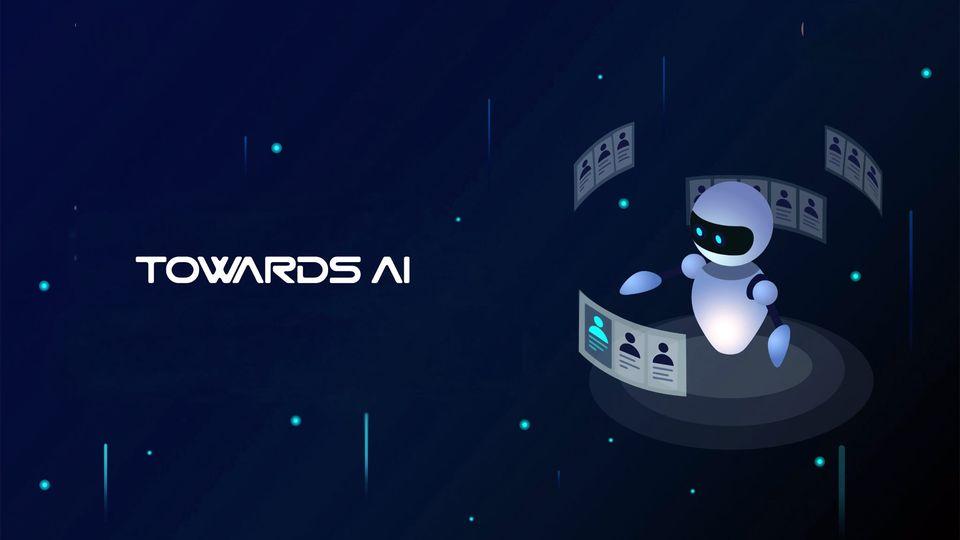
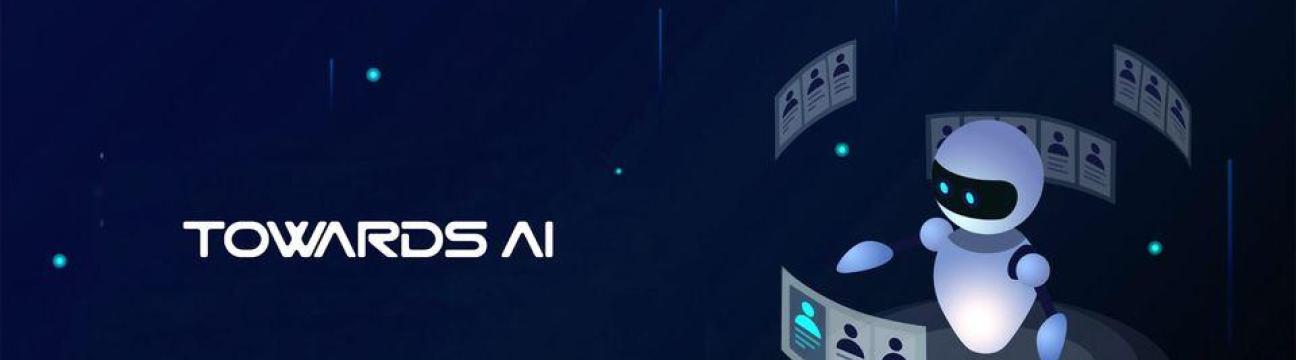
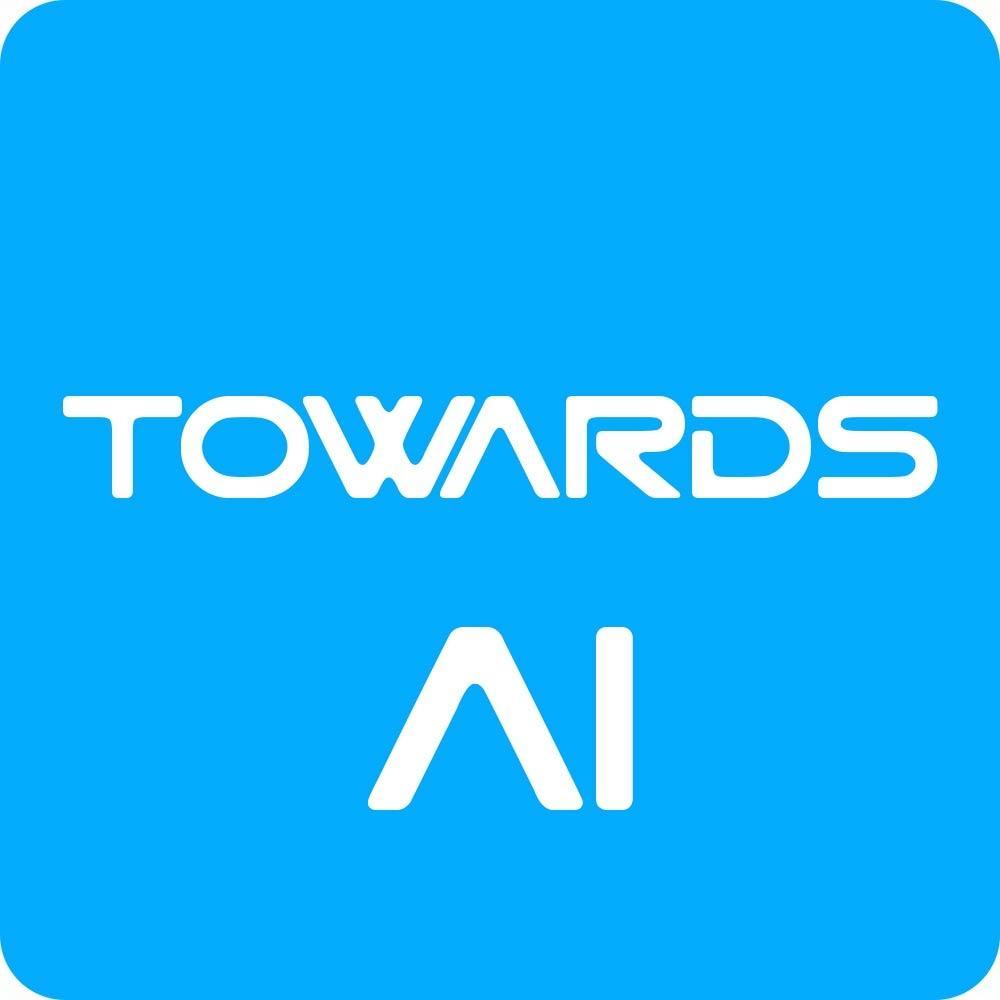
The leading AI community & content platform making AI accessible to all.
