because they have no other choice, its only a matter of time before someone else gets it right. And when that happens, usability stops being an afterthoughtit becomes the decidingfactor.Dont miss out! Join my email list and receive the latestcontent.The utility-over-usability effect explains why bad UX persists was originally published in UX Collective on Medium, where people are continuing the conversation by highlighting and responding to this story.0 Comments ·0 Shares ·89 Views
-
How Im using AI to streamline persona and journey map creationuxdesign.ccUnlocking efficiency and creativity.Example persona and journey map created by KyleSoucy.While AI has the power to enhance our work, it cannot replace the essential insights gained from conducting actual UX research and analysis.AI is a tool that helps us get the job done more efficiently; it does not replaceus.With over 20 years of experience in UX research, I was initially skeptical about integrating AI into our research practices. However, Ive found that Generative AI (GenAI) tools like ChatGPT, Google Gemini, Microsoft CoPilot, Claude.ai, etc., are immensely helpful. They do more than just assist in analyzing data to uncover insights; they also excel at transforming these insights into effective deliverables such as UX personas and journeymaps.There are many ways to write AI prompts to create UX personas and journey maps. Just google How to use AI to create UX personas? and youll find plenty of resources (some of which Ive used and cited at the end). So, why am I writing this article if theres already a lot out there? After a year of research and experimentation trying out several variations, Ive discovered a successful approach using a mix of prompts with unique nuances that dont appear to be covered in anything Ive read. So, I wanted to share my findings in the hopes that they could also help other fellow UX researchers. As our field continues to evolve, sharing our findings and fostering collaboration will be essential to unlocking the full potential of AI in UX. On that note, I invite you to share your experiences in the comments section if youve tried any of these prompts or something different that yielded greatresults.PersonasCreated by author via Canva.comThere are two main types of UX personas: those based on actual user research and those based on assumptions. Whether youre creating data-informed personas or assumptions-based personas (a.k.a. proto-personas, ad-hoc personas, alignment personas, etc.), GenAI can help immensely. Side note about proto-personas: In my experience, many people outside the product team dont understand the difference between personas and proto-personas. Therefore, when creating proto-personas, I prefer to use the term assumptions-based personas so its clear that research still needs to be conducted. Theres nothing worse than presenting a proto-persona and having stakeholders think theyve checked the persona box and can move on without conducting actual user research!If youre interested in learning more about how to create proto-personas, I highly encourage you to check out Tamara Aldins great work on alignment personas.Even when a persona is based on user research, the distilled information is presented in a fictional way while still accurately describing the average user of the product. Whether its a name, background story, tagline, etc., these made-up elements help bring a persona to life, making it authentic and memorable while enhancing its ability to resonate with the audience. I dont know about you, but I always struggle with writing these fictional bits of a persona, and its where I get the most help fromGenAI.After conducting research and analysis, I know what data I want to include in a persona and how it should be segmented, but I struggle with the best way to convey it. Some of you may consider this the fun part of persona creation, but personally, I can spend hours just spinning my wheels on coming up with the right tagline. Im a data nerd and love qualitative analysis, but I dont necessarily consider myself the most creative writer, which can sometimes make a persona truly meaningful. Its not to say that I cant get there alone, but it takes me much longer than someone with this gift. EnterAI!Recently, Ive been experimenting with using HeyMarvin, ChatGPT Team, and other GenAI tools to help me fill in the creative writing blanks that I typically struggle with when creating personas. To use AI in this way, Ive found that its most effective if you do the heavy lifting yourselffirst.Step 1: Gather all your research data andinsightsCollect all your interview transcripts and/or research reports if youve conducted user research. If youre doing proto-personas, you hopefully have a lot of secondary research documents to analyze (brand strategy & industry reports, EOY reports, previous research reportssurvey results, market research reports, customer service reports, etc.) or some kind of document(s) that captures the teams assumptions of the target audience.Step 2: Decide on your persona sections and behaviorpromptsNo two personas are created the same. Choosing the sections for your persona that fit your research needs is essential. As Steve Mulder mentions in his book, The User is Always Right, every element of a persona should have a purpose, meaning that it should help a team to better understand and empathize with that user group. Always keep the background relevant to the context of your product or service. Theres no point in including excessive personal background information that does not impact how the persona uses the product or its features.If you want to learn more about deciding what information to include, watch my free 1-hour course, How to Create and Use UX Personas.Step 3: Upload documents to GenAI tool (i.e. yourdataset)Different tools will allow you to upload different file formats. Currently, you can upload PDFs, TXT, JPEG, PNG, DOCX, CSV, and XLS file formats in ChatGPT Plus orTeam.Data privacy: Any file you upload to ChatGPT Plus is retained indefinitely within the service, and those files may also be used by OpenAI to train its models, so its best to refrain from uploading files with any important personal information. If you require a more secure option where chats are not used for training and data is encrypted, youll need to use a ChatGPT Team account or invest in a secure UX research analysis tool like HeyMarvin or Dovetail. I also want to note that there is currently a security issue with ChatGPT Team where its impossible to restrict team members from inviting new members to the workspace, so you must review your workspaces Members page regularly. ChatGPT Enterprise does not have this security weakness. Please check with your IT team before using any GenAI tool with your customer and/or company data to ensure that you adhere to their data security regulations.Step 4: Provide AIpromptYou can adjust the following prompt below to fit the needs of yourpersonaAI PROMPT: [COMPANY] is redesigning their [PRODUCT] to better speak to their target audience. The [PRODUCTS] primary audience is [X]. According to the marketing team, the target audience is defined as [SHORT DEFINITION]. The primary audience uses [PRODUCT] to accomplish [USER GOALS].Write a persona for the [PRIMARY AUDIENCE] based on the definition providedand the attached background materials and research transcripts. The personaneeds to include the following sections: Values, Motivations, Affinities(i.e. brands or organizations that share a similar target audience andcommon interests or values), Goals, Challenges, Needs, Demographics,Behaviors, and Preferred Touchpoints with the Company (online and offline).Step 5: Review and refineresultsIf you find that the first response is not in-depth enough or you would like it to include specific information, you can ask GenAI to refine it in several ways. For personas, I like to provide behavior prompts. For instance, you can use the prompt below to ask GenAI to re-write certain sections of the persona. By the way, these are the same prompts I use with cross-functional teams when facilitating persona workshops to develop proto-personasAI FOLLOW-UP PROMPT: Use the following behavior prompts to re-write the Motivations and Affinities sections for this persona:Motivations1. Personal Goals: a. What are your persona's short-term and long-term personal goals? b. What motivates your persona to achieve these goals?2. Professional Aspirations: a. What are your persona's career aspirations and ambitions? b. What drives your persona to succeed in their professional life?3. Incentives and Rewards: a. What types of incentives or rewards are most appealing to your persona (e.g., financial, recognition, personal growth)? b. How does your persona respond to different forms of motivation (e.g., intrinsic vs. extrinsic)?Affinities1. Lifestyle Choices: a. What lifestyle choices does your persona make that reflect their affinities (e.g., choosing sustainable products, preferring local businesses)? b. How does your persona's lifestyle align with their personal values?2. Preferences and Interests: a. What hobbies or interests does your persona have that indicate their affinities (e.g., hiking, reading, attending cultural events)? b. How do these interests influence their daily life and interactions?3. Social Behavior: a. How does your persona interact with others in social settings? Are they more introverted or extroverted? b. What kind of social activities does your persona enjoy (e.g., group events, one-on-one interactions)?4. Environmental Consciousness: a. How important is environmental sustainability to your persona? What actions do they take to support this value? b. What kind of environmentally friendly products or practices does your persona prefer?5. Brand Loyalty: a. What brands does your persona feel a strong affinity towards and why? b. How do your persona's values and affinities influence their brand preferences?6. Decision-Making Style: a. How does your persona approach decision-making? Are they more analytical or intuitive? b. What factors are most important to your persona when making decisions (e.g., data, recommendations, personal feelings)?This follow-up prompt should result in a more rich result. You could choose to provide the behavior prompts in your initial GenAI prompt, but Ive noticed better results if I spoon-feed the data and do iterative refinement. Putting too many demands in one prompt confuses the model. Patrick Neeman, author of Mastering AI Assistants for User Experience Designers and Product Managers, suggests including user research questions in the prompt to ensure it accurately reflects real users. He suggests adding to your prompt, Generate user research questions that would validate this user persona as correct.Using large language models (LLMs) to segment a userbase into distinct personas based on behavior patterns, preferences, demographic data, etc., is super helpful. However, its important to remember that you can inadvertently perpetuate or even amplify biases if theyre present in the data youre using to trainthem.About accuracy: Remember that all GenAI tools are fallible and can provide wrong answers. Ultimately, you must do your due diligence to ensure the information provided accurately reflects your collected data. If the dataset youre using contains historical biases or imbalances (e.g., in terms of gender, ethnicity, or socioeconomic status), the models outputs will likely reflect thesebiases.TIP: As Bill Bulman mentions in his article, Crafting Personas with AI-Augmented Research (a step-by-step guide), you could also write a prompt to get a day in the life narrative for your persona. Bill provides the example prompt: Provide me a day in the life story for a persona, using the following user interview data, as a baseline.Whether data-informed or assumptions-based, creating UX personas is a nuanced process that significantly benefits from GenAIs support. These tools can assist in crafting the intricate details that make personas relatable and valuable, particularly for those who may not naturally lean towards creative writing. By meticulously gathering and analyzing user data, defining persona elements that genuinely reflect user needs, and strategically using AI to enhance the creative aspects of persona creation, we can develop more accurate and engaging user personas.Journey mapsImage of free journey map template via nulivo.com.You would follow the same steps outlined in creating personas for creating journey maps but with some noted differences to Steps 2 and4.Step 2 would be to decide on your journey map elements. You want to spend a good deal of time defining the stages of your journey and the user data you want to present during each stage (e.g., actions, thoughts, jobs-to-be-done, etc.). I believe GenAI should not handle this heavy lifting. Instead, you will include this in your AI prompt along with your dataset (i.e., the same docs you uploaded above in Step 3, along with your finalized persona).For Step 4 (the AI prompt), I like to use a prompt thatdetails:Who is on the journey (i.e. yourpersona)What journey theyreonThe stages of thejourneyThe information we want to include during each stage (e.g. actions, thoughts, jobs-to-be-done, etc.)AI PROMPT (Generic example):Write a journey map for [x] persona. The map should focus on the journey of [x]. The stages would be: 1) [x] , 2) [x], 3) [x], 4) [x], and 5) [x]For each stage, please provide:1) Jobs to be Done (i.e. Goals - What is the customer trying to achieve?)2) Actions (What does the customer do? What information do they look for? What is their context?)3) Frustrations / Challenges (What does the customer want to achieve or avoid?)4) Online Touchpoints (What online parts of the [x] service do they interact with?)5) Offline Touchpoints (What offline parts of the service do they interact with?)6) Moments of Truth (A customer interaction that can create a positive or negative impression of a brand, product, or service that affects their purchasing decision.)For example, if I were creating a journey map for ordering food through a mobile app, my prompt might looklikeAI PROMPT (Specific example of ordering food using mobile app):Using the provided dataset, which includes a detailed persona, user research reports, survey results, market research reports, and customer service interactions, create a comprehensive user experience journey map for a food delivery mobile app. The persona for this map is 'Chris', a busy software developer who enjoys diverse cuisines. The journey map should incorporate data-driven insights from the dataset at each stage and across various dimensions to accurately represent Chris's experience.Stages:1. Awareness: How Chris discovers the app through ads, social media, or friends.2. Consideration: Chris evaluates the app's features, benefits, and user reviews.3. Signup/Onboarding: The steps Chris takes to download, register, and learn to use the app.4. Exploration: Chris is browsing through various restaurant options and menu items.5. Decision: The moment Chris chooses a restaurant and a meal, including any customization.6. Transaction: Completing the order with payment and delivery details.7. Fulfillment: Monitoring the order's progress and receiving updates until delivery.8. Post-Delivery: Actions post-receipt of the order, including eating, resolving issues, and reviewing.For each stage, please provide:* Actions: Detail each action Chris takes within the app at every stage.