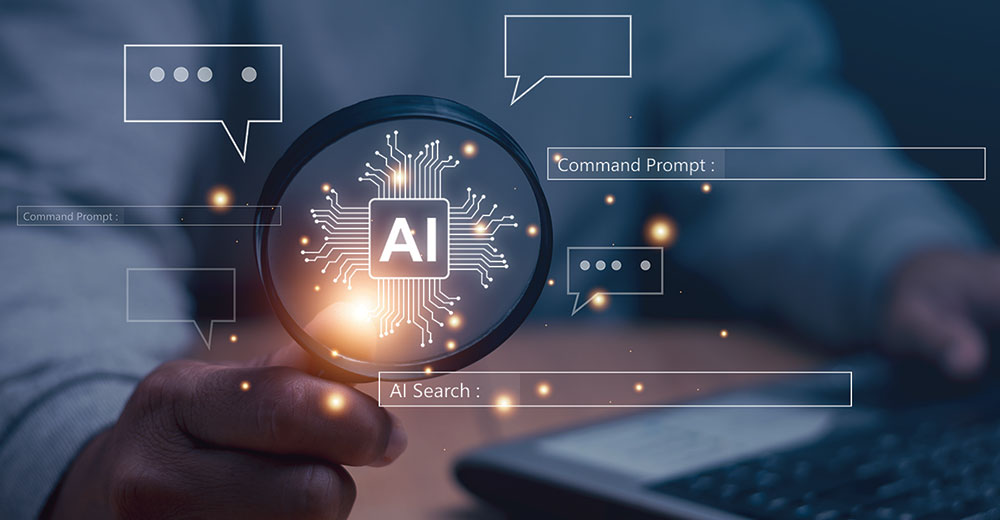
WWW.TECHNEWSWORLD.COM
AI Search Threatens Digital Economy, Warns Researcher
AI Search Threatens Digital Economy, Warns ResearcherBy John P. Mello Jr.November 12, 2024 5:00 AM PT ADVERTISEMENTYour Salesforce Data, Your ResponsibilityLearn best practices to effortlessly protect your Salesforce Data with Rubrik. Find Out How Search engines using artificial intelligence could harmfully disrupt the digital economy, warns a researcher at Harvards Berkman Klein Center.[I]f AI search becomes our primary portal to the web, it threatens to disrupt an already precarious digital economy, Benjamin Brooks wrote in a recent article in the MIT Technology Review.Today, the production of content online depends on a fragile set of incentives tied to virtual foot traffic: ads, subscriptions, donations, sales, or brand exposure, he explained. By shielding the web behind an all-knowing chatbot, AI search could deprive creators of the visits and eyeballs they need to survive.Brooks urged the AI industry to address the content compensation problem before others do it. The AI industry should use this narrow window of opportunity to build a smarter content marketplace before governments fall back on interventions that are ineffective, benefit only a select few, or hamper the free flow of ideas across the web, he wrote.We need to bear in mind that these new systems, these new business models, are just taking off, he added in an interview with TechNewsWorld, but however we respond to these challenges, its important we do so in a thoughtful, measured and targeted way. Thats why industry should take the lead here.Government is now more confident regulating content and regulating bargaining than it ever was before, he said. The AI search industry should be mindful of that. With that additional pressure over the coming years, the industry should get ahead and build a smarter solution before government turns to blunter solutions.AI Search Impact UnclearSo far, the impact of AI search on the wallets of content creators is still foggy. Its not yet clear, but there is a strong argument to be made that there will be reduced traffic to many publishers, said Greg Sterling, co-founder of Near Media, a news, commentary, and analysis website.The evidence is mixed, he told TechNewsWorld. During the Google SGE period, there was evidence that organic links were being pushed down the page and therefore [were] less visible. Introduced in December 2023, Googles Search Generative Experience provides overviews of search topics using AI.However, he continued, there was little research on actual click behavior. Google claims that links within AI results get more engagement. We need to do more research on this issue.Chris Ferris, senior vice president of digital strategy at Pierpont Communications, a public relations agency in Houston, added that AI search will exacerbate the problem that already exists with traditional search. Most web pages dont get any traffic from organic search, he told TechNewsWorld.He cited research published by Search Engine Land predicting that organic traffic will fall between 18% and 64% because of AI search. Mark N. Vena, president and principal analyst at SmartTech Research in Las Vegas, noted there is growing evidence that AI-driven search, such as generative AI summaries in search engines, has caused a decline in click-through rates to content providers websites, as users increasingly consume information directly from AI responses.News outlets and niche content creators have reported reduced traffic from traditional search sources when AI systems generate comprehensive answers, he told TechNewsWorld. While detailed impact studies are ongoing, this trend indicates potential risks to publishers ad revenue and visibility.Without doubt, AI-driven search tools risk reducing traffic to content providers sites, potentially undermining ad revenues and subscriber models, he added.Without users clicking through to original sources, content creators may struggle to monetize their work, threatening the sustainability of quality journalism and niche content. Balancing AI-driven convenience with adequate attribution and redirection to content providers will be essential to preserve a healthy digital content ecosystem.Eyeball Apocalypse OverblownDev Nag, CEO and founder of QueryPal, an enterprise chatbot based in San Francisco, asserted that the narrative that AI search