2k writers | 330k followers
2k writers | 330k followers
1 people like this
361 Posts
2 Photos
0 Videos
0
Reviews
Share
Share this page
Recent Updates
-
40 Ways DeepSeek AI Will Upgrade Your Life Instantlytowardsai.net40 Ways DeepSeek AI Will Upgrade Your Life Instantly 0 like February 25, 2025Share this postAuthor(s): Mukundan Sankar Originally published on Towards AI. Say Goodbye to Stress This AI Assistant Handles Everything from Finances to ChoresThis member-only story is on us. Upgrade to access all of Medium.Image generated using ChatGPT by the authorImagine having an assistant who helps you juggle work, finances, family, and personal growth all without judgment. Thats what DeepSeek promises to be. Unlike traditional AI models that keep things hidden, DeepSeek is open and transparent, meaning you can trust and understand how it works. But what does this mean for you? How can an advanced AI model actually help in the real world? Lets dive into 40 practical, everyday ways DeepSeek can make life easier.DeepSeek is an AI model designed to be smarter, clearer, and more accessible than traditional AI. It doesnt just spit out answers it explains why those answers make sense. Imagine talking to a super-intelligent friend who actually walks you through their reasoning, instead of just handing you an answer with no context.Unlike many AI models, DeepSeek is open-source. That means developers and businesses can improve it, make it more accurate, and customize it to work for everyday people not just big tech companies.Meal Planning & Recipes DeepSeek can revolutionize your cooking routine. Imagine coming home after a long day and having an AI assistant Read the full blog for free on Medium.Join thousands of data leaders on the AI newsletter. Join over 80,000 subscribers and keep up to date with the latest developments in AI. From research to projects and ideas. If you are building an AI startup, an AI-related product, or a service, we invite you to consider becoming asponsor. Published via Towards AITowards AI - Medium Share this post0 Comments ·0 Shares ·4 Views
-
Words Matter: Are Language Barriers Driving Quiet Failures in AItowardsai.netAuthor(s): Kris Naleszkiewicz Originally published on Towards AI. The AI revolution is upon us, transforming how we work, live, and interact with the world.Yup. We know. Weve all heard.The media loves to cover spectacular successes and failures.But what about the quiet failures? The stalled projects. The initiatives that never quite get off the ground. Not because the technology doesnt work but because something more human gets in the way.Is this a familiar situation? Youre discussing an AI solution with a client. Theyre excited. Youre excited. The initial meetings go great. Lets bring in more stakeholders! they say. Soon, youve got the infrastructure team involved, five additional use cases under consideration, and another business executive at the table. The energy is palpable.Everyone sees the potential.And then the fighting starts. Or maybe not fighting exactly, but something more subtle. Resistance. Friction. Suddenly, a project that had everyone thrilled hit a wall. Why? Everyone was excited. Everyone saw the value. What changed?The answer might surprise you: its language.Not Python or Java or SQL but the everyday language we use to talk about AI. We have no shortage of technical challenges, but the most unexpected roadblocks to AI adoption often stem from a fundamental shift in how we need to work together. AI isnt just the new electricity of our age its forcing unprecedented collaboration between groups that previously operated in comfortable silos.When straightforward terms like performance, explainability, and risk carry such different meanings across teams, its no wonder some AI projects struggle to gain traction. These concepts form the foundation for discussing, evaluating, and implementing AI systems, but their meanings shift depending on whos using them. This linguistic flexibility isnt just a communication challenge its a window into deeper questions about professional identity, authority, and the changing nature of expertise in an AI-augmented workplace.As we introduce increasingly complex technical terminology around AI, these fundamental translation gaps only widen, creating invisible barriers that technical solutions alone cannot address.Setting the StageWe have all heard AI is the new electricity, but what that comparison misses is that when electricity transformed manufacturing, it didnt just change how things were powered it fundamentally restructured how people worked together.The same thing is happening with AI but more broadly. Electricity mainly required engineers and operators to collaborate. AI? Its forcing everyone to work together in unprecedented ways.AI Enthusaism Slipping Away. Image generated in DALL-E by author.Data scientists need domain experts to understand the problems theyre solving. Business leaders need technical teams to understand the possibilities and limitations. Front-line workers need to collaborate with both groups to ensure solutions work in the real world.And heres the kicker none of these groups are particularly good at talking to each other. Not because they dont want to, but because theyve never had to at least not at this depth.When Silos CrumbleThink about traditional technology implementations. You had clear handoffs: Business teams defined requirements, technical teams built solutions, and users learned to adapt. Everyone stayed in their lane and spoke their own language, and things mostly worked out.AI doesnt play that game.When data scientists build a model, they need to understand the business context not just surface-level requirements. When business teams deploy AI solutions, they need to understand more than just features and benefits they need to grasp concepts like model drift and edge cases. And users? Theyre not just learning new interfaces; theyre learning to collaborate with AI systems in ways that fundamentally change how they work.This isnt just cross-functional collaboration its forced interdependence. And its causing friction in unexpected places.LendAssist: Illustrative ExampleLets introduce LendAssist, an LLM-based mortgage lending assistant that we will use to illustrate this new reality.On paper, its straightforward. An AI system designed to streamline mortgage lending decisions, reduce processing time, and improve accuracy. LendAssists struggles highlight a critical challenge in AI adoption: seemingly straightforward terms can have radically different meanings for different stakeholders, leading to miscommunication and misunderstanding.What constitutes performance for a data scientist might be completely different for a data scientist building the product, the loan officer working with the product, or a customer interacting with the product.Similarly, explainability can have varying levels of depth and complexity depending on the audience.And risk can encompass a variety of issues and concerns, from technical failures to ethical dilemmas and job displacement.In the following sections, well explore these three key areas where language barriers arise.Expertise Paradox in AI AdoptionBefore we dive into specific challenges with LendAssist, lets discuss the expertise paradox, a fundamental tension that underlies them all.When LendAssist was first introduced, something unexpected happened. The most resistance didnt come from technophobes or change-resistant employees it came from the experienced loan officers and underwriters. The experts whose knowledge the system was designed to augment became its biggest skeptics.Why? The rapid rise of AI presents a unique challenge for experts in traditional fields. Its like suddenly finding yourself in a world where the games rules have changed, and your hard-earned expertise might not translate as seamlessly as youd hoped.This expertise paradox is a psychological and organizational hurdle that often gets overlooked in the excitement of AI adoption. Traditional tech leaders feel threatened by the need to start over as learners. Subject matter experts struggling with AI systems that challenge their domain expertise. There is a tension between deep knowledge of traditional systems and the need to adapt to AI-driven approaches.Organizations often face a delicate balancing act. They need to leverage their existing experts valuable experience while embracing AIs transformative potential. This creates tension and uncertainty as teams grapple with integrating traditional knowledge with AI capabilities.Through my work with AI implementations, Ive noticed a consistent