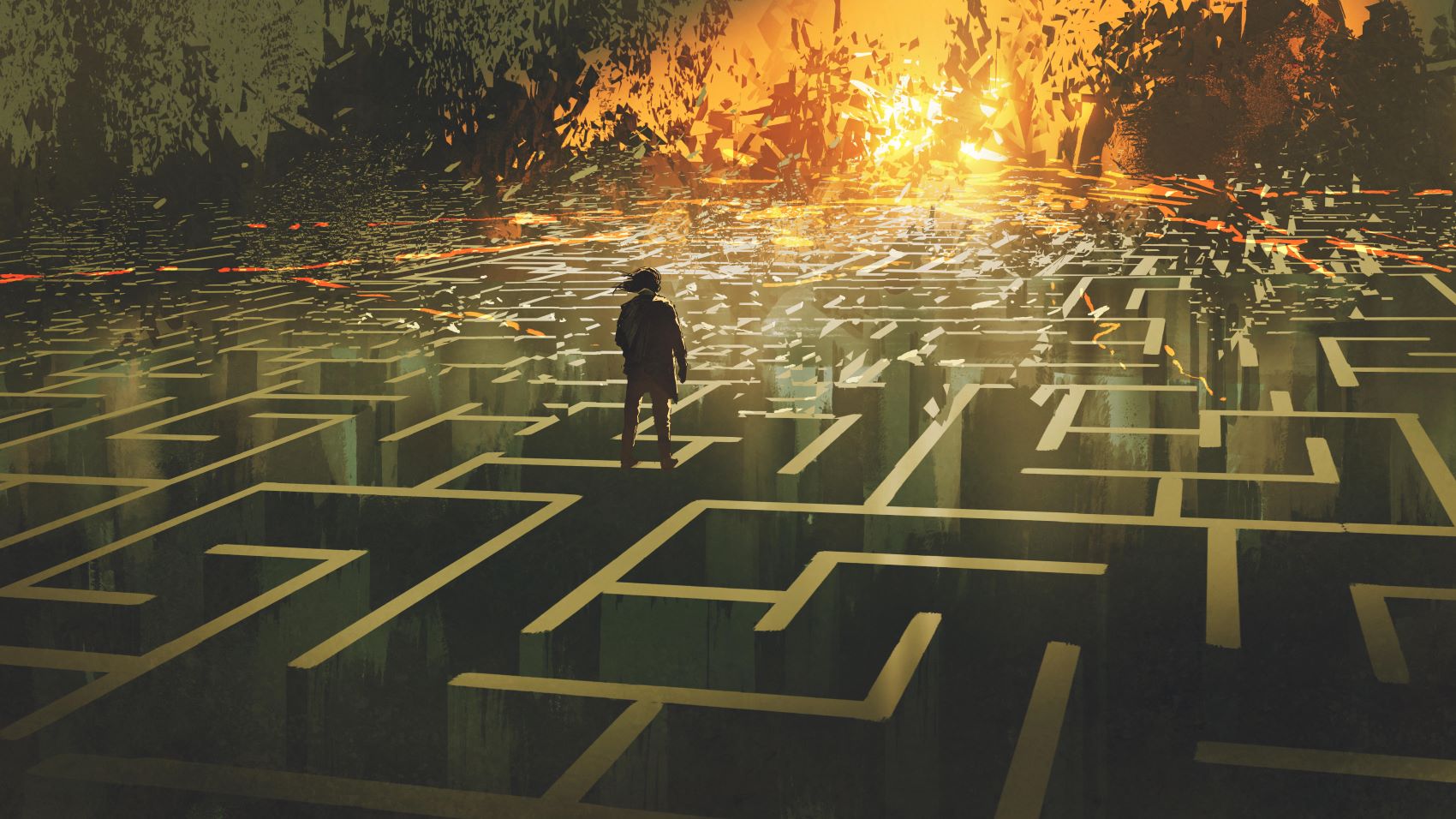
AIs Hidden Cost: Will Data Preparation Break Your Budget?