* Thoughts: Capture what Chris is thinking as he progresses through each stage.* Emotions: Describe Chris's emotional state at key points across the journey.* Touchpoints: Identify where Chris interacts with the app and any other service element.* Frustrations: Note any specific frustrations or challenges Chris encounters.* Jobs to Be Done (JTBD): Outline the underlying needs or tasks Chris aims to fulfill at each stage.Ensure the journey map is visually structured and differentiates between these aspects, providing a holistic view of the user experience.When reviewing your results, if the journey map does not accurately reflect your dataset, GenAI might be making assumptions!LLMs are very good at predictive analytics and forecasting user actions, and when they take a guess, its not called out in the results unless you specifically instruct them to doso.For this reason, I prefer to refine the prompt to learn where the gaps in the researchareAI REFINED PROMPT (Example to clearly mark assumptions):Create an updated UX journey map for the food delivery app using the provided dataset, which includes user research reports, survey results, and customer feedback. Where the dataset is incomplete or lacks specific information required for a comprehensive understanding of the user journey, you are permitted to make logical assumptions. Clearly labelthese assumptions within the journey map to distinguish them from data-driven insights.Specific Instructions:1. Data Integration: For each stage of the journey map, use direct insights from the dataset. Clearly cite the data sources (e.g., specific survey questions, report pages) that support these insights.2. Identifying Assumptions: Where necessary information is missing from the dataset, make and clearly mark assumptions. Provide a rationale for each assumption based on the context of available data.3. Assumption Labels: Use a distinct visual or textual label (such as 'Assumption' or a special symbol) next to any content in the journey map that is not directly supported by the dataset but is instead an educated guess by the AI.4. Clarify and Rationalize: For each assumption, include a brief explanation of why this assumption is made based on related trends or patterns observed in the dataset.5. Detail and Accuracy: Ensure that each stage of the journey map includes detailed descriptions and visualizations, clearly distinguishing between data-derived insights and assumptions. This should enhance the maps overall clarity and usefulness for decision-making.Outcome Expected: The journey map should seamlessly integrate factual datawith necessary assumptions, providing a comprehensive and practical view ofthe user experience. Each assumption should be clearly marked andjustified, allowing stakeholders to understand and evaluate the basis ofthe insights provided. This will aid in strategic planning and designimprovements, ensuring they are both data-informed and adaptable to areasof uncertainty.TIP: As Nate Jones mentions in his excellent newsletter, Your Pocket Guide to Prompt Engineering: How to Get the Most from AI Models, if you want a response to be in a specific format, you should be sure to specify this in your prompt (e.g. Put the response in atable.)Including opportunities & solutions: For each stage of a journey map, I typically like to provide an additional swim lane for Opportunities (suggestions for improvements based on the identified touchpoints, emotions, and frustrations) and Solutions (proposing design or feature enhancements that could improve the UX based on the insights gathered). To me, this is the sweet spot where the research data in the journey map becomes the most actionable. Ive found that its best NOT to use GenAI to help write these lanes. Typically, the dataset doesnt include the collective teams expansive knowledge to solve the UX problems presented in eachstage.Ive found using AI with these prompts can significantly streamline the creation of journey maps, making the process quicker and more efficient. By leveraging AI, we can more easily integrate the findings from complex datasets into each stage of the user journey, ensuring that every aspect, from actions and thoughts to challenges and interactions, is accurately captured. AIs capability to predict user behavior and generate detailed visualizations can enhance the effectiveness of journey maps. However, it is crucial to manually review and adjust these AI-generated maps to ensure they incorporate human insights and accurately reflect real user experiences to provide a more actionable and comprehensive view of the user journey. While AI can assist in forecasting and visualizing user behavior, the strategic inclusion of human insights remains invaluable in crafting a journey map that truly reflects and improves the userjourney.Moving forward: balancing AI efficiency with humaninsightAs we continue to integrate GenAI into the UX design process, its crucial to find the right balance between leveraging its computational power and maintaining the human touch that is essential for creating authentic and impactful user personas and journey maps. By combining AIs efficiency with our expertise and creativity, we can enhance our workflows to produce deliverables that are profoundly resonant with users actual needs and experiences. I encourage my fellow UX professionals to experiment with these tools, share their experiences, and continue to push the boundaries of what we can achieve together in this exciting intersection of technology anddesign.If youre interested in learning more, consider joining the AI for UX Slack group created by Patrick Neeman. We talk a lot about using AI for UX to better understand how to properly use it to advance our industry. I hope to see youthere!References and furtherreadingNeeman, Patrick. uxGPTMastering AI Assistants for User Experience Designers and Product Managers. Available at GPT PromptGuides.Aguilar, Nelson. Your Guide to Uploading Files to ChatGPT (and Why You Would Want To). Available atCNET.Jones, Nate. Your Pocket Guide to Prompt Engineering: How to Get the Most from AI Models. Available at Nates Newsletter.Bulman, Bill. Crafting Personas with AI-Augmented Research (a step-by-step guide). Available at LinkedIn.Morrison, Dylan. UX and AI: Developing a user persona with ChatGPT. Available atMedium.Harley, Aurora. Personas Make Users Memorable for Product Team Members. Available at Nielsen NormanGroup.Adlin, Tamara. Alignment Personas Framework. Available at AdlinInc.Mulder, Steve. The User is Always Right: A Practical Guide to Creating and Using Personas for the Web. Available atAmazon.Soucy, Kyle. How to Create and Use UX Personas. Available onYouTube.How Im using AI to streamline persona and journey map creation was originally published in UX Collective on Medium, where people are continuing the conversation by highlighting and responding to this story.0 Comments ·0 Shares ·114 Views
-
Designing for AI Engineers: UI patterns you need to knowuxdesign.ccA reference article with guiding principles, patterns, and personas to help designers become more AI literate.As the Head of Design at Modular AI, my team is going from 0 to 1 on a lot of AI products and workflows this year. Even though Ive been designing for developers for years, Ive personally had to quickly ramp up on some AI specific topics and build out our personas, so I thought Id share a few best practices and top references Ive established formyself.Guiding UI principles:Condensed information is welcomed. Much like the B2B industries of data science and fintech, these personas welcome dense dataas well as its designed with a strong hierarchy. So, reduce thosemargins!Parsed metadata is the most clear. Extracting out the metadata and making it search/filter/sortable is important. Tables, side drawers, tagging systemsthese are the main design system elements torefine.Remember Jakobs lawusers spend more time on other sites than your own. There are strong leaders in AI, such as HuggingFace and OpenAI. If theyve established a pattern and theres no strong reason to deviate, go with it. I know, I know, this goes against first principles, but theres a time and a place for first principles. Illl leave it to you to decide that for your team androadmap.A great sources for designing for developers is Githubs design system. Here are a few of their principles that are clearly custom to the developer persona:Its not fully shipped until itsfastAnything added dilutes everything elsePracticality beatspurityApproachable is better thansimpleHalf measures are as bad as nothing atallEncourage flowFavor focus overfeaturesSo with those principles in mind here are some key interfaces that my team has released this year. Keep in mind my team is 4 people (2 designers & 2 developers), and were a fast-paced start-up. Our designs are all released as MVPs at first, then iterated once live, and often built off of existing systems to get from 0 to 1 and get real external validation as fast as possible. All of the designs in this post were created by myself and Will Rust together. Design is a teamsport.The screens Illcover:Product 1: Chat with an LLM (Simple marketing pageversion)As end users, were very familiar with chat interfaces by now. Chatting with an LLM (large language model) as a developer is almost identical to the end user experiences on AI platforms like Chat GPT, Duck Assist, or Perplexity. Its about being as clean as possible and providing fast and conciseanswers.The main difference is that an AI developer is also evaluating the model in terms of its speed, ease of building on top of it, its compatibility, and deploying it.Keep it as clean as a traditional search engine, but offer a subtle 2nd layer of whats happening under the hood, including things like links to the documentation, benchmarking data showcasing the speed of the model, and other data such as cost per token. Here is how we solved for that atModular.UI frameworks we used: https://www.gradio.app/, Mantine,TailwindScreen 1simple start chatting intro screen showing the user which model it is, what hardware its running on, and the traffic volume its handling.And of course everything is light/dark mode compatible. Heres the darkmode version where we embedded the chat inside our developer console.Screen 2 chat results, showing benchmarked data, as well as links to tutorials, the code, and next steps for deployment of thismodel.Left: Expanded benchmark results; Right: Consolidated benchmarksScreen 3The embedded version. For this version, we trained MAX specifically on our documentation using RAG. We started this experiment for internal users only and are hoping to releasesoon.A few choice references:Chatgpt; PerplexityGroq, HuggingFace chatProduct 2: Model RepositoryOne of the most important things that an AI developer needs to do is evaluate GenAI models. This starts with searching through a model repositoryalso called a model garden, model library, or model hub. For marketing/SEO reasons, this is usually a logged out experience, with the main goal being to show users all the models that your platform is compatible with, and encouraging them to log in if they want to test the model more seriously. Our model repo is live at builds.modular.comThe most important features here are essentially search and filter. You want to make sure the user can find what theyre looking for, from a large dataset of what might be hundreds to thousands ofmodels.Screen 2Model detail page. This page tells the user how many variants we have of each model, how to quickly run the model locally, and how to deploy it to a GPU in the cloud if they wantto.There are some fun technical details related to model parameter size, quantization, hardware compatibility, etc. But in the end it all comes down to gestalt clustering and creating the right hierarchy.Designs created by Eve Weinberg (me) and WillRustMy top references here are Huggingface (because theyre the leader in this space), Kaggle (because they have a thriving community), together AI (because theyre Enterprise), and Replicate (because 80k hits per day isalot):Together.aiLike with all successful UI, keep in mind the users mindset here. The AI developer is thinking about models in terms of what task the model can complete, the size of the model, its performance, and who madeit.Product 3: PlaygroundA playground refers to an environment designed for experimentation, testing, and learning without the risks of affecting live systems or production environments. For AI developers, it more specifically will include things like trying out the AI models, testing prompts, and fine-tuning parameters without the need for extensive infrastructure.Were just getting ours up and running now, so Ill just talk about the main features and show some references.Main features:Logged in and Billing connected. Just a small note, but users usually need to connect a credit card because youre officially running AI computenow.Sliders to control the models parameters, change the model entirely.Pay-as-you-go pricingLeft: Vercel; Right:GoogleThis segues perfectly into the whole console experience.Product 4: Developer ConsoleFrom a layout and information architecture perspective, this is like any other desktop console. We use tailwind, mantine, and chartjs to makeours.We got a ittle carried away with bespoke numbering for abitThe fun UI features here are the data monitoring dashboards. If youve ever designed an Enterprise dashboard then the rest honestly is the sameRole based access control, billing, organization hierarchy with members inside that can invite other members, notifications, settings, etc.Product 5: DocumentationOk, lets get into some bread and butterDocumentation! Everyones favorite thing to design;) Heres ours. This was actually my first time ever fully owning the design of a documentation site. We use Docusuarus for ours, as well as Algolia search, which has fantastic UI out of thebox!https://docs.modular.com/maxAs far as the information architecture goes, weve discussed a few approaches here, and narrowed in on two overarching concepts:Follow the users JTBD (jobs to be done)in what order do they accomplish