pattern in how experts respond to this challenge. It typically manifests as three competing pressures Ive started mapping out to help teams understand whats happening.Maintaining Credibility I still know what Im doingExperts feel intense pressure to demonstrate that their knowledge remains relevant and valuable. Ive watched seasoned loan officers, for instance, struggle to show how their years of experience still matter when an AI system seems to make decisions in milliseconds.Embracing Change: I need to adapt to AIAt the same time, these experts recognize they need to evolve. This isnt just about learning new tools its about fundamentally rethinking how they apply their expertise. Ive seen loan officers transform from decision-makers to decision interpreters, but this shift rarely comes easily.Preserving Value: My experience mattersPerhaps most importantly, experts need to find ways to show how their experience enhances AI capabilities rather than being replaced by them. The most successful transitions Ive observed happen when experts can clearly see how their knowledge makes the AI better, not obsolete.The key to successful AI adoption is finding a balance between these three corners. Experts need to acknowledge the limitations of their existing knowledge, embrace the learning process, and find ways to leverage AI to enhance their expertise rather than viewing it as a threat.Despite these challenges, there are inspiring examples of experts successfully navigating the expertise paradox. These individuals embrace AI as a tool to augment their expertise and guide others in adapting to AI-driven approaches.GenAI Rollouts by Maturity. (McKinsey, 2025)This could explain a puzzling trend in AI adoption. A McKinsey survey completed in November 2024 and published in January 2025 and discussed in Superagency in the Workplace: Empowering people to unlock AIs full potential found that while one-quarter of executives have defined a GenAI roadmap, just over half remain stuck in the draft being refined stage.The technical capabilities exist, but organizations struggle with the human side of implementation. As technology continues evolving at breakneck speed, roadmaps must be built to evolve but we should recognize that many of the barriers arent technical at all.The invisible psychological and organizational traps repeatedly derail even the most promising AI initiatives.Performance A Multifaceted ChallengeThe data science team is ecstatic. LendAssists new fraud detection model boasts a 98% accuracy rate in their meticulously crafted testing environment. Champagne corks pop, high-fives are exchanged, and LinkedIn posts are drafted. But the celebration is short-lived. The operations team pushes back, overwhelmed by a 30% increase in false positives that clog their workflows.Meanwhile, the IT infrastructure team grapples with the models insatiable appetite for computing resources.And the business leaders, well, theyre left wondering why those key performance indicators (KPIs) havent budged an inch.Welcome to the performance paradox of AI adoption, where impressive technical achievements often clash with the messy realities of real-world implementation.Performance in AI is a chameleon, adapting its meaning depending on whos using the word. To truly understand this multifaceted challenge, we need to dissect performance through the lens of different stakeholders:Business Performance: The language of executives and shareholders focuses on the bottom line. Does LendAssist increase revenue? Does it reduce costs? Does it improve customer satisfaction and retention? Does it boost market share?Technical Performance: This is the domain of data scientists and engineers who are focused on metrics and algorithms. How accurate is LendAssists risk assessment model? Whats its precision and recall? How does it compare to traditional credit scoring methods regarding AUC and F1-score?Operational Performance: This is the realm of IT and operations teams concerned with utilization, efficiency, and scalability. How fast does LendAssist process loan applications? How much computing power does it consume? Can it handle peak loads without crashing? How easily does it integrate with existing systems?Human Performance: This is the often-overlooked dimension, focusing on the impact of AI on human workers. Does LendAssist make loan officers more productive? Does it reduce errors and improve decision-making? Does it enhance job satisfaction or create anxiety and resistance?But performance challenges are just the beginning.When different groups cant even agree on what good performance means, how do they explain their decisions to each other or, more importantly, customers?This brings us to an even thornier challenge: the crisis of explainability.Explainability The Black Box DilemmaA loan officer sits across from a client whos just been denied a mortgage by LendAssist. The client, understandably bewildered, asks, Why? The loan officer, with 20 years of experience explaining such decisions, finds herself staring blankly at the screen, unable to provide a clear answer. This isnt just about a declined mortgage its about a fundamental shift in professional authority, a moment where human expertise collides with the opacity of AI.Explainable AI (XAI) is no longer a luxury; its required to maintain trust, ensure responsible AI development, and navigate the evolving landscape of professional expertise.However, explainability itself has layers of understanding for different stakeholders, too.Technical Explainability Challenge: Our model shows high feature importance for these variables This might satisfy data scientists, but it leaves business users and clients in the dark. How does LendAssists technical team explain the models risk assessment to the data science team in a technically sound and understandable way?Process Explainability Challenge: But how does this translate to our existing underwriting workflow? Integrating AI into established processes requires explaining how it interacts with human decision-making. How does the data science team explain LendAssists integration into the loan approval process to the loan officers and underwriters, clarifying how it augments their existing expertise?Decision Explainability Challenge: How do we explain this to the customer? Building trust with clients requires clear, understandable explanations of AI-driven decisions. How do loan officers explain LendAssists loan denial decision to the client in a transparent and empathetic way without resorting to technical jargon?Impact Explainability Challenge: What does this mean for our business and regulatory compliance? Understanding the broader implications of AI decisions is crucial for responsible adoption. How do executives explain LendAssists impact on loan origination volume, risk mitigation, and compliance to stakeholders and regulators in an informative and persuasive way?Explainability isnt just about understanding its about authority.When professionals cant explain why decisions are made in their own domain, they lose not just control but their role as knowledge authorities. This can lead to resistance, fear of obsolescence, and difficulty integrating AI into existing workflows.Risk Navigating UncertaintyThe CTO champions LendAssist as the future of lending, painting a picture of streamlined workflows and data-driven decisions.The compliance team, however, sees looming regulatory disasters haunted by visions of biased algorithms and data breaches.Middle managers envision organizational chaos, with confused employees and disrupted workflows.Loan officers on the front lines of client interaction fear professional extinction and are replaced by an emotionless algorithm that spits out loan approvals and denials with cold, hard efficiency.It has the same technology but radically different risk landscapes.However, these surface-level conflicts mask a deeper pattern that reveals how organizations and individuals process the fundamental changes AI brings.Hidden Psychology of Risk When Talking about AIWe can break down this complex risk perception into four distinct levels:Level 1: What if it doesnt work? (Technical Risk) This is the most immediate and obvious concern. Will LendAssists AI models be accurate and reliable? Will the system be secure against cyberattacks? Will it comply with relevant regulations? But beneath these technical anxieties lies a deeper fear: losing control over familiar processes. When compliance officers obsess over LendAssists error rates, they often express anxiety about shifting from rule-based to probability-based decision-making. Theyre