www.informationweek.com
Shane Snider, Senior Writer, InformationWeekFebruary 4, 20256 Min ReadTithi Luadthong via Alamy StockDuring many major tech conferences and events in 2024, talk of implementing artificial intelligence was a common theme as IT leaders are tasked with creating new GenAI tools for business. But a common refrain was the need to prepare data for machine learning.That need for clean data may slow AI launch efforts and add to costs.A recent Salesforce report found CIOs are spending a median of 20% of their budgets on data infrastructure and management and only 5% on AI. A lack of trusted data ranked high on the list of CIOs' main AI fears. In another report, research firm International Data Corporation (IDC) says worldwide spending on AI will reach $632 Billion in 2028.The industry was caught off guard as OpenAIs ChatGPT quickly launched the GenAI arms race two years ago -- many companies are faced with juggling data needs with getting that data AI-ready. Spending on data preparation could be a significant upstart cost for AI, varying with the size and maturity of different businesses and organizations.Preparing data for AI is a tricky and potentially costly task. IT leaders must consider several factors, including quality, volume, complexity of data, along with preparing for costs associated with data collection, cleaning, labeling, and conversion suitable for an AI model. When added on top of needs for new hardware, software, and labor costs associated with GenAI adoption, and the bills add up quickly.Related:CIOs and other tech leaders are faced with presenting AI as a potential value creator and possible revenue generator. But many companies face an uphill battle when it comes to ROI on new GenAI programs, the time and cost to prepare data often doesnt lead to immediate returns.Spending Money on Data to Make Money with AIBarb Wixom, author and principal research scientist at MITs Center for Information Systems Research (MIT CISR), says leaders can point to specific successes at other companies that have more mature AI rollouts. Those companies, she says, have built strong data value through forward-looking governance.AI has to be viewed, not as AI, but as a part of the data value creation or data realization, she tells InformationWeek in a phone interview. I call it data monetization converting data to money. If organizations and especially leaders just consistently think about AI in that context, you wont have a problem if an organization is trying to reduce its cost structure by a certain percentage, or trying to increase sales in some way, or increase service growth -- whatever the objective is -- thats often big money. Even if you have an extraordinary investment in AI, the outcome could be orders of magnitude greater.Related:With tech budgets tightening in the face of macroeconomic woes, IT leaders need to convince non-technical members of the C-suite that data preparation is a worthwhile investment. Wixom points to success stories in the financial services industry where IT leaders had strong credibility within their executive team. One such leader, she says, used an internal consulting group to accumulate use cases to present a more traditional business plan to executives. They road-mapped how they were going to build out over four years -- they were able to deliver that, Wixom says.But other organizations may not be as mature in their data governance as a major financial institution. In those cases, an incremental, bottom-up approach can be effective as well. You dont have to start with the vision of all thats going to be done but by taking an incremental approach that builds capability, where you learn along the way and establish not silos, but a growing enterprise resource.The next step: Finding the right architecture to align with your AI goals. Data mesh and data fabric are two competing modern data architecture frontrunners that are similar but have key differences.Related:Mesh or Fabric? Modern Data ArchitecturesIn the pre-GenAI era, data governance was relatively straightforward. Many companies pooled data into data lakes that stored large amounts of raw data. For AI use, that generalized architecture can create bottlenecks that hinder productivity. Data fabric and data mesh architectures are becoming the new industry standards when it comes to GenAI implementation. Thats because these modern architectures integrate data from multiple sources into a unified view, simplifying data maintenance, and reducing time and costs.Data Mesh:Using a data mesh architecture can be a good option for those looking to empower separate business units with data ownership.Data Fabric:Data fabric offers centralized architecture, integrating data across an organization. This method allows a unified data structure with a central governance.But those new architectures come with a price. Higher startup costs and ongoing maintenance fees can pose significant barriers to entries for some enterprises, depending on the size and current state of data governance. Data mesh will likely have higher up front costs. Data fabric has lower implementation costs but will likely cost more to maintain.So, its important to understand potential use cases to justify the spend and to understand which architecture is right for your organization, experts say.Inna Tokarev Sela, chief executive officer and founder of data fabric firm Illumex, points to specific use cases that can most benefit from modern data architectures. She says organizations that can most benefit from data fabric include those which aspire to create a degree of automation, self-service access to data analytics by business users, workflow automation, and process automation. She says businesses with disparate teams who need to use data to build analytics and collaborate can benefit from a data fabric architecture.Data fabric and data mesh are like the Montagues and Capulets, or the Hatfields and McCoys, says Kendall Clark, co-founder and CEO of data firm Stardog. Its like a frenemy rivalry they are so similar that nobody can tell them apart, but its the small differences.Because data fabric is so similar, Clark says clients will request data fabric but what they are really describing is data mesh architecture. So, its more important to have a strong understanding of your businesses unique data needs. The labels really arent that important.Where to Start? Finding the 'Rallying Point'You dont have to get the decision right, you just have to choose, Clark says of picking a new data architecture for GenAI implementation. I would start by picking a super critical, important problem that will make a real difference for your organization. Something that will make your business save more money, manage risk, make more money, make people more productive -- those are the keys to driving the business forward. You need to pick one as your rallying point.No matter your starting point, a successful switch to any data architecture requires clean, well-governed data, MIT CISRs Wixom contends. It doesnt matter if its data mesh or data fabric, if we just do the practices the way we really should for instance, like using good metadata, all of the sudden, you have interoperability because you have consistency and standards. The problem is that most organizations are silos and spaghetti -- they havent followed the textbook rules to begin with so theyre in remediation mode.About the AuthorShane SniderSenior Writer, InformationWeekShane Snider is a veteran journalist with more than 20 years of industry experience. He started his career as a general assignment reporter and has covered government, business, education, technology and much more. He was a reporter for the Triangle Business Journal, Raleigh News and Observer and most recently a tech reporter for CRN. He was also a top wedding photographer for many years, traveling across the country and around the world. He lives in Raleigh with his wife and two children.See more from Shane SniderNever Miss a Beat: Get a snapshot of the issues affecting the IT industry straight to your inbox.SIGN-UPYou May Also LikeWebinarsMore WebinarsReportsMore Reports
0 Comentários
·0 Compartilhamentos
·54 Visualizações