will destroy content creation by stealing eyeballs fundamentally misunderstands how content ecosystems have evolved.Think about how we transitioned from paid newspapers and centralized TV/movie studios to ad-supported online content, he told TechNewsWorld. Each shift brought predictions of doom, yet we ended up with far more content and from more content creators than ever.AI is poised to dramatically expand content reach through better discovery, translation, and personalization. Rather than destroying the content economy, AI search is likely to create a more efficient marketplace where quality content finds its intended audience more effectively.He contended that the evidence so far doesnt support the eyeball apocalypse narrative.While AI search is changing how people discover content, were seeing content creators adapt by producing more focused, high-quality material that AI systems can better understand and distribute, he said. The real transformation isnt about losing eyeballs. Its about shifting from a mass-market advertising model to more sophisticated monetization approaches.Nag predicted two primary models emerging: content licensing, where creators get paid for allowing AI systems to learn from and reference their work, even if its available openly otherwise, such as the recent Google-Reddit agreement, and a value-share system, where AI platforms distribute revenue based on how often they reference and synthesize a creators content. Thats currently possible with RAG-based systems that are able to provide explicit references like Perplexity and could be adapted to systems which are directly trained through sophisticated attribution tracking, he explained.Search engines could do as TikTok and YouTube do and share their revenue to build up creators feeding their services, added Rob Enderle, president and principal analyst at the Enderle Group, an advisory services firm in Bend, Ore.However, as AI advances, he told TechNewsWorld, it may need less and less human creators, which will be problematic to this future outcome.Ross Rubin, the principal analyst at Reticle Research, a consumer technology advisory firm in New York City, pointed out that AI search is the culmination of something seen over the course of decades.Back in the day, long before AI, there was Ask Jeeves, he told TechNewsWorld. It didnt execute on the idea very well, but the idea was, rather than get a whole bunch of links, you got an answer. In many cases, thats what the person searching wants. Its a better experience to be given information up front and not have to piece it together or track it down from multiple information sources.Downside of Big Content DealsIn his article, Brooks was critical of AI companies cutting deals with big media companies to avoid litigation or government intervention. This policy of selective appeasement is untenable, he wrote. It neglects the vast majority of creators online, who cannot readily opt out of AI search and who do not have the bargaining power of a legacy publisher.It takes the urgency out of reform by mollifying the loudest critics, he continued. It legitimizes a few AI firms through confidential and intricate commercial deals, making it difficult for new entrants to obtain equal terms or equal indemnity and potentially entrenching a new wave of search monopolists.In the long term, it could create perverse incentives for AI firms to favor low-cost and low-quality sources over high-quality but more expensive news or content, fostering a culture of uncritical information consumption in the process, he added.At this point in the development of AI search, Sterling said its still too early to tell how the game will play out. We have many assumptions and fears but need to test them and produce real data so that were not operating from a place of pure conjecture, he observed.John P. Mello Jr. has been an ECT News Network reporter since 2003. His areas of focus include cybersecurity, IT issues, privacy, e-commerce, social media, artificial intelligence, big data and consumer electronics. He has written and edited for numerous publications, including the Boston Business Journal, the Boston Phoenix, Megapixel.Net and Government Security News. Email John.Leave a CommentClick here to cancel reply. Please sign in to post or reply to a comment. New users create a free account.Related StoriesMore by John P. Mello Jr.view allMore in Search Tech
0 Comments
0 Shares
149 Views