grappling with the uncertainty inherent in AI systems, where outcomes arent always predictable or easily explained.Level 2: What if it works too well? (Operational Risk) This is where things get interesting. As AI proves its capabilities, concerns shift from technical failures to operational disruptions. How will LendAssist impact the daily work of loan officers and underwriters? Will it disrupt existing processes and create confusion? Will it lead to job losses? But the real fear here is more personal: Will AI erode the value of human skills and experience? When loan officers worry about LendAssist processing applications too quickly, theyre asking, Will speed make my experience irrelevant? Theyre grappling with the potential for AI to diminish their role and authority in the lending process.Level 3: What if it works differently than we expect? (Strategic Risk) This level delves into the broader implications of AI adoption. Will LendAssist have unintended consequences? Will it disrupt the competitive landscape? Will it create new ethical dilemmas? But the underlying fear is about professional identity. When managers resist LendAssists recommendations, they often protect their identity as decision-makers more than questioning the AIs judgment. Theyre grappling with the potential for AI to redefine their roles and responsibilities, challenging their authority and expertise.Level 4: What if it changes who we are? (Identity Risk) This is the deepest and most existential level of risk perception. Will LendAssist fundamentally change how we work and interact with each other? Will it alter our understanding of expertise and professional identity? Will it reshape our values and beliefs about the role of technology in our lives? This is where the fear of obsolescence truly takes hold. When senior underwriters label LendAssist too risky, theyre expressing fear about transitioning from decision-makers to decision-validators. Theyre grappling with the potential for AI to transform their sense of self-worth and professional purpose.How technical and identity risks become intertwined makes AI risk assessment particularly challenging. When a loan officer says, LendAssists risk models arent reliable enough, they might be expressing fear of losing their ability to make judgment calls or anxiety about their role in the organization changing.The more organizations focus on addressing technical risks, the more they might inadvertently amplify identity risks by suggesting that human judgment is secondary to AI capabilities. As AI systems like LendAssist become more capable, they dont just present technical risks they force us to reconsider what it means to be an expert in an AI-augmented world.These layered challenges might seem insurmountable when viewed through a purely technical lens. After all, how do you solve a technical problem when the real issue lies in professional identity? How do you address performance concerns when different stakeholders define success in fundamentally different ways?What Ive found is that acknowledging these language barriers is the first crucial step toward overcoming them. When we recognize that resistance to AI adoption often stems from communication gaps rather than technological limitations, we open up new paths forward.The Path Forward: A Practical PerspectiveOnce you recognize these language barriers, they become surprisingly manageable. Were not just dealing with technical challenges were dealing with translation challenges. We need to become multilingual in the different ways our stakeholders talk about and understand AI.The organizations Ive seen succeed with AI adoption arent just technically sophisticated theyre linguistically sophisticated.They create a shared vocabulary that respects different perspectives. They recognize expertise transitions as a core implementation part and build bridges between technical and professional languages. They value communication skills as much as technical skills.ConclusionThis isnt just another factor to consider in AI adoption its often the factor determining go or no go decisions.The good news? While technical challenges typically require significant resources, language barriers can be addressed through awareness and intentional communication. Were all figuring this out together, but recognizing how language shapes AI adoption has been one of the most potent insights. Its changing how I approach projects, how I work with stakeholders, and, most importantly, how I help organizations navigate the fundamental changes AI brings to professional expertise.The choice isnt between technical excellence and human understanding its about building bridges between them.And sometimes, those bridges start with something as simple as recognizing that we might mean different things when we say performance, explainability, or risk.Further reading and citationWhy AI Projects Fail and How They Can SucceedBy some estimates, more than 80 percent of AI projects fail. Thats twice the rate of failure of information technologywww.rand.orgKeep Your AI Projects on TrackAI-and especially its newest star, generative AI-is today a central theme in corporate boardrooms, leadershiphbr.orgSuperagency in the workplace: Empowering people to unlock AIs full potentialAlmost all companies invest in AI, but just 1% believe they are at maturity. Our new report looks at how AI is beingwww.mckinsey.comJoin thousands of data leaders on the AI newsletter. Join over 80,000 subscribers and keep up to date with the latest developments in AI. From research to projects and ideas. If you are building an AI startup, an AI-related product, or a service, we invite you to consider becoming asponsor. Published via Towards AI0 Comments ·0 Shares ·20 Views
-
TAI #141: Claude 3.7 Sonnet; Software Dev Focus in Anthropics First Thinking Modeltowardsai.netAuthor(s): Towards AI Editorial Team Originally published on Towards AI. What happened this week in AI by LouieAnthropic Claude 3.7 Sonnet reasoning model stole the show this week. This is partly due to how quickly you can test and see the models coding talent with native code rendering in Claudes Artefacts feature. This was also a positive week for open-source reasoning LLMs with Alibabas QwQ-Max-Preview now available and set for an imminent open-weights release. Meanwhile, Prime Intellect released SYNTHETIC-1 the largest open reasoning dataset yet, comprising 2 million verified math, coding, and science reasoning traces from DeepSeek-R1 alongside a 7B model fine-tuned on this data. This dataset will be valuable for fine-tuning reasoning models and customizing them to specific domains. Meanwhile, OpenAI disclosed that ChatGPT has hit 400 million weekly active users, which we calculate now covers 7.2% of global internet users!Anthropic Claude 3.7 Sonnets headline feature is its extended thinking mode, where the model now explicitly shows multi-step reasoning before finalizing answers. Anthropic noted that it focuses its reinforcement learning training on real-world code problems relative to math problems and competition code (a slight dig at OpenAIs o3 Codeforces focus here). This focus shows very impressive SWE-bench agentic coding capabilities while Maths benchmarks lag other leading reasoning models. Claude 3.7 Sonnet scores 62.3% without thinking mode on SWE (70.3% with a scaffold on a subset of problems), significantly ahead of OpenAI o1 at 48.9%, o3-mini high at 49.3% and in the ballpark of OpenAI o3s reported 71.7% score. On AIME MATH 2024, Claude 3.7 Sonnet scores 61.3% pass@1 (80.0% with parallel scaling), a big jump from Sonnet 3.5 News 16.0% but behind Grok-3s 83.9% (93.3% with parallel scaling) and OpenAI o3 at 96.7% score with parallel scaling.Looking at GPQA Diamond, Claude 3.7 Sonnet with Extended thinking achieves 78.2% pass @1 (or 84.8% with up to 64k tokens, including parallel scaling of multiple samples). This outperforms OpenAI o1 at 75.7% (78.3% with parallel scaling) and OpenAI o3-mini at 79.7%. However, Grok-3 thinking mode wins here with pass @1 80.2% (84.6% with parallel scaling), and OpenAIs unreleased o3 still leads at 87.7% with parallel scaling. In non-thinking modes, Claude 3.7 Sonnet scores 68.0% below Grok-3s non-thinking score of 75%, but outperforming Gemini-Pro 2.0 at 65% and OpenAI 4o at 51%.Claude 3.7 Sonnet keeps the same price as 3.5 even in thinking mode: $3 per million input tokens and $15 per million output tokens. This is a positive surprise given both OpenAI and Deepseek charge a large premium for their reasoning models which we think is justified by higher compute and memory usage and lower inference batch