these tasks. For us, its: test locally, serve, deploy to cloud, monitor,scale.Follow the architecture of your product. For us, we have a whole platform that goes from low-level GPU programming to auto-scaling in cloud, so this is also compelling for us to categorize in thisway.Whichever you choose, a landing page that reiterates this is critical.Our most visited page is our Quickstart (not surprisingly!). And were continuously optimizing this page for faster competition, and higher completion rates.Some references:Streamlit Docs (easier when the product surface issmaller)And as I mentioned, Algolia search is fantastic. Heres how it looks forus:Search lightboxedSearch page allows for search across all products andversionsProduct 6: Recipes &CodeRecipes (or cookbooks) is a silly, yet standardized way, of referring to step-by-step instructions. In their most interactive form they are notebooks, where each section is executable code. In their simplest form theyre just a series of instructions with copy/paste-able code snippets.As I mentioned, we build extremely iteratively, so our MVP is truly just rendering a github code example, with metadata called out on the right. I find this this audience, the cleaner and simpler the better. The content itself is so dense, the design shouldnt call attention toitself.A large part of the design system for these articles, is just how you render code, so having an organized system for light and darkmode is a key focus. We use mantine system for this and set our custom colors there, and you can lean on established best practices for CLI color schemes, as shown in this article: https://hamvocke.com/blog/lets-create-a-terminal-color-scheme/Design system for stylingcodeAnd dont forget to have some fun with the CLI, ascii art, and clear progress bars! For UX of the CLI, I just try and teach my engineering teammates the basic 10 NNG principles, and the top one leveraged is visibility of systemstatus.Progress bars in theterminalUsing the ascii art generator tool: https://convertcase.net/ascii-art-generator/Whats next?Im really excited to build so many more workflows and share them. I hope to focus on agentic workflows and cloud monitoring dashboards next.Two low code agentic workflow images from the tooln8n.Bonus imageAI EngineerPersonasAs a reference, here is a genericized version of the personas that I created for Modular. Our bullseye is the Solution Builder fornow.Stay tuned, and thx forreading.EveDesigning for AI Engineers: UI patterns you need to know was originally published in UX Collective on Medium, where people are continuing the conversation by highlighting and responding to this story.0 Comments ·0 Shares ·100 Views
-
Plot twist: How UX design can tell your brands storyuxdesign.ccWhile storytellers construct narrative arcs, UX designers create user journeys.Brands are everywhereour laptops, jackets, even water bottles are allbranded.But brands matter. When you buy a new water bottle, you dont just buy any water bottleyou buy THE water bottle (whatever it means to you). This is where brands come inyou think of its visual identity, product quality, and values. You buy from brands that signify something toyou.According to Blair Brady, a companys brand is its most important asset; no matter the industry orproduct.Your brand isnt just your logo. Its how you interact with the worldfrom your customers to your employeesand it all stems from thebrand.-Brady in Your Brand Is Your GreatestAssetIts not just a brand, but brand stories. A brand may have a defined mission, but it must show it to consumers (or users) at every touchpoint.Yetis drink-ware and cookware productpageBrand stories are told with UX design. Since more users interact with websites and apps to purchase goods, UX is essential.Lets review how UX design is involved in brand storytelling, why you must treat the users journey like a narrative arc, then discuss storytelling tools you have in yourtoolkit.How the heck is UX involved in brand storytelling?Every company has an established brand. Some we know, like Apple and Coca-Cola, while others we cant name off the top of our heads. What do well-known brands have incommon?They use brand storytelling to claim and personify their brandsconnecting with their targetusers.What goes into brand storytelling? How does UX design contribute to bringing it tolife?What is brand storytelling?Brand storytelling represents a brands identity, and is conveyed through every user touchpoint (website interactions, physical stores, etc.) to engage, build trust, and develop lasting relationships.To develop relationships, brands must connect with users at an emotional level.Jeff Rosenblum, a digital marketing expert, recognizes that brands cant only produce great products; they must resonate with users desires through every interaction.Its about connecting with people authentically, filling the gaps in their journey, and providing realvalue.-Rosenblum in 5 Principles Of Telling Great BrandStoriesFor example, think about Patagonias brand. Their mission statement is, Were in business to save our home planet. Their identity is demonstrated throughout touchpointsInstagram posts showing people wearing their apparel and their website offering discounted, used items (WornWear).Examples of Patagonia Instagram postsPatagonia uses narrative elements effectively, which creates a brand personality for users to relate and remember.Before you create a brands narrative, you need to decide on the brands personality. Is the brand environmentally-conscious or is it innovative? Whichever the personality, every user touchpoint supportsit.UX design is the vehicle for storytellingUser experience design carries the brands story by clearly speaking to users. Because UX creates an intuitive digital journey, users are guided through a narrative the brand wants totell.But UX goes beyond aestheticsNielsen Norman Group (NNG) explains that brands are both experienced and expressed by visuals, tones, and behavior.More specifically, UX tells the brands story through visual design, information architecture, and interactions. Lets talk more abouteach.I. VisualdesignFonts, colors, and imagery work cohesively to set the tone for the experience and reinforce the brands personality.Coca-Cola uses a bright red alongside white and black to create an exciting, yet friendly personality. IBM uses a muted blue with white and black to convey professionalism and confidence. These visual elements combine to emotionally appeal and influence user behavior.II. Information architectureInformation architecture (IA) creates the structure and organization of content presented to users. Organized websites help users navigate through digital touchpoints to comprehensively tell the brandsstoryExample structure of a websites information architectureIntuitive user flows make it clear what the brand stands for, what it offers, and why it matters to users. If the IA is cluttered, the brands story gets lostleaving users frustrated (and abandoning thesite).III. InteractionsEvery interaction, like a click or swipe, adds depth to the brands story by creating a dynamic experience.An engaging notification after completing a task (a confirmation snackbar) makes users feel they are interacting with something alive. Interactions give users reassuring feedback and contribute to the overall emotional experience.Treat the user journey like a narrative arcThe user journey functions like a narrativeevery step, interaction, and decision builds towards a satisfying goal. But instead of the storys hero living happily ever after, the user successfully completes atask.This is done by creating a narrative through user flows, embedding emotional design in the UX, and adding personalization.Create a narrative through userflowsJust like storytellers construct a plot, UX designers structure the user flow to be a logical progression. Adobe relates user flows to road-maps to drive users in the right direction; you must understand users, their goals, and what information they need to optimize theUX.Each step in the flow (or road-map directions) leads the user forward in their journey without confusion.But confusion happens, so flows account for both happy and sad paths. You want the user to succeed, but they may encounter errors. Its important to recognize that narrative arcs can take a turn for the worse, and leave users locked up in a dungeon. This way, the UX helps the user make theirescape.The user flow as a narrative arcThe key to crafting the narrative through user flow is seamlessness. Transitions between actions are smooth and intentional, guiding the user between steps and moving them towards their goal (I.E., making a purchase or finding information).Embed emotional design inUXEmotional design focuses on positive, delightful experiences that make users feel something. A story, brand, or design without happiness, suspense, or anger is boring and monotonous.The theory of emotional design has been around for over 2 decades with Don Normans Emotional Design: Why We Love (or Hate) Everyday Things, but didnt begin trending in UX until recently.Everything has a personality: everything sends an emotional signal. Even where this was not the intention of the designer, the people who view the website infer personalities and experience emotions.-Norman, What is Emotional Design(ED)?Warm colors, rounded fonts, and appealing visuals make websites feel welcoming. Bold call-to-actions and intuitive navigation create a sense of confidence. Entertaining animations add layers of emotion to retainusers.Embedding emotions into UX design turns ordinary into a memorable experience to meet functional and emotional needs.Add personalizationTo deepen the narrative arc, personalizing the story makes each user feel like its uniquelytheirs.Personalization tailors the experience based on user data and behavior, like recommending products based on browsing history. Nielsen Norman Group (NNG) encourages personalization to deliver what users need when they need it, but with intentional execution.When a user sees content speaking directly to their preferences, it creates a sense of recognition and strengthens their brand connection.For example, Spotify is well-known for their Spotify Wrapped, which gives users a personalized data compilation of their years activity. A Wrapped tells a user their top-5 artists, what city they have the most in common with based on music tastes, and allows users to share their results on socialmedia.Example of a Spotify Wrapped, released in December2024Rather than passively consuming content, users are given a personalized experience that adapts to themcreating a sense of ownership in their relationship with abrand.Visuals and interactions as storytelling toolsDesigners have many storytelling tools in their kit (fonts, colors, imagery, oh my!) they can use to optimize a brands storylets look ateach.Set the tone through fonts andcolorsFonts communicate a brands character before a single word is read. A sans-serif font (Helvetica) makes the brand feel modern, while a serif font (Merriweather) suggests the brand is more traditional.Colors influence users mood and perception on a subconscious level. A brands color palette ensures visual harmony and reinforces the brand. In Heres why all fast-food signs are red, its suggested that fast-food brands use red not because it make us hungry, but because its a stimulating and exciting color. In contrast, big-tech companies choose calming blues to project a sense of reliability and security, shownbelow.Contrast of colors and perceived feeling between IBM and McDonaldsFonts and colors act as the brands conversation starter to users, and users decide to continue the conversation or leave simply from these visuals (its that powerful). Fonts and colors shape the users associations the moment they encounter a design, which makes or breaks their brand connection.Choose imagery to speakvolumesImagery can be implemented with photographs, illustrations, or custom icons; whichever one selected, the visuals communicate the brandsmessage.Tech startups opt for futuristic imagery and minimal iconography to emphasize characteristics like innovation. Wellness brands use organic imagery and rounded icons to convey tranquility. Regardless of style, the imagery must be consistent and aligned with the brandsvoice.When imagery is thoughtfully chosen and consistently applied, they illustrate the brands message and emotionally connect withusers.Bring the story to life with animationsMicrointeractions, like a button changing color when hovered over, strengthen the storytelling experience by making it feel interactive. They create small moments, but boost user engagement and tell more about the brand. For example, a cheerful, bouncy button on a childrens site reflects the brands lightheartedness, while a polished transition on a luxury brands site conveys sophistication.Animations establish the pacing of an experience. They guide the user through content in a controlled, deliberate way. Val Head, an animation expert, says animation captures and directs the users attention to the most important content through hierarchy.Of all the available design tools at our disposalsuch as color, type, and layoutanimation is the one that talks with its outsidevoice.-Head in Six Principles of Using Animation in UXDesignThe style of animations and microinteractions support the brands core values. Sustainable brands (Kowtow) use slow, organic movements in its website elements to convey calmness. Tech startups (Mailchimp) use jumpy, precise animations to emphasize efficiency.Brand stories are not just about marketing campaigns, and UX design is not just about creating pretty interfaces. They are combined forces which shape how users experience and connect with abrand.From the deliberate use of fonts, colors, and imagery to smooth navigation and dynamic interactions, UX design tells the brands story. By integrating emotional design and personalizing the user journey, brands can engage users and develop lasting relationships.Plot twist: How UX design can tell your brands story was originally published in UX Collective on Medium, where people are continuing the conversation by highlighting and responding to this story.0 Comments ·0 Shares ·94 Views
-
Simplest, dumbest reason your meetings suckuxdesign.ccThis is one you can fix all by yourselfno need to schedule more meetings to make bad meetings more tolerable.Continue reading on UX Collective0 Comments ·0 Shares ·116 Views
-