sizes possible when the average output generation length is longer (even with the same architecture). Via the API, Claude 3.7 Sonnet users also get extra flexibility to control the budget for thinking: you can tell Claude to think for no more than N tokens (up to 128k) allowing you to decide your own trade-offs between latency, cost, and capability.Anthropic recently revealed that 37% of Claude Chatbot queries are software-related and this doesnt even count the heavy use of Claude APIs for coding copilots and agents such as Cursor. Perhaps Anthropic is beginning to see this as a point of differentiation against OpenAI, Google Gemini, and xAI in any case beyond Claude 3.7s focused improvement on software-related benchmarks Anthropic also released its own coding agent. Claude Code is now in beta as a research preview. Designed to operate directly in the terminal, it integrates directly with developers workflows without additional servers or complex setups. Claude Code can edit files, fix bugs, execute tests, search through git history, resolve merge conflicts, and create commits all through natural language commands.Why should you care?As we noted last week reasoning models with test time compute scaling capability have become the new battleground for SOTA in LLMs. Now, Anthropic has also joined the party (following OpenAI, Deepseek, Alibaba, Google Deepmind, and xAI). Potentially in part due to this competition, Anthropic has also come with a more user-friendly approach both in its subscription chatbot, where 3.7s Thinking mode can simply be toggled (similar to Grok-3) and in its API where it provides the most flexibility yet for directly controlling thinking token usage and offers flat pricing vs. the base model. It will take some practice to test how well this thinking token control works and how much benefit you really get from pushing to the highest settings.On our side, the early testing of 3.7 shows some impressive capabilities, particularly in front-end code with mini apps and websites. We think this will only accelerate the momentum in LLM Native coding or what Andrej Karpathy calls vibes coding, where even experienced developers can quickly learn new software skills and languages top-down starting with natural language and an LLM-generated project. More on this soon, with the imminent release of our LLM native Python course for coding novices!Hottest News1. Anthropic Introduced Claude 3.7 Sonnet, Their Hybrid Reasoning ModelAnthropic announced Claude 3.7 Sonnet, its most intelligent and first hybrid reasoning model. It can produce near-instant responses or extended, step-by-step thinking that is visible to the user. API users also have fine-grained control over how long the model can think. Anthropic also introduced a limited research preview of Claude Code, a command-line tool for agentic coding.2. Prime Intellect Released SYNTHETIC-1, the Largest Open Reasoning DatasetPrime Intellect has introduced SYNTHETIC-1, an open-source dataset designed to provide verified reasoning traces in math, coding, and science. Built with the support of DeepSeek-R1, this dataset consists of 1.4 million structured tasks and verifiers. The objective of SYNTHETIC-1 is to improve reasoning models by supplying them with well-organized, reliable data, addressing the shortcomings of existing resources.3. Arc Institute Developed Evo 2, the Largest AI Model for BiologyArc Institute developed Evo 2, trained on the DNA of over 100,000 species across the entire tree of life. The model can write whole chromosomes and small genomes from scratch. It can also make sense of existing DNA, including hard-to-interpret non-coding gene variants linked to disease.4. Alibaba Unveils QwQ-Max-PreviewAlibaba launched QwQ-Max-Preview, a new reasoning model in the Qwen family of AI models. The model is built on the Qwen 2.5 Max and specializes in mathematics and coding-based tasks. The model is in its preview stage, and the company is expected to announce its full version soon.5. Open AI Introduced the SWE-Lancer BenchmarkSWE-Lancer introduces a benchmark with over 1,400 freelance software engineering tasks from Upwork, valued at $1 million. It evaluates model performance on tasks, ranging from $50 bug fixes to $32,000 feature implementations, through tests verified by engineers. Frontier models struggle with most tasks.6. Perplexity AI Open-Sourcing a Post-Trained DeepSeek-R1 With Censorship RemovedPerplexity AI introduced the R1 1776 model, a post-trained DeepSeek-R1 designed to eliminate Chinese Communist Party censorship while maintaining high reasoning abilities. Rigorous multilingual evaluations using human annotators and LLM judges confirmed that decensoring did not impact the models core reasoning capabilities. The model performed on par with the base R1 model across various sensitive topics.Five 5-minute reads/videos to keep you learning1. Andrej Karpathys Early Access Review of Grok 3In this article, Andrej Karpathy evaluated Grok 3, noting its strong performance in thinking tasks, comparable to OpenAIs models. Despite issues with humor and ethical sensitivity, it surpassed DeepSeek-R1 and Gemini 2.0 in some areas.2. DeepSeek vs. ChatGPT A Detailed Architectural and Functional BreakdownThis article compares two leading LLMs, ChatGPT and DeepSeek. It focuses on architectural design, training methodologies, performance, and limitations. While ChatGPT is more generic and can handle a broader variety of tasks, DeepSeek becomes a more feasible alternative for tightly focused applications.3. Build Your LLM Engineer Portfolio (Part 2): A 3-Month RoadmapThis step-by-step guide is designed to help you build, refine, and showcase a RAG portfolio to kickstart your career. It provides a comprehensive plan for your three-month journey toward creating an impressive RAG portfolio. Youll find essential preparation steps to establish a solid foundation for your projects, seven impactful projects that will enhance your expertise and help you stand out, and strategies for deploying and presenting your portfolio to achieve maximum exposure.4. 1 Billion ClassificationsThis blog explains how to calculate cost and latency for large-scale classification and embedding. It analyzes different model architectures, benchmarks costs across hardware choices and provides a clear framework for optimizing your own setup.5. Insights on Crosscoder Model DiffingCrosscoder-based model diffing is a promising method for isolating differences between two models with a single SAE training run. In this note, Anthropic discusses a few unexpected observations when applying this technique to real models. This will be helpful for researchers working actively in this space.Repositories & ToolsAibrix is an open-source initiative that provides building blocks to construct scalable GenAI inference infrastructure.FlashMLA is an MLA decoding kernel for Hopper GPUs optimized for variable-length sequence serving.Mastra is a Typescript framework that helps you build AI applications and features.Top Papers of The Week1. Accelerating Scientific Breakthroughs With an AI Co-ScientistThis paper introduces AI co-scientist, a multi-agent AI system built with Gemini 2.0. The agent acts as a virtual scientific collaborator to help scientists generate novel hypotheses and research proposals. It can potentially help accelerate the clock speed of scientific and biomedical discoveries.2. Qwen2.5-VL Technical ReportQwen2.5-VL advances visual recognition with enhanced object localization, robust document parsing, and long-video comprehension. It accurately extracts structured data and analyzes charts. Featuring dynamic resolution processing and Window Attention, Qwen2.5-VL reduces computational overhead.3. MoBA: Mixture of Block Attention for Long-Context LLMsThis paper introduces Mixture of Block Attention (MoBA), an innovative approach that applies the principles of Mixture of Experts (MoE) to the attention mechanism. This architecture demonstrates superior performance on long-context tasks while seamlessly transitioning between full and sparse attention, enhancing efficiency without compromising performance.4. Native Sparse Attention: Hardware-Aligned and Natively Trainable Sparse AttentionNSA, a Natively trainable Sparse Attention mechanism, improves efficiency in long-context modeling by integrating algorithmic innovations with hardware-aligned optimizations. It achieves substantial speedups and maintains model performance across benchmarks. NSAs dynamic hierarchical strategy combines coarse-grained token compression with fine-grained selection, excelling over Full Attention in 64k-length