The design game has changed and I dont know where I fit anymoreuxdesign.ccAfter two decades in designleading teams, managing million-dollar portfolios, and launching products used by millionsIm now questioning myplace.Reflecting on my journey, Ive had the privilege of navigating the dynamic world of design for over two decades. From leading a 10-person team at my own agency to scaling You.i TV from 22 to more than 210 employees, I grew the design team from 2 to 21 while collaborating with powerhouse brands like the NFL, Fox, Turner Television, and Cartoon Network. This journey ultimately led to a $100 million acquisition by Warner Media. Along the way, Ive also deepened my expertise in mid-sized companies across B2B, B2C, crypto, and AI sectors, while venturing into the complex world of enterprise software.Yet, despite these accomplishments, I find myself at a crossroads, questioning my place in an industry that has evolved dramatically.This introspection surely isnt unique tome.Many mid-career professionals grapple with similar feelings of uncertainty and reevaluation. The challenge lies in aligning ones passion and expertise with the industrys current demands, all while seeking personal fulfillment andbalance.Original design for a Podcast Framer Team template I am workingon.Is this aproblem?Perhaps its more of an opportunitya chance to redefine success, mentor emerging talent, and leverage our rich experiences in new, innovative ways. The design landscape may have changed, but the core principles of creativity, problem-solving, and human-centric thinking remain as vital asever.I still love design. The craft. The details. The elegance of a well-thought-out experience. The feeling of bringing something into the world that makes life just a little bitbetter.But lately, I feel like Im standing at a crossroads.Im in my 40s (okay, Im 48), and my ambitions have shifted over time. Trying to find my voice and build a personal brand as a designer on , Linkedin and other platforms feels a lot like a teenage identity crisis. The relentless drive I had when I started in this fieldthe late nights, the hustle, the constant push for the next big thingdoesnt fuel me the same wayanymore.Ive been online for many years, maybe it should have been different, but currently, it feels like Im trying to talk to my 16-year-old self Im continuously questioning myself onhereShould I even? What should I do Should I keep going Am I trying to be someone else? Mid-lifecrisis?Personal wallpaper designEvolving throughtimeIm no longer chasing titles, prestige, or the illusion of success defined by external validation. Im chasing balance and projects I truly want to workon.I want to create work that matters, but not at the expense of my health, my relationships, or my ability to simply enjoy life. I want deep, meaningful impactnot just metrics andoptics.I still love designthe craft, the problem-solving, and the beauty of creating something meaningful. But the game has changed. And Im left wondering whats next for the industry andmyself.AI, the creator economy, fragmentized designers, freelancing, smaller agencies, and so on. The world is evolving so damnfast.Technology has advanced more in the last thirty years than in the previous two thousandNielsBohrDesign isnt just about designing anymore. Its about playing the online game, building an audience, and becoming a content machine. It feels less about the work and more about marketing yourself.The creator economy is no longer a trendits the new reality. The creator economy is currently valued at approximately $104.2 billion and is projected to double by 2027. Globally, it encompasses a staggering 207 million content creators.Leaders like Justin Welsh, Dan Koe, Naval, Alex Hermozi, Chris Do (and many others) have mastered the game, building massive audiences, establishing undeniable credibility, and turning their ideas into highly profitable courses. Theyve proven that personal branding isnt just a buzzwordits a business model. But now, as more people chase the same formula for success, the space is becoming an echo chamber. Everyone is optimizing, iterating, and repackaging the same messages, and originality is starting tofade.I see younger designers putting in 20-hour days, grinding, iterating, chasing trends. They create, post, optimize, and repeatrelentlessly. And I wonderdo I still belong here? Can I still compete? Or is there a different game I should beplaying?I got into design because I loved solving problems, making things better, and creating experiences that mattered. Now, I feel like I have to sell myself before I even get to the work. And thats exhausting for me. Im probably not the onlyone.This might make me sound like my grandparents reminiscing about the good old daysyou know, the classic When I was young, we walked five miles to school in 12 feet of snow speech. But the truth is, things really havechanged.I grew up before the internet was in every pocket. Before social media dictated careers. Before design became a game of algorithms, engagement metrics, and personal branding. I learned design when craftsmanship mattered more than how well you could market yourself.Back then, if you were good at what you did, the work spoke for itself. Now, it often feels like the work is secondary to how well you package and sell it. You can be an incredible designer, but if youre not posting daily, optimizing for reach, and playing the social media game, youre invisible. Marketing is a new tool every designer needs tomaster.And that makes me wonder: Is this evolution? Is it a distraction? Is it even for me? Is this what I trulywant?As you can see, Im questioning a lot right now. Not just about design, but about whats next for me. Do I double down and adapt? Or do I carve out a new path that feels more aligned with what I trulywant?My biggest challenge has been overcoming my digital shyness. Does this reflect 20 years of creative experience? floods my head every time I post something. Even if I know Ive created products used by millions, I often feel like an impostor syndrome when someone posts a fancy button design which gets millions ofviews.Picture of myself frombehindAdapt, evolve ordie.Navigating a design career over two decades brings both profound insights and evolving challenges. As I reflect on my own personal journey, I recognize the shifting landscape of our industry and the need to adapt while staying true to ones corevalues.Michael F. Buckley explains it really well. Having the ability to shift your perspective allows us to realign our thinking. For me, it feels like Im struggling to see the bigger picture. The rapid rise of new design technologies and specialties in AI advancement is clouding my judgment and shaking my confidence.But, like with everything, sometimes all you need is to step back, take a breath, and regain perspective before movingforward.Embracing change and continuous learningThe design field is in constant flux, with new tools, methodologies, and trends emerging regularly. To remain relevant, its essential to adopt a mindset of lifelong learning. This doesnt mean chasing every new trend but rather discerning which advancements align with your design philosophy and can enhance yourwork.MentorshipSharing wisdom and gaining newinsightsOne of the most fulfilling aspects of a long-standing career is the opportunity to mentor emerging designers. Sharing experiences not only aids in their development but also offers fresh viewpoints that can reignite your passion. Mentorship is a two-way street; while you provide guidance, you also gain insights into new design paradigms and cultural shifts. Organizations like ADPList connect designers globally for mentorship opportunities.Redefining successAligning career with personalvaluesWith experience comes clarity about what truly matters. Its crucial to periodically assess your career goals and ensure they align with your personal values. This might mean prioritizing projects that offer meaningful impact over those that merely add to your portfolio. An article on UX Collective delves into building a design career that resonates with ones corebeliefs.Building an authentic personalbrandIn todays digital age, establishing a personal brand is vital. However, authenticity should be at its core. Share your journey, including both triumphs and challenges. This transparency not only humanizes you but also builds trust within the design community.Prioritizing well-beingThe foundation of sustainable creativityThe drive to excel can sometimes lead to burnout. Its imperative to establish boundaries, practice self-care, and seek support when needed. Remember, your well-being is the bedrock of sustained creativity and innovation.Picture Picture of me and my family on a vacation to Xochomilco, MexicoWrapping this open-hearted conversationMaybe youve felt this toothe pull to evolve, but not knowing exactly where to go next. The sense that the industry is shifting, and youre trying to figure out where you fit in all ofit.While the design industry continues to evolve, anchoring oneself in continuous learning, authentic connections, and personal well-being can provide a compass to navigate the journey. Embracing these principles ensures that we not only stay relevant but also find fulfillment in the work wecreate.I dont have the answers yet. But I know Im not alone in this. If youre feeling the same way, lets talk. Lets figure it out together.2025's Creator Economy Statistics That Will Blow You Away40 Creator Economy Statistics You Need To Know in 2025Where Is The Creator Economy Headed In 2025?growing old in the design industry at DuckDuckGoIm currently a Design Principal & Creative Director at SAP, a spiritual and a health enthusiast. I write about design, skills, careers, and systems. Im all ears for your take on anything design or, feel free to connect with me on Linkedin orX.The design game has changed and I dont know where I fit anymore was originally published in UX Collective on Medium, where people are continuing the conversation by highlighting and responding to this story.0 Comments ·0 Shares ·111 Views
-
Duo run over by a Cybertruck?!uxdesign.ccDuolingos mascot is apparently dead. Long live Duo.Continue reading on UX Collective0 Comments ·0 Shares ·110 Views
-
Putting the ink into design thinkinguxdesign.ccLets start with a thought experiment. Im going to give you a single word and lets see what comes to your mind. Dont overthink it. Its not a trick. There are no prizes. No winners or losers. Just let your mind conjure up a picture in response to this word...MAGNUMHeres one I preparedearlier.Having run this activity in workshops and training courses over the years, Im confident therell be some mental images of chocolate-smothered ice cream, a few Clint Eastwoods in Dirty Harry, maybe the legendary photo agency, a Tom Selleck or two, or an oversized bottle of champagne. There are lots of ways to interpret and visualise this singleword.Its a good demonstration of what happens in most meetings. Everyone nods and agrees and leaves happy, thinking their Magnum is the one everyone sees and agrees with. Disappointment and disagreement are postponed for anotherday.Somewhat perversely, many people host meetings so they dont have to make a firm decision. They encourage people to talk in the abstract without anyone having tocommit.As designers, our skill is in taking the abstract and making it real. Its good to create something everyone can look at and have a shared conversation around. Being able to go from 0 to 1 at speed is a form of alchemy (and we can repeat this trick when what we show needs changing).Exploring ideas by making amark.We turn our thinking into sketches, scamps, wireframes, prototypes, and high-fidelity designs. We lower risk, save money and speed up project delivery by communicating ideas that facilitate more meaningful discussions. As a designer, dont go to a meeting without one or two visuals to focus the conversation around.Be selective. Not everything in every fidelity.In design projects, Ive noticed a tendency for folk to fall into the trap of stepping through the fidelity of the designs in a linear fashion. Some rounds of 6-ups then become sketches for pages before being tidied up into wireframes and passed into Figma. But you dont always need every step for everypage.A colleague of mine has a phrase I really like: Were designing websites for users, not pictures of websites for users. Its a useful reminder that the digital design process has an end goal, and our efforts should be targeted at getting useful solutions into the hands of the user as soon as possible. In the wise words of designer Brenda Laurel: Design isnt finished until someones usingit.The following three questions are helpful when working out what to create and whats the right fidelity at the righttime.1. Whats the quickest, cheapest thing I can create to help make the next design decision?Your visual output should be steered by the problem youre trying to solve and the people you need input from. The work you create should help you explore the challenge at hand. At the early stages of a project, I generally find exploring concepts more important than the execution of the artefact. Even more so when I can show and talk through theideas.Im always looking to do less where I can. The time I save can then be invested where itll have the most impact or where the design challenge is the trickiest.I sketch on paper when I need to think freely, flesh out unusual ideas, or generate a wide range of possibilities. When I need to add finesse, polish and personality, I tend to work in high fidelity. But not always. If a developer can work from a sketch on a napkin or an ordered pile of post-it notes then this is as far as Ill take my designwork.Low fidelity doesnt mean shallow thinking. In fact, the opposite. The best way to arrive at the concepts, metaphors, and models that will drive any design work is to do it early in a project and through many iterations and criticalreviews.Talking and drawing around a whiteboard.Youre answering different questions with different mediums. In one complex project working with a travel company, a whiteboard was the perfect canvas to start investigating ideas. Working in a team in a shared space helped unblock inertia. The whiteboard forced conversation and collaboration, and whiteboard pens excel at preventing any chance of drawing in finedetail.Most importantly, in this project, the next design decisions were made by people in the room who could congregate around the whiteboard.2. What can I create to best demonstrate the essence of theconcept?Its easy for interesting ideas to get lost in the backlog or to be considered only at the end of a project if theres any time left. Creating micro demos throughout a project helps avoidthis.These small proofs of concept dont have to be digital. Its amazing what you can mock up with paper, pens and basic craft equipment. You dont have to wait until youve figured out the entire end-to-end journey. Better to create demos for parts of the product as you goalong.As designers, we can over-fixate on the precision of execution to the detriment of the thinking behind the design. With a demo for a concept, its useful to remind yourself youre not showing the proposed design; youre showing your ideas that may (or may not) edge toward being the delivered design.Performance, accessibility, browser compatibility, alignment with brand guidelines, considered typography, and beautiful touches of design delight are all important, but not yet. At this stage, its perfectly okay for your demo to be bootstrapped together with poor code and held together with sticky tape. Test out your ideas with something tactile and throwaway while you have the time and space in aproject.A micro demo to show early ideas for a complex interaction pattern.I worked on a project with an intricate checkout flow with layers of conditional logic. Designing small discreet demos to pilot novel interaction ideas helped evaluate and develop our potential solutions. In this case, getting a feel for how something behaved in a browser was the fastest way to try and share new ideas without becoming too attached and committed to any ofthem.3. How can I most effectively share the thinking behind the design with decision-makers?Until you get into the final phases of delivery, the thinking behind the design is more important than how it looks. Early design sketches are ways of expressing your thinking. The success of your sketches is in helping whoevers looking at them more easily imagine the finalproduct.In communicating the thinking behind the designs, Ill take time to identify who needs to make what decisions and what will help them to doso.In most projects, you use a variety of channels and formats to share your work in progress. The trick is to use the best channel for the decisions you want made and the situation of the decision-makers. To do this, I start by answering some questions:Who is the decision-maker, and what do they need to make a confident decision?Is the conversation going to be synchronous or asynchronous? If theres a choice, whats my preference?Whats the perceived size and implication of the decision beingmade?What type of feedback am I looking for? A firm agreement or a nod to indicate that Im moving in the right direction?How familiar are people with the challenge, and how much context is required?Your job is to make it easy for the decision-makers to arrive at a timely, informed, and confident conclusion on how to progress.Using Loom to talk through some early designideas.Im a big fan of using screen recordings to show work early to get feedback on ideas. Theyre quick to produce, easy to distribute, and for the viewer, they are convenient to watch when they have the time and space to doso.Showing the work and simultaneously sharing the thinking behind it is a potent combination. This approach is especially useful when the design ideas are more radical and when decision-makers benefit from having more time to reflect on and consider the proposed approaches.Ive found that keeping videos short and focused on a single design decision is most effective. The recordings dont need to be Hollywood blockbusters. However, like all good stories, they need to have a beginning, middle, and end. Avoid a tour through the interface. Instead, highlight the problem youre trying to solve and explain why and how your proposed ideas provide an interesting and impactful solution.Putting the ink into designthinkingDesign can make the abstract concrete. It allows us to play around with and stress-test new ideas. Design enables us to explore possible futures and then bring them tolife.In articulating ideas, look to create just enough to express an idea, to spark a better conversation, and to rapidly investigate alternative (and hopefully radical)options.I like to think about my work as being a step on the road to confident decision-making. Most of my work is a set of disposables rather than deliverables, and I celebrate this. The value is in the depth of thinking and clarity of communication that comes from creating visuals to share. Thats more important than the quality of the marks on thepage.This article was originally published on the Clearleft website: https://clearleft.com/thinking/putting-the-ink-into-design-thinkingPutting the ink into design thinking was originally published in UX Collective on Medium, where people are continuing the conversation by highlighting and responding to this story.0 Comments ·0 Shares ·90 Views
-
Your brain has been tricked, and its loving ituxdesign.ccIs Design a game of subtle manipulation and beautiful lies? Do designers not just make things work but also make you believe?Continue reading on UX Collective0 Comments ·0 Shares ·96 Views
-
Are Duolingos AI video calls just a gimmick or a valid way to study?uxdesign.ccTurning the traditionally boring world of language learning upside down.Continue reading on UX Collective0 Comments ·0 Shares ·83 Views
-
Fail early: the hidden design principle behind great UXuxdesign.ccWhy good designs fail as early as possible in the userjourney.Theres a principle in design that doesnt have a nameat least, as far as I knowbut can make or break products and services due to the intense frustration and rage elicited from users when the principle is violated. Ive taken to calling it failing early because I keep seeing it pop up everywhere.Just to clarify, Im not referring to the fail fast principle in startup culture in which its preferable to spend minimal resources to test an idea, but its a tangentially relatedconcept.At first glance, failing early doesnt sound like a good thing. Why would I want my service to fail at all? you might be thinking.But hear meout.Why is it important to failearly?Whether your product is software, or a retail operation, or any other service, you cant fulfill all customer requests all of the time. You might have geographic constraints that limit your service coverage to certain areas, disruptions to your supply chain that make certain products unavailable, or you might simply get requests that are outside of your core competency.In those situations, its better to let the customer know at the earliest possible time that they cant get what they want. Thats failing early in a nutshell.In a way, failing early is an extension of the UX heuristic visibility of system status, which advises us to present feedback to the user as quickly as possible in order to create trust through predictable interactions.Failing early vs. failinglateConsider the scenario when an experience fails late. Lets say you go to a restaurant. You leaf through the menu, take a few minutes to decide, and finally call the server. The server takes your order. After 15 minutes of waiting, he informs you that the salted fish fried rice is unavailable and asks if you want to have it replaced. You reluctantly agree. After all, youve already spent 15 minutes waitinga sunk cost fallacy, but lets leave that discussion for another time. After another 20 minutes of waiting, the iced tea and beef brisket arrives but the server informs you that the dumplings you wanted are unavailable. By then your frustration is reaching volcanic levels but its too late to back out now. You eat the food you didnt want in the first place, make a payment, and leavevowing to leave a badreview.Compare that to the alternative of failing early. You arrive at the restaurant and take your seat. As soon as you sit down, the server immediately informs you that dumplings and salted fish fried rice are unavailable. You decide to go elsewhere.Flow chart illustrating the fail late vs fail early restaurant scenario we discussedFailing late creates so much frustration and annoyance because it wastes your time and locks you into suboptimal options. Conversely, failing early helps you avoid wasting time and resources and maximizes your autonomy.Applying the fail early principle to a food deliveryappSo how do we apply the Fail Early principle?First, you need to identify your failure scenarios. For a food delivery app, this might include the following:The restaurant isclosedThe delivery address is outside of the serviceareaA specific food item is unavailableExpected delivery delays due to a lack ofridersThen, you need to design your customer journeys and flows so that the failure scenarios can surface at the earliest possible time. This is why its common for delivery apps to ask for your location at the beginning of the journey so that it can immediately filter out all restaurants that dont include your address in their servicearea.Screenshot of the Puregold grocery delivery app which asks for the location so it can filter out grocery items relevant for a givenbranchClosed restaurants can be handled by either hiding them from the search results or showing them from the search results but placing a label and applying visual styling to indicate that theyreclosed.Unavailable food items can be handled similarly by applying visual styling and clear labels to indicate theyre out ofstock.Screenshot of Grab Food delivery clearly communicating unavailable itemsFailing early in digital banking and mobilewalletsUsing the method we discussed earlier, lets first identify a key failure scenario for digital banks: third-party downtime. Mobile wallets and digital banking apps typically integrate with a swathe of other third parties to provide services such as paying bills, adding money to your account, sending money across banks, and so on. Inevitably, these other parties will experience downtime.Now, as weve talked about, we need to push the failure as early as possible in the user journey to avoid frustration.For example, if theres a bank transfer facility and the third-party is down, then we shouldnt let the customer proceed with the transaction at all so that they dont waste time typing the recipients account number and amount, especially if they havent saved those details previously.Screenshot of mobile wallet showing that one of the banks, BDO, is unavailableHow do you actually failearly?We now know how to apply the principle on a high level. Theres still one remaining question, though: how do we actually inform the customer in a digital app or service? Should we show an error? Hide the problematic service or product? Disable theitem?As usual, it depends on the context. The most important thing is clearly communicating why they cant proceed with something.For out of stock products, you could place an Out of stock or Unavailable badge along with subtly greying out some of the colors, though keep in mind your contrast ratios for accessibility.Screenshot of e-commerce app with Sold Out badge on A3 paper item, Out of Stock indicated under Quantity, and button label changed to Add to Wishlist instead of Add toCartFor unavailable services like our bills facility on downtime earlier, we can apply a similar badge along with slightly greying out the icon. Then, show an error message when they tap on theicon.What you SHOULDNT do is just disable buttons or actions without any explanation via copy or error message. Disable buttons is generally bad practice because the user often doesnt know why its disabled.What if you hid the unavailable item instead? Well, hiding has a similar issue: the user doesnt know what happened and why they suddenly cant find a feature or product they wanted. Usually, its only a good idea to hide something in the UI if its irrelevant to the current mode or situation. There are some exceptions.In our example of a food delivery app earlier, you could initially hide the restaurants that are closed, i.e. outside business hours, but provide a filter to show closed restaurants so that customers can order for the next day. Or, you could sort the search results so that the closed restaurants show up at the bottom. That way, the results are more relevant but the customer can still find the closed restaurants.Key takeawaysFailing early means informing customers as soon as possible when you cannot meet their needs, which helps avoid frustration and wastedtimeIdentify potential failure scenarios and find ways to surface them to the user as early aspossibleClearly communicate why the product, service, or function is unavailableFail early: the hidden design principle behind great UX was originally published in UX Collective on Medium, where people are continuing the conversation by highlighting and responding to this story.0 Comments ·0 Shares ·109 Views
-
When AI takes the drivers seatuxdesign.ccOpenAI Operator and the shift to AI-first interfaces.Made with MidjourneyHow do we create user-centered experiences when our users arenthuman?Watching OpenAIs Operator navigate the web, I cant help but think about the early days of my career in the user testing lab at AmericanExpress.As a design engineer, I sat quietly watching customers use our mobile banking prototypes. Each interaction told a story: the smile of delight when something worked intuitively, the furrowed brow of confusion when it didnt, the forced politeness of someone clearly just telling us what they thought we wanted to hear. It was fascinatingand crucialto see our work through humaneyes.That kind of scene has defined software development for decades. But with AI agents like Operator, this human-centric approach to design is starting to feel almost quaint. Were entering an era where much of the software we create wont be used by humans at all, but by AI. This shift fundamentally changes how we think about interfaces and challenges our core assumptions about human-computer interaction.Source: OpenAIDemoAI is changing from copilot todriverAt first glance, Operators interface appears simple: a chat UI with an embedded browser. But this simplicity hides a seismic shift in software interaction.The core tension lies in the interface itself: humans need a visual interface to take action and monitor the AI, but the agent would be perfectly content with just some plain text or an API. As agents take the lead in software interaction, what becomes of our carefully crafted visual interfaces? How do we balance agent-first interaction while allowing humans to effectively takecommand?This evolution mirrors what weve seen with autonomous vehicles, where Tesla and Waymo have maintained controls like steering wheels and pedals while gradually increasing vehicles autonomy. But while physical vehicles face real-world complexity that slows their transition to full self-driving, software agents operate in more controlled digital environments. Here, the transition from providing assistance to taking the lead could happen far morerapidly.Building trust through collaboration and visualfeedbackEven if these agents were ready for full autonomy today (which they arent), users arent ready to grant it. While theres excitement about Operators potential, it will take time for people to adjust. Theres no foundation of understanding andtrust.This need for trust-building is evident