sequences during decoding, forward propagation, and backward propagation.5. CodeI/O: Condensing Reasoning Patterns via Code Input-Output PredictionCodeI/O enhances reasoning in language models by transforming code into input-output prediction formats, exposing models to reasoning patterns like logic flow planning and decision tree traversal. This method improves performance across multiple reasoning tasks.Quick Links1. OpenAIs COO recently shared some key updates in a post on X. ChatGPT has surpassed 400 million weekly active users. He also hinted at the upcoming GPT-4.5 and GPT-5 releases for both the chat interface and the API. Additionally, free users will have unlimited access to GPT-5 and enhanced agent capabilities.2. Meta announces LlamaCon, its first generative AI dev conference focusing on open-source AI developments. Despite competitive pressures from DeepSeek, Meta plans to release new Llama models with reasoning and multimodal capabilities.3. Together AI secured $305 million in Series B funding, led by General Catalyst and joined by notable investors like NVIDIA and Salesforce Ventures. This investment accelerates the companys AI Acceleration Cloud for open-source and enterprise AI application development.Whos Hiring in AIGen AI Developer @Syncreon Consulting (Toronto, Canada)Junior Data & AI Engineer Emirati @Ghobash Group (Dubai, UAE)AI Software Engineer (Mid/Senior) @SmartDev (Hanoi, Vietnam)Global Data Office Intern Summer 2025 @Visa (CA, USA)PDM Engineering Data Administrator @Bosch Group (Burnsville, MN, USA)Software Engineer NLU @Cognigy (Remote)Interested in sharing a job opportunity here? Contact [emailprotected].Think a friend would enjoy this too? Share the newsletter and let them join the conversation.Join thousands of data leaders on the AI newsletter. Join over 80,000 subscribers and keep up to date with the latest developments in AI. From research to projects and ideas. If you are building an AI startup, an AI-related product, or a service, we invite you to consider becoming asponsor. Published via Towards AI0 Comments ·0 Shares ·16 Views
-
Agentic RAG: Mastering Document Retrieval with CrewAI, DeepSeek, and Streamlittowardsai.netAgentic RAG: Mastering Document Retrieval with CrewAI, DeepSeek, and Streamlit 0 like February 25, 2025Share this postLast Updated on February 25, 2025 by Editorial TeamAuthor(s): Vikram Bhat Originally published on Towards AI. This member-only story is on us. Upgrade to access all of Medium.Image generated using napkin.aiIn my previous blog, I explored building a Retrieval-Augmented Generation (RAG) chatbot using DeepSeek and Ollama for privacy-focused document interactions on a local machine here. Now, Im elevating that concept with an Agentic RAG approach powered by CrewAI. This project harnesses CrewAIs multi-agent framework to process documents locally, delivering precise retrieval and concise responses without compromising data security. Running entirely on your local machine with Ollama and DeepSeek for robust language processing, it integrates seamlessly with CrewAI and features an intuitive Streamlit UI. Lets dive into building this secure, agent-driven RAG chatbot step-by-step.Building a RAG Chatbot with Context-Aware Responses Locally using DeepSeek, LangChain, and Streamlitgenerativeai.pubGitHub Repo: The complete code is available on GitHub. Follow this blog for a detailed implementation guide.To set up and run this Agentic RAG locally, ensure you have the following setup:Python 3.10 or Higher Install Python from python.org. Version 3.10 or newer is required for compatibility with the libraries used in this project.Required Libraries Install the necessary dependencies using the following command:pip install streamlit crewai langchain pypdf faiss-cpu sentence-transformersThese include Streamlit for creating an interactive user interface, CrewAI for orchestrating the multi-agent Read the full blog for free on Medium.Join thousands of data leaders on the AI newsletter. Join over 80,000 subscribers and keep up to date with the latest developments in AI. From research to projects and ideas. If you are building an AI startup, an AI-related product, or a service, we invite you to consider becoming asponsor. Published via Towards AITowards AI - Medium Share this post0 Comments ·0 Shares ·15 Views
-
How AI is Revolutionizing Education: Key Benefits and Trendstowardsai.netLatestMachine LearningHow AI is Revolutionizing Education: Key Benefits and Trends 0 like February 24, 2025Share this postAuthor(s): Karen Cartar Originally published on Towards AI. How AI is Revolutionizing Education: Key Benefits and TrendsThe education sector faces a transformation through Artificial Intelligence (AI) which actively reshapes various industries across the world. The education sector undergoes transformation through AI because it implements both customized teaching approaches and automated operational tasks that modify student learning methods and instructor instruction styles.The education field undergoes significant transformation through AI-powered technologies like machine learning and natural language processing and predictive analytics which active learning spaces from standard classrooms. The following article investigates AI changes in education by examining both its significant advantages as well as upcoming trends in learning modifications.Key Benefits of AI in Education1. Personalized LearningAI applies personalized educational experiences to individual students as its main educational benefit. A conventional teaching framework applies identical instructional approaches across all students yet many students finish ahead while others face difficulties. AI powered apps created by AI App Development Company in IndiaExamples:Adaptive learning platforms: The education technology known as adaptive learning platforms makes use of AI algorithms to adapt exercise complexity according to how students respond to them. Educational content through DreamBox and Knewton automatically shifts according to how each learner progresses.Intelligent tutoring systems: Through AI tutors students get personalized support and immediate feedback which enables individualized instruction beyond formal lessons.2. Enhanced AccessibilityEducation becomes more accessible through AI because the technology develops resources which accommodate students who face disabilities along with language differences. AI applications created by Software Development Company in IndiaExamples:Speech-to-text applications: The speech-to-text applications deliver live lecture transcription that enables hearing-impaired students to track classroom discussions effortlessly.AI-powered language translation: Language translation through artificial intelligence enables students to understand their coursework through their mother language thanks to platforms such as Duolingo and Google Translate thus helping students understand better while taking part in class activities.Text-to-speech tools: The text-to-speech tools NaturalReader and Microsofts Immersive Reader turn educational materials into audio forms which benefit visually impaired students when accessing their educational content.AI-driven captioning: AI systems use automated caption generation to provide accessibility to online lectures and videos which benefits all learners especially those who have hearing difficulties.3. Efficient Administrative ProcessesSchool administrators achieve enhanced teaching efficiency through AI because it eliminates tedious paperwork processes thus enabling educators to dedicate more time to teaching activities. AI apps that operate repetitive administrative work to improve educational institutions operational efficiency along with their resource management.Examples:Automated grading systems: AI-driven grading software evaluates tests of all kinds including multiple-choice answers along with rough draft submissions to programming work to create faster and immediate student feedback.Chatbots for student support: The implementation of AI-powered chatbots at universities enables the processing of standard student inquiries about course information along with deadlines and academic regulations which shortens response periods while enhancing student contentment levels.AI-powered scheduling assistants: The AI scheduling platform assists educational institutions to run schedulers and distribute classrooms alongside faculty assignments while it maximizes resource allocation and minimizes scheduling conflicts.Attendance tracking and monitoring: An AI system with facial recognition technology together with RFID-based attendance