in the interface design, which prioritizes visibility into the agents actions. This visual feedback loop is crucial for building user confidence, especially compared to prior voice-only interfaces like the Rabbit R1, where course-correcting an agents actions proved much more challenging.The user can take control from the agent. Source: OpenAIDemoCreating a sandbox for agents to play andlearnThe technical decisions behind Operators release reflect an emphasis on safety with careful consideration of capability andcontrol.OpenAI opted for a remote browsing approach instead of enabling computer use directly on users local machines. While this rules out some capabilities, it creates a sandboxed environment where the agent can operate while maintaining security. It also enables the benefit of running many workflows in parallel by distributing tasks across browsers in thecloud.This is how a new workforce emergesAs renowned AI researcher Andrej Karpathy noted on Operators launch day: I think 20252035 is the decade of agents youll spin up organizations of Operators for long-running tasks of your choice (eg running a whole company).Despite the initial demo, Operator is not a basic tool for ordering groceriesits the prototype of a tool capable of running entire businesses.Even in its limited release, Operator represents a key milestone in OpenAIs quest to unlock AI as an active participant in the digital ecosystem. By launching within the $200/month Pro tier, the company can gather valuable training data from power users while limiting initial exposure, then gradually expand the tools footprint as it improves.The strategy is clear: start small, gather data, improve capabilities, expand access, and apply to as many workflows as possible.Rethinking what the futureholdsFor those of us whove spent our careers laser-focused on human users, its time to expand our thinking. Just as I once sat watching humans navigate banking software, I now find myself studying AI agents navigating the web. The fundamental questions remain similar: How do we ensure reliable, efficient interaction? How do we enable appropriate control? How do we buildtrust?The next generation of software will need to serve both human and AI users, often simultaneously. Success wont just be about technical capabilityit will be about finding the right balance between automation and oversight, between AI capability and human control. And perhaps most crucially, it will be about designing experiences that make this collaboration feel even better than prior software interfaces weve come to know andlove.If you enjoyed this post, subscribe to my newsletter or follow me on social media: X, LinkedIn, Bluesky.When AI takes the drivers seat was originally published in UX Collective on Medium, where people are continuing the conversation by highlighting and responding to this story.0 Comments ·0 Shares ·105 Views
-
How to design for troubleshooting when the user workflow isnt clearuxdesign.ccHow do you design when theres no set path users follow?Continue reading on UX Collective0 Comments ·0 Shares ·93 Views
-
Why AI (desperately) needs designersuxdesign.ccArtificial Intelligence, despite its benefits, presents designers with unprecedented challenges that demand ouraction.The responsibility of us designers to protect user interest and value has never been so important. Not only were living in a trendy era with the rise of ChatGPT and many other AI tools, but were also experiencing how these systems shifts control away from the user. Whether its automating tasks, discovering hidden patterns, enhancing user experiences, generating new content, or optimising decisions, AIs existence is driven by its ability to learn from data and improve over time without explicit programming. Currently, the speed and the way these super powerful technologies are being rolled out, we cannot follow as society, which causes great impact on the economy, on mental health, on culture and on society aswhole.We, designers, have to own up to our role in this emerging predictive world because we have our invisible hand meddling with the controls Helen ArmstrongThis quote is from the wonderful book Big Data, Big Design: Why Designers Should Care About Artificial Intelligence authored by Helen Armstrong, which explores the intersection of design and artificial intelligence, emphasising the role of designers in shaping AI-driven experiences. In a predictive digital world, we play a crucial role in ensuring that AI solutions are ethical, transparent, and aligned with human values. We must consider the potential biases in data, the impact on user privacy, and the societal implications of our creations. By prioritising responsibility and accountability, designers can help build a future where AI technologies enhance human capabilities and improve quality oflife.The book explores these issues in depth, featuring contributions from various designers, educators, and researchers who work at the intersection of artificial intelligence and design. It serves as my primary reference for the questions and discussions raised in this article, but I have drawn from numerous other sources to provide a comprehensive perspective, all of which are listed at the end of thisarticle.https://helenarmstrong.info/big-data-big-design/The central theme of the bookand of this articleis to emphasize the responsibility of designers in the ethical development of AI products and services. Our discipline is fundamentally human-centric, focusing on understanding and addressing human needs and desires within the broader societal context. The psychology, research, and strategy we employ in designing our products have a profound impact on the physical, mental, and emotional well-being of people worldwide.AI is human-centric?Human-centric design, like inclusive and participatory approaches, ensures fairness in data models, mitigates bias, and promotes inclusivity. It is widely recognised that human-centric design has been the guiding principle of all design frameworks and processes over the past decade, and this should be no different when working with AI. These perspectives have been valuable because they inform decision-makers about potential human impacts and help anticipate unintended consequences. Initiatives, such as Stanford Universitys Human-Centered AI Institute and MITs substantial investment in AI education, exemplify the global effort to prioritise a human-centric approach. According to this article by Sarah Tan, by exploring AIs human impact through various Human-Centered Design (HCD) approaches, design aligns values between humans and machines, integrating ethics at the projects core.Putting humans at the center of AI systemsHuman-Centered Artificial Intelligence: Three Fresh Ideas. Shneiderman B.(2020)Advocating for user needs, design research has the power to examine AI implications in real-world contextsaddressing socio-economic dynamics too. However, this isnt easy. We, as designers working in the industry, often feel pressured to quickly adopt the latest trends without time for reflection. We are frequently required to use behavioural research to influence user choices, sometimes at the expense of their well-being. Additionally, we must operate in fast-paced environments, adopting to the move fast and break things mentality popularised by early Facebook culture. This approach can make it harder for us to design thoughtfully and ethicallyBut how to stand up for people in the face of suchforces?AI capabilities all designers shouldknowHeres what I aim to achieve with this article: to inspire designers to get more involved in creating AI systems, so we can make the process more ethical and human-centered. This means using design thinking as our guide to reduce the negative impacts of AI on society by developing human-centered AI systems and guidelines.How we can achieve this is still being figured out, and we are all part of this process! To help, I want to highlight the main capabilities discussed in Armstrongs book, where we designers should be actively involved. We need to advocate for design decisions that meet user needs and respect the socio-economic context in an ethical way. It wont be easy, but we can start paving the way with these elements.1. Designing to power up predictionsPrediction is a central capability of machine learning (a subset of AI that involves training algorithms on data to make predictions or decisions without being explicitly programmed). In the context of predictive modelling, the goal of machine learning is to forecast future events or outcomes and anticipate behaviours based on patterns found in historical data.For instance, we can think of Gmails feature that autocompletes sentences based on your past behaviour. Over time, the system learns an individuals style so well that it can finish sentences and even suggest appropriate tones or sentiments.Screen capture from my personalGmailOr we can think of the numerous applications across various industries, such as stock price forecasting, inventory and supply chain optimisation, healthcare predictions (such as disease outbreak forecasting), targeted marketing campaigns, and other personalised user experiences.While these AI-driven predictions arent always 100% accurate, they offer significant benefits to businesses. The advantage is that by anticipating future demands, trends, and needs, companies can better prepare for opportunities and gain a competitive edge. For that end, designers are required to plan for a shifting experiential landscape when working with intelligent technology in their projects.On the other hand, designers cannot blindly apply AI technology without the risk of subjecting humans to discrimination, surveillance, and/or manipulation, not just individually but at scale. Predictive models can sometimes reinforce existing biases or lead to unintended consequences.Face Cages are a dramatization of the abstract violence of the biometric diagram. More on: https://zachblas.info/works/face-cages/Designers have a responsibility to foresee these potential issues and take steps to mitigate them, ensuring that their designs are ethical and inclusive. As Kate Crawford, AI Now Institute founder, putsis:Understanding the politics within AI systems matters more than ever, as they are quickly moving into the architecture of social institutions: deciding whom to interview for a job, which students are playing attention in class, which suspects to arrest, and muchelse.2. Designing to anticipate future scenariosIn her book, Helen Armstrong explores the concept of anticipation, showing how designers can use AI to predict and meet user needs proactively. This involves leveraging data-driven insights to stay one step ahead of users, reducing friction and enhancing the overall user experience.One advantage of anticipatory design is that it reduces decision fatigue by minimizing the cognitive load on users through predictive systems. These systems offer relevant choices or take actions on behalf of users. For example, companies like Netflix and Amazon use predictive analytics to recommend products or content. However, there are ethical implications, as such systems might infringe on user autonomy or privacy. As Armstrong rightfully states on page210:The promise of anticipatory design lies in its potential to craft experiences that feel intuitive and personalised. Yet, with this power comes the duty to wield it responsibly, ensuring that the human element remains at the heart of every design decision.Fortunately, there is a forward-thinking approach that allows us to create products and services that are resilient to change and can evolve with user expectations. Designers can use AI to simulate and model potential future scenarios, anticipating various user needs, environmental factors, and societal changes. This ensures that our designs remain relevant and adaptable.Flock Defines Flight Risk to Make Insurance for Drone Operations Simple: the insurance company worked with studio IF to create an interface that help clients understand how automated decisions were made around insurance rates.3. Designing to create personal experiencesPersonalisation in user experience design involves tailoring interfaces, content, and interactions to meet the specific needs, preferences, and contexts of individual users. When we talk about personalisation in the context of AI, it often means leveraging predictive technologies and data analysis to deliver experiences that are more relevant and valuable to users at any given moment. Personalisation ensures that the information or actions presented to the user are useful, usable, and desirable, inspired by the popular quote from design researcher LizSanders.AI can enhance personalisation by analysing vast amounts of user data to identify patterns and preferences, using predictive models to anticipate what a user might need or want nextthis can involve considering the users current context, such as location or time of day, to provide more relevant suggestions. However, personalisation also raises ethical concerns, including privacy, bias, and transparency. This is why we, designers, play a crucial role in ensuring that personalisation is implemented effectively and ethically. We must understand user needs and preferences through research and testing, and continuously gather feedback to refine personalisation features.https://medium.com/media/6cf6ef6eec5b59e000dc098865a8e440/hrefWe can say that personalisation is a powerful tool in a designers toolkit, but as Armstrong emphasizes, the success of personalised experiences hinges on the thoughtful and ethical application of AI insights. It is crucial to always prioritise the users best interests, ensuring that personalisation efforts are not only effective but also respectful and beneficial to the individual.4. Using AI as a DesignMaterialDesigning for AI is challenging because the technology is invisible and abstract. As designers, if we cant sketch a solution, it feels like losing a crucial ability. As interaction design professor Philip Van Allen puts it, its like our arm is cut off. To address this, he created a no-code programming environment for his students, providing easy access to machine learning. The tool is called Delft AI Toolkit and it simulates an AI system in 3D, allowing designers to observe and manipulate the systems behaviour and data sources in a virtual space before investing time and expertise into model training and building a physicalrobot.https://medium.com/media/7554deb21a70eb4fc12cd2a0689fdd80/hrefAnother approach to working with AI as a design material comes from John Zimmerman, a professor at Carnegie Mellon University. He developed a method that focuses on the AI system capabilities rather than the underlying technology. Zimmerman observes that many of his design students are uncertain of whats possible when working with AI technologies, so he uses a match-making system which helps them grasp the AI systems potential. For example, he presents a two-class text classifier and asks, What could you do with this, and for whom?. This method helps designers identify existing capabilities and apply them in innovative ways. As with any design tool, the more we experiment with it, the more comfortable we become, and the more examples and abstractions of AI capabilities we will generate.Janelle Shanes experiments with DALL-E 3 playfully tease out all the ways algorithms can get things wrong. Here, she jumps into the algorithmic training process to generate candy heart messages. More on: https://www.aiweirdness.com/dall-e3-generates-candy-hearts/A third approach, which I find particularly effective, is using the Object-Oriented UX (OOUX) methodology. OOUX focuses on objects before actions, aligning with how users perceive the real world. It borrows concepts from object-oriented programming but applies them to user experience design. This approach works well with AI workflows by organising data and interactions around objects, making complex AI systems more intuitive for non-developers by matching their mental models. Sophia Prater is a leading expert in the OOUX field, and I highly recommend her materials and podcasts on thesubject.On a personal note, I recently completed my first project using this approach for a native AI product. The methodology was incredibly helpful. It allowed me to visualise the systems functionality and limitations more clearly, which also aided the engineers. Additionally, it made my design process more tangible through object mapping and user flows, enhancing the quality and accuracy of my proposed solutions.A beautifully chaotic collaboration between me and the engineering team using the OOUXapproachML is the new UX. I envision UX practitioners leveraging machine learning as a design material creatively and thoughtfully, guiding users and technologists toward a deliberative ML-mediated futureQian Yang, Cornell University5. Designing to reduce climate impact ofAIAlthough this isnt mentioned in the book and isnt a AI capability, it is certainly one of the key skills designers should possess, especially in the 21st century with the rise of AI technologies.Theres an article called How to Design Climate-Forward AI Companies by Santhi Analytis that emphasises the important role designers play in mitigating the environmental impact of AI technologies. It frames the challenge of AIs growing carbon footprintfueled by energy-intensive processes like model training and data center operationsas one that requires urgent attention from both engineers and designers. Designers can contribute by creating energy-efficient UI/UX elements, minimizing unnecessary data and media loads, and applying green software principles. Additionally, designers can push for socially equitable uses of AI by developing inclusive, climate-conscious user experiences.The Intercept Brasil (in Portuguese) also published last year an article stating that the major tech companies like Microsoft, Google, and Amazon are now turning to nuclear energy as a solution in response to the growing energy demands of AI and data centers. Microsoft plans to reopen the Three Mile Island plant, Google has partnered with Kairos Power for small reactors, and Amazon has invested $500 million in nuclear technology. Many of these data centers are being built in countries from the Global South, where labor and infrastructure costs are lower, raising concerns about resource exploitation. While nuclear energy is carbon-free and efficient, it is costly and carries risks, and critics argue that big tech is addressing a crisis of its own making, prioritizing rapid technological expansion over sustainability.The industrys move fast mentality raises concerns about whether nuclear energy can truly keep pace with AIs accelerating energy consumption, or if it will simply become another chapter in big techs history of unchecked growth. This Vox documentary tries to answer this question:https://medium.com/media/8d8c5a33bba53566072d960d2be0f0f4/hrefIn this other article from MIT, the author Andrew Winston also highlights the growing environmental impact of AI and provides recommendations to reduce its carbon footprint, such as using existing models instead of creating new ones. It emphasises the role of designers in this issue, urging them to develop AI expertise to simplify complex concepts, communicate the nutriscore of AI in their products (its usage and environmental cost), and adhere to an ethical code of conduct similar to the EUs AI Act, ensuring AI products are safe, transparent, traceable, non-discriminatory, and environmentally responsible.As designers, we can influence user behaviour and company policies by incorporating transparent data models that reflect the true costs of AI, thus ensuring the technology not only solves human problems but also helps tackle environmental challenges.Conclusion: Lets takeactionIts easy to feel overwhelmed by the urgent need for AI ethics. AI is a versatile and dynamic field, capable of performing a wide range of tasks that can help solve real-world problems. However, algorithmic systems often reflect and amplify existing societal biases. Until interfaces clearly communicate the logic behind these algorithmic decisions, users wont be able to hold these systems accountable. This is why designers and the communities they serve need to understand digital rights. We must hold ourselves and the industry accountable for the choices we make through ourdesigns.The key question is: Will we allow machine learning to prey on those already victimised by society, or will we use this technology as a mechanism for equity andjustice?Here are some actions we can start taking as designers in this industry: Design for anticipation to prepare for multiple possible futures, making our designs more flexible and future-proof Think critically about the long-term impacts of our designs and the data-driven decisions that underpinthem Articulate digital rights and guide users with transparency toward options through design interactions Break down complex privacy agreements into quick, comprehensible, just-in-time interactions Prototype future-facing concepts, like personal privacyagents Support collective digital rights organisations and community-driven dataset initiatives Ask early questions about ethics and biases to ensure technology adapts to human needs, not the other wayaroundHow do you approach building AI products? What examples do you know of ethical and inclusive design solutions?ReferencesBig Data, Big Design: Why Designers Should Care About Artificial IntelligenceHelen Armstrong (Book)Design and the Question of HistoryTony Fry, Clive Dilnot, Susan Stewart(Book)Human-Centered Design for AIWebinar by Niwal Sheikh (Product Design Lead, Netflix) byIxDFHow to Design Climate-Forward AI CompaniesArticle by SanthiAnalytisThe problem with AI development today: Designers need to step upArticle by SarahTanWill AI Help or Hurt Sustainability? YesArticle by AndrewWinstonWhat is predictive AI?Article by CloudflareReviewing the Terms & Conditions of popular generative AI toolsArticle by DavidSerraultThe Problem with AI Development: Designers need to step upArticle by SarahTanDesign For AI (Artificial Intelligence)Article by Sudarshan SahuDesign Against AIWebsite by JohnMaedaThe Intercept: Big techs apelam para energia nuclearArticle from Portal InvestNE mentioning the original piece from The Intercept BrasilOOUXWebsite by SophiaPraterAI Now InstituteWebsiteWhy AI (desperately) needs designers was originally published in UX Collective on Medium, where people are continuing the conversation by highlighting and responding to this story.0 Comments ·0 Shares ·118 Views
-
Pokmon Go: addictive by designuxdesign.ccHow Niantic utilizes emotions to manipulate player behavior.My bookcase, top shelf so you know itsgood.One of my favorite Pokmon is Trubbish, a tiny trash bag full of personality. Trubbish claims this spot for many reasons: for being from the region based on my home of New York City, for rightfully roasting the city as dirty, and in no small part for all the undeserved hate received upon its initial reveal. This silly little guy is just a toothy cutie with so much love togive.One of the great things about Pokmon as a brand is how every single creature is a favorite for somebody somewhere. The series has a curious way of bringing together a diverse assortment of people through shared passion. Pokmons staying power stems from the attachments we form with our creatures. I still have Pokmon caught and trained over decades in digital storage. The thought of parting with any of my massive collection gives me no amount of pleasure.That very same power stemming from emotional bonds is also utilized by the mobile spinoff Pokmon Go to harness its own unique power: the ability to be so poorly designed yet so deeply addictive. Niantic, the developer of Pokmon Go (PoGo) twist this strength to their advantage, using it to hook players through repeating cycles of gain and loss. Players become ensnared within an ecosystem designed to encourage the chase of that next high through the apps use of time-limited manufactured scarcity. The scarcity, in turn, causes FOMO, the fear of missingout.Please dont misunderstand. I very much enjoy Pokmon Go; its my favorite addiction. As a player myself, I write this article holding both love and fear inside me. I deeply love the Pokmon brand as well as my creatures contained within the mainline series games and mobile PoGo app. I also feel fear, specifically of some form of retaliation. The developer has a demonstrated pattern of treating players with disdain and responding in unpredictable, often unhelpful ways.As a designer however, I cannot help myself, questioning and dissecting the mechanics utilized to keep me coming back for more. Despite the realization I am often not having fun engaging with gameplay mechanics, WHY do I keep playing? What does the app actually offer? Well, it offers FOMO. A seeming lack of respect for players. A mediocre to poor user interface. Inconsistent accessibility advances. Shifty data collection practices. And yet, despite it all, I still find myself thinking about the app first thing in the morning and right when I close my eyes atnight.Pokmon Go launched in 2016, revitalizing the Pokmon brands popularity to levels not seen since the Pokmania of the late 1990s. Pokmon itself has long been a mainstay of pop culture, becoming the highest-grossing media franchise of all time spread across games, animation, trading cards and more to a total sum of over $100 billion USD. While Niantic has multiple apps under their care, PoGo is by far their most popular. It has helped the company grow their valuation to nearly $9bUSD.These Pokmon-themed jumbo jets epitomize the omnipresence of Pokmania in the late90s.As a longtime Pokmon fan, I downloaded PoGo on day one but found the app lacking depth. It wasnt until a few years had passed that I returned to find a wildly different experience. As a designer, I discovered a decent product without sufficient care applied to craft its interweaving systems and interactions. As a player, I discovered my very first Pokmon dressed in a fun Halloween costume. Which is then stronger, logic oremotion?Overall, the apps interface is satisfactory but unoptimized. As a daily player, the locations of numerous key features used semi-regularly remain challenging to learn. I am consistently seeking out how to initiate a playgroup known as a party because access is buried behind a three-tab submenu within the page detailing player stats. Such difficulty is common throughout the entire experience. Features are located within unintuitive menu locations. Such design choices fail to facilitate the easy formation of mental models that aid user comprehension.There are numerous examples of this throughout the app. News updates are contained behind a two-tab submenu at the top of screen under three levels of pages from the bottom of another screen. Thats at least three clicks and several moments of confusion before remembering the proper path. Despite gaining a sort of muscle memory to brute force my way through the interface, I have to apply conscious effort often to complete tasks. It is the ineffective (bad) design itself that screams, I dont know where anythingis!One of the key tenets of Pokmon is catch em all. Doing so has always required trading between players. PoGo actively discourages trading, a key gameplay mechanic. Within the app, the trading user flow (the path taken from screen to screen) is slow and clunky. Rather than encourage trading through a quick, seamless experience, the app wastes players time booting them out to the main page after each completed trade. To their credit, finding your way back to the trade only requires clicking into player stats, navigating the submenu to locate the friend list, then scrolling through your full list (or sometimes not.) At least the actual trade menu is a confusing design with unclear instructions for navigating forward or back. Simple, effective design.Similar time-wasting interactions crop up throughout the broader experience, not merely trading. A few seconds often does not amount to anything significant. However, these interactions accumulate within an environment that creates demand through the use of time-limiting content. Fighting Rocket grunts, PoGos main antagonist, includes three to ten seconds of flavor text without any diversity, practical use or method to disengage. Raid battles, the equivalent of boss fights, include lengthy intro animations. Most battles end with generous animations (three to five seconds) and again more flavor text. Keep in mind that both battle types are time-locked to no more than 45 real-world minutes in total. For an app built around catching the best creatures, a lot of time is wasted getting to the part where you can catch creatures.As far as accessibility is concerned, the current design is inconsistent. A number of great features do exist. One piece of clever design allows single-handed play. One broadens the use of haptic feedback. Yet another combats color blindness, including visual icons to display weather or shiny statuses. (For those unaware, shiny Pokmon are rare spawns with alternate color combinations.) Catching shiny spawns is always a moment of excitement and triumph, yet someone with color blindness might miss out without non-color-based notifications. Kudos,Niantic.However, such successes providing equal opportunities to all players are quickly matched by careless design. The capture success ring, used to aim and display the probability of catching a Pokmon, is