systems performs automated roll call operations to achieve accurate student attendance tracking.4. Data-Driven InsightsEducational institutions can Hire Software Developers in IndiaExamples:Predictive analytics: Through predictive analytics education institutions receive warning about students who might face risk of withdrawal from school which permits personalized support.Classroom performance analysis: The AI systems measure classroom performance through student engagement data which helps teachers develop better teaching approaches.Curriculum improvement: Through AI-driven feedback analysis educational institutions can improve curriculum content by spotting course learning gaps which enables them to enhance the educational program effectiveness.Student learning behavior analysis: AI tools evaluate student learning material engagement to find spots where supplementary learning resources and substitute educational approaches would prove beneficial.Emerging Trends in AI-Driven Education1. Intelligent Tutoring SystemsStudents using AI-based tutoring programs benefit from individualized instruction that happens through interactive educational sessions. Complex subjects require guidance from these systems that enable learners to understand concepts according to their individual learning pace.Examples:AI chatbots: AI chatbots through Squirrel AI and Carnegie Learnings MATHia system provide instant tutoring services to students and tailor lesson plans according to their acquired knowledge.Gamified learning: The educational technique of gamification produces better retention and motivation through adaptive challenges and quizzes and reward systems as well as the use of AI-driven gamification technologies such as Duolingo and Kahoot!.Real-time adaptive feedback: The AI tutors analyze student development to give immediate performance assessments that direct students to specific areas requiring support.2. AI in Assessment and EvaluationThe use of artificial intelligence enhances performance quality of student examination assessment systems which supports teachers by reducing their work while preserving academic fairness and maintaining testing objectivity.Examples:Writing tools like Grammarly and Turnitin powered by AI evaluate student work for grammar rules and document organization alongside originality while offering quick feedback that strengthens their composition abilities.Academic integrity through proper screening takes place when AI systems running plagiarism checkers like Copyscape and Unicheck discover matching student work.AI systems that evaluate student responses offer instantaneous recommendations for supplementary study content which matches specific areas where students have performed poorly.ProctorU and similar AI-based remote proctoring systems monitor online exams to detect suspicious activities thus maintaining academic honesty.3. Virtual Reality (VR) and Augmented Reality (AR) IntegrationAI-powered VR and AR technologies generate educational environments that boost both student devotion and their understanding of subjects.Examples:Virtual science labs: The combination of artificial intelligence with simulations leads students to perform experiments virtually so science learning becomes more interactive while removing potential risks.Historical simulations: AI-enhanced virtual reality provides students with virtual access to explore historical events and ancient civilizations at both a historical and present time standpoint.Medical training simulations: The combination of artificial intelligence with virtual reality serves medical students to practice surgical procedures as well as clinical techniques in secure and authentic circumstances.Mathematics & engineering visualization: Artificial Intelligence through AR technology enables students to render complex mathematical equations as well as engineering prototypes so they can solve problems more effectively.4. AI for Teacher SupportAI technology supports educational needs by enhancing lesson quality together with assignment evaluation and classroom behavioral analysis which results in superior teaching conditions.Examples:Lesson planning tools: Computers available in lesson planning analyze curriculum directives along with educational data from students to match teaching approaches with ideal study materials.Student engagement tracking: The technology employs AI-powered cameras together with sensors to read student body signals and facial cues which determine their levels of engagement and detect periods when students lose focus.Automated grading and feedback systems: AI tools implement automated grading and feedback procedures which help teachers focus on advanced instructional activities because assignments receive automated evaluations followed by constructive assessments.AI-driven professional development: AI enables teachers to access customized training recommendations through its system as it helps educators learn about contemporary teaching techniques.ConclusionArtificial intelligence currently transforms education through enhanced personalized learning experiences while improving access options and simplifying administrative management processes. The deployment of AI systems requires proper ethical management while guaranteeing equal access for students under constant human supervision. Businesses Hire Mobile App Developers in IndiaJoin thousands of data leaders on the AI newsletter. Join over 80,000 subscribers and keep up to date with the latest developments in AI. From research to projects and ideas. If you are building an AI startup, an AI-related product, or a service, we invite you to consider becoming asponsor. Published via Towards AITowards AI - Medium Share this post0 Comments ·0 Shares ·72 Views
-
Introduction To Federated Learningtowardsai.netLatestMachine LearningIntroduction To Federated Learning 0 like February 24, 2025Share this postLast Updated on February 24, 2025 by Editorial TeamAuthor(s): William Lindskog Originally published on Towards AI. Brought to you by Flower Labs.Breaking Down AI: What Makes It Work?Artificial Intelligence (AI) is transforming everything from self-driving cars to personalized medicine to financial fraud detection. But what exactly makes AI work?Practically, AI consists of 3 fundamental components:AI Models The brains of AI. Models process data and make decisions, like recognizing faces in photos or predicting stock market trends. Most modern AI models, such as neural networks, are inspired by the way the human brain works where information flows through layers of interconnected nodes, leading to a decision.Hardware The muscle behind AI. If youve heard of GPUs (Graphics Processing Units), you already know why they matter. GPUs are highly efficient at handling AI computations, enabling faster training and inference. This specialized hardware is why AI has accelerated so rapidly in the last decade.Data The fuel of AI. The more quality data an AI model has, the better it performs. Everything from search engines to chatbots to healthcare AI relies on vast amounts of high-quality data to learn and improve.But heres the problem: Data has become AIs biggest bottleneck.Traditional recipe for breakthroughs in AI. We are running out of publicly available data.The AI Data Problem: Why Traditional Approaches Dont ScaleAI models dont improve on their own. They need continuous exposure to diverse, high-quality data to become more accurate, reliable, and useful. The challenge? Access to public data is shrinking, not growing [1]. Many industries e.g. healthcare, finance, automotive, and beyond have the data that could supercharge AI, but its trapped behind barriers that make large-scale AI training incredibly difficult. [2]The Best Data Is PrivateThe most valuable datasets e.g. medical records, financial transactions, personal conversations, and real-world driving data,cant simply be shared or copied without violating privacy laws and ethical standards.Hospitals cant share patient records with external AI labs due to HIPAA and GDPR [3,4]. These silos hold the potential to train AIs that possess capabilities beyond what is currently possible. While there are efforts to make data publicly available, it is an insignificant amount to what is kept within organizations.Banks cant expose customer transactions to train better fraud detection models [5]. Collaborative efforts would make enable better detection of fraudulent transactions and anti-money laundering. Yet, today it can be challenging detecting these with data from entities within a larger enterprise.Users dont want their private messages collected for