expressed solely through color. A colorblind person would need to learn other methods to comprehend the chances of success or risk wasting supplies. A colorblind person may not even realize the feature exists. A handful of small visual functions exist that I only personally learned of through my local community sharing tips and tricks in conversation.I will show respect to Niantic for the consistent A/B testing performed to optimize the app (or optimize, depending on how pessimistic the app makes me feel any given day) with various visual and layout tweaks. Some changes have made the app more aesthetically appealing though also more technologically demanding. Other changes, such as the overhaul of player avatars, have been met with fierce backlash from the player community. Despite vocal opposition and continued pressure on the developer, Niantic saidnothing.A developer has every right to change their product to suit the aspirations they envision for success. However, a product cannot be successful without customers. Consider the numerous examples of Niantic promising specific bonuses for timed events (remember FOMO: if you dont play now, youll miss out!) only to backtrack later via tweet offering decreased or entirely different bonuses. Examining any design tweaks alongside their history of and inability to properly message official changes via press releases or other channels, I often dont know what to expect at best or am expecting something different at worst. For a company worth $9b USD, this concedes a basic lack of respect for players. Perhaps it is a sign of internal management issues. (Why notboth?)It is important to understand how design can play a role in creating addiction before continuing. In his book Hooked, Nir Eyal details how habits, a precursor to addiction, can be built through certain repeating design patterns. These frameworks occur over four stages. Initially a Trigger, such as a push notification or advertisement, is required to cause a user to engage with a product or app. Afterward, an Action is taken, whether clicking a button, scrolling a feed or catching aPokmon.A visual representation of the different stages in Nir Eyals Hooked model, courtesy of the authorswebsite.This leads to the most critical step in the process, the Variable Reward or any unique content that changes upon each individual interaction with a product. Offering something new with each experience subsconsciously teaches users that something great is always just around the corner, such as a video that makes us smile or that rare Pokmon spawn we have been seeking out. This variation in what we find, specifically when it is something we like, causes the release of dopamine in the brain which in turn causes us to feel good. Positive feelings keep users engaged with a product longer. Just one more swipe. Just one more round. Just another minutemore.By engaging often enough, the user will begin to establish the Investment within a product. Investment operates similarly to the sunk-cost fallacy, where users feel commitment through the amount of time or effort placed into a product. This could potentially look like establishing a lengthy list of personal friends on Facebook, a photo collection of life experiences on Instagram or even a trained roster of creatures in PoGo. Consider me guilty ascharged.After enough passes through this Hooked cycle, users eventually internalize what were once external triggers, converting them into internal triggers. A push notification alerting you of new photos from your friends becomes a thought: I wonder what my friends posted online. I should check. Internal triggers can manifest as thoughts or feelings. It is here that a habit isborn.Habits and addiction can be viewed as two sides of the same coin. Both are patterns of recurring thoughts, both conscious and subconscious. Transitioning from habit into addiction is not only nuanced but also outside my area of wisdom and expertise. Lets instead examine how Niantic uses these tactics to build a product that utilizes emotion to keep users in their mobile ecosystem in spite of the previously discussed poordesign.As mentioned, I stepped away from playing PoGo at launch. The mechanics did not translate to my brain which was more familiar with the mainline Nintendo games. Returning to the app to catch costumed Pokmon was an exciting experience that the mainline games did not have. What a fun way to see my first Pokmon! After spending time growing comfortable with the app and later integrating myself into a local player group, I learned the general sentiments stewing within the community. Top of this list? Niantic only cares about the money, not theplayers.Caught 10/25.From a design perspective, I find this subjective sentiment to hold some objective weight. Examining recent updates, the app has made it easier and quicker to spend Pokcoins, the in-app currency. Coins can be acquired slowly through gameplay (a daily limit of 50) or more quickly and in more significant amounts through real-world currency (at a rate of roughly 100 coins per $1 USD.) I find it revealing that facilitating microtransactions via the proliferation of coin usage and event tickets takes precedent over quality of life, gameplay-centric improvements but I do not find it surprising.Designing for sales via microtransactions is not new, having become a genre unto itself known as gacha games. What is new is Niantic finding mainstream gaming success. Pokmon Go is an outlier, the developers most successful and profitable product by a wide margin. Without the strength of the Pokmon brand behind it, PoGo most likely would not have found anywhere the level of gaming success ithas.The most recent updates provide more options to spend your coins in locations where the app makes interaction unpleasant. For example, I often find my in-app storage full. To catch more Pokmon, I need to have free space. A new button recently appeared, encouraging me to buy more storage space directly from within my storage instead of requiring me to make a conscious choice to navigate to the in-app shop. An optimist would argue this makes the action easier to complete. A realist would point out how slow it is to sort through Pokmon in storage, making clearing space one of the most labor-intensive tasks in the entire app. A pessimist would ask, Why waste time clearing space when you can instead spend money to get back to gameplay more quickly?This focus on encouraging spending habits through specific interactions extends even further. A newer feature to the app, small and large Pokmon (XXS and XXL respectively) offer nothing to gameplay outside of the anemic PokContest feature. Contests can be entered to win rewards such as supplies and experience points. Critically however, the Pokmon used in contests take up additional storage space. The apps newest feature repeats this pattern. Known as Dynamax raids, these particular Pokmon are primarily used to catch additional Dynamax Pokmon. Instead of using previously caught creatures, the app encourages players to catch additional copies in order to catch more, initiating a repeating cycle, taking up storage space and making the need for additional storage increasingly necessary.Also worth noting is players can opt to spend coins after Dynamax raids to increase the rewards received. Again, the pessimist asks, Why play more when you can spendmore?The screen players encounter after a completed Dynamaxraid.For rural players or those with disabilities, one of the most vital features facilitating playability and accessibility is a remote raid pass allowing access to high-level raids. These remote passes remove the need to physically be present in a location with sufficient players, typically dense urban environments. High-level raids are often the only way to gain powerful and/or legendary Pokmon. In a shocking reversal, the developer limited the number of remote raids allowed daily and increased the cost of passes 250%, from $1 USD to $2.5USD.This has been a sore spot in the community for ages. Niantic chose to discourage remote raids instead of encouraging in-person raids. Disappointingly, Niantic chose to do so in a way that actively hurt their most disadvantaged players instead of uplifting those able to play in the specific way the developer deemed as optimal. Negative reinforcement (increased real-world cost and less ability to participate for some) was utilized over positive reinforcement, such as better stats or rewards for in-person raids. Overall, I think this says a lot about the relationship between developer andplayer.Why though? Why choose to make such changes? That is the real question.All the information presented so far supports my primary assertion: Pokmon Go is intentionally designed to promote specific player behaviors through means both subtle and overt using the Pokmon franchise as leverage Except the behaviors discussed are not the behaviors intended.To put it simply, Pokmon Go is not aiming to be a thoughtful or well-designed game. The app preys on our emotional nature as human beings to encourage purposeful play patterns through the use of manufactured scarcity. The app solidifies these patterns into habit in service of collecting user GPS / location data. It hides this goal behind creature-collection gameplay, wearing the Pokmon brand as a mask like an antagonist walking through a foggy setting in a horrorfilm.(Note: This design ethos is in direct contrast to Nintendos own design creed, optimizing software for the fun and enjoyment of playing games. It is important to point out that while Nintendos design philosophy emphasizes the joy of play, their business philosophy is distinctly less consumer-friendly. For the purposes of this article, I am focusing on the philosophy and principles of design, not business.)The app is designed as a means to collect geospatial data in large quantities. That is speculation on my part. However, when I shift my perspective when considering Niantics design goal from earning revenue via microtransactions or even creating a fun Pokmon game to collecting GPS information, a lot of decisions in the app regarding encouraged player behavior make moresense.Niantic was founded in 2001 by a team of geospatial scientists and researchers as an augmented reality (AR) company. Their first project was an interactive map and model of the planet known as Keyhole. Three years after its founding, the company and its product were both acquired by Google who later renamed the product as GoogleEarth.For a company with expertise in complex navigational mapping tools, the transition to gaming was certainly distinct. I dont believe it is fair to suggest that developers at Niantic might completely lack any passing interest in video games. However, it is fair to suggest that leaping from one product category to another, entirely different category would not maintain some traces of what camebefore.Niantic used their development knowledge to create a product that habitualizes users into walking vast distances, collecting GPS data of routes, roads, paths, etc. as the user played. They utilized the strength of the Pokmon brand to supply reasons for users to want to collect this sort of data. By limiting the availability of creatures available, the app preys off players fear of not collecting powerful versions of their favorite Pokmon. FOMO and purposeful design choices turn players preexisting desire to catch em all into an addictive act, very cleverly supplying the developer with a recurring source of the type of revenue actually soughtafter.Piggybacking off the Pokmon brand was truly inspired. It allowed Niantic to make a product with minimal polish and lackluster game design techniques because Pokmon the brand had nearly twenty years of already established history when Pokmon Go launched in 2016. History breeds loyalty. Loyalty breeds a willingness to overlook flaws. The Pokmon brand breeds a unique, if entirely fabricated, emotional connection to creatures that exist only through digital sprites, animated cartoons and stuffed plushies.Perhaps it really is just a product meant to earn income through player purchases. That is plausible. Consider this: while the brand attracts a wide range of ages and backgrounds, Pokmon has always held a large percentage of children among its player base. Knowing how the app utilizes emotion as a means to encourage specific play behavior, how ethical is it to design a product with that goal? Does it become less ethical when considering the clear correlations gacha gaming has with gambling and the ages of the Pokmon user population?I cannot provide definitive answers to those questions. Perhaps the apps goal is truly as straightforward as collecting income via microtransactions. Perhaps itsnot.Niantic recently announced its plans to use data collected from users of its products to create a large geospatial model to train AI models to navigate within the physical world. Perhaps this is a positive thing; perhaps not. Do we choose to again trust a company who manipulated us once already? Its turtles all the waydown.Living in a world where artificial intelligence (AI) not only exists but is proliferating, we as humans have to shift our mindsets and willingness to accept facts in certain ways. AI can quickly and easily create almost-lifelike content without the need for a skilled artist to supply talent, time and desire. These tools have shifted the status quo. We as humans must learn to adapt as well. This requires self-awareness and flexibility, two key strengths in our modernworld.Once again, please dont misunderstand. As both a designer and player, I aim to supply the tools, knowledge and conversation to allow informed choices so that users have the agency to make their own decisions. All too often, choices are made for us, most often recently with the goal of training cryptic AI systems with obscured motives. What do we sacrifice in service of such goals? What benefit do wereceive?Humans have been smarter than computers for all of recorded history so far. That may change one day. We as humans have the benefit of emotion and complex reasoning abilities whereas computers are better at precise calculation. Until the day comes when computers become smarter than us (and hopefully beyond that day), we have the ability tochoose.To choose whether we want to sacrifice our agency and our data to companies whose motivations we cannot be sureof.To choose how we utilize our human freedoms and community.To choose which Pokmon gets the status of being labeled somebodys favorite.Trubbish, a toothy cutie with so much love togive.Pokmon Go: addictive by design was originally published in UX Collective on Medium, where people are continuing the conversation by highlighting and responding to this story.0 Comments ·0 Shares ·121 Views
More Stories