improving chatbots. This is quite obvious, but we do want personalized AIs helping us check for grammatical errors and typos.As a result, many AI models end up being trained on public or outdated datasets that dont reflect real-world scenarios. The AI remains detached from the actual environment where its supposed to operate.Data Is Scattered Across the WorldEven when data can be used, its rarely in one place. Instead, its fragmented across multiple devices, companies, or institutions. Creating a centralized dataset is cumbersome. Larger platforms and organizations can benefit from big consumer groups and in-house datasets, but even data transfer between in-house entities/units is not always straightforward. Smaller research groups often need to resort to publicly available data or construct their own. While this is possible, it is not only time-consuming but costly.Medical AI needs patient data from multiple hospitals, but each hospital has its own isolated dataset. Furthermore, making this sort of data public often means anonymizing the data, which complicates matters when attempting to develop personalized AI models.Self-driving cars collect sensor data, but who actually owns the data that originates in a vehicle? For example, a company like Volvo might have its own fleet of vehicles but is running 3rd party software to power self-driving car capabilities. Yet, this software is running on hardware produced by another company which actually stores that data. To top it off, the operating system might be something like Android, so at what company does the data actually reside? This is where collaboration is needed.Large language models (LLMs) improve with real user interactions, but personal conversations are stored on individual phones. Thus, in order to develop their capabilities, they need more data from users phone. Nevertheless, centralizing this data is out of the question. People are used to 2-way encryption techniques and do not want their messages to be stored elsewhere than their phone.Centralizing this data into one place is often impossible due to logistics, security, and trust issues.AI Needs More Data But Its Getting LessThere is a clear correlation between the more quality data an AI gets, the better the performance [6]. Therefore, for anyone interested in seeing AI get better, we need to ensure that it can access at least knowledge from more data. Nevertheless, the amount of publicly available data is decreasing rapidly [1]. This leads to a paradox:AI models require massive, real-world datasets to improve. You might have heard that the latests LLMs are trained on almost all text on the internet. While this is up for discussion, there is no doubt that they train and fine-tune their models on enormous amounts of data.The best datasets are locked in silos. While there are several reasons for this e.g. regulations or corporate policies, an argument can be made for collaboration being beneficial. Take healthcare as an example. Suppose there are healthcare clinics fragmented across regions. Privacy laws hinder them from sharing patient data, but there might be only one clinic large enough to have enough data to train a somewhat accurate cancer predictor. The smaller clinics dont have enough quality data and would benefit hugely from knowledge from the larger clinic. Vice versa, the larger clinic would also benefit from more data from all the different clinics but privacy laws put an end to this. While the reason for the law might at one point been reasonable, the current outcome is not.So, whats the solution? How can AI train on more data while respecting privacy and security?This is where Federated Learning [7] (FL) changes the game.FL addresses a fundamental limitation in AI development: access to high-quality, real-world data is diminishing, yet AI models require more data than ever to improve. Current methods rely on centralizing datasets for training, but as we have established, this approach is increasingly infeasible due to logistical barriers. The question, then, is how do we enable AI to improve without direct access to more data?A Paradigm Shift in AI TrainingFL presents a novel approach: instead of bringing the data to the model, we bring the model to the data. This decentralized method allows multiple entities, whether they be hospitals, financial institutions, or individual devices, to collaboratively train an AI model without sharing raw data.FL is built upon a simple yet powerful mechanism:A global AI model is initialized and distributed across decentralized participants (clients). Lets take next word prediction for texting on smartphones. The task is arbitrary but something that many people might be familiar with.Each participant trains the model locally using its own private dataset. This means that the AI used to predict the next word on your phone will train itself on the messages that you type. Now, important, the messages themselves are still kept on your phone and not sent anywhere. The AI learns from the messages but doesnt in itself store a message that you have sent.Instead of sending raw data to a central server, only model updates (weight adjustments, gradients, or encrypted parameters) are shared. This means that your texts/messages are not shared with other devices and the intrinsic structure and build of the AI on your phone is shared, not the data.A central coordinator aggregates these updates to improve the global model while ensuring that no single participants data is exposed. Thus, we still learn from millions, maybe even billions of devices, but the data never leaves, in this case, the phone.This cyclical process repeats over multiple training rounds, leading to an AI model that benefits from a diverse, distributed dataset without compromising privacy or security.An initial model is hosted on the cloud (1), it is sent out as copies of itself to, in this case, different smartphones (2), each copy of the model is trained on data from the respective smartphones which results in slightly different models as they have been exposed to different texts/messages (3), the model updates are sent back to the server not the texts themselves (4), where they are aggregated to form a better AI (5).Theoretical Foundation of Federated LearningFL builds upon several well-established principles in distributed optimization and privacy-preserving machine learning:Decentralized Stochastic Gradient Descent (SGD): Instead of computing gradients from a centralized dataset, gradients are computed locally and aggregated at the server [7].Secure Aggregation: Techniques such as differential privacy [8] and homomorphic encryption [9] ensure that individual updates remain confidential while still contributing to model improvement.Statistical Heterogeneity Management: Unlike centralized training, where datasets are curated for uniformity, FL must account for non-IID (independent and identically distributed) data, meaning different clients may have vastly different distributions. Take the case of driving assisting capabilities in automotive. Suppose you have 2 drivers: the aggressive one (1) who likes to hit the gas and see the car take off quickly, and then the more environmental friendly one (2) who cares more about getting to the final destination having save fuel. If an AI trains on the data from these 2 people in a federated fashion, the end model might be okay but just okay. Seeing that their behaviors vary greatly, it might have landed somewhere just right in between, which doesnt serve either one perfectly. Thus, FL needs to be able to handle this, and current state-of-the-art frameworks can. [1012]This approach allows AI to scale beyond traditional limitations, accessing knowledge from previously inaccessible, private, or fragmented datasets. We are already seeing FL being deployed at mass scale in various industries. Frameworks like Flower [10] enable experimentation with your FL solutions and can be used to deploy for real-world applications.Applications of Federated Learning Across IndustriesHealthcare: Preserving Patient Privacy While Advancing AIThe potential for AI in healthcare is undeniable: AI models can assist in diagnosing diseases, predicting patient deterioration, and optimizing treatment plans. However, strict regulations such as HIPAA (U.S.), GDPR (EU), and local data protection laws prevent hospitals from sharing sensitive patient records. This is understandable as patients do not e.g. want images of their bodies or blood values being distributed across the globe. Moreover, such sensitive data can be used in harmful ways, thus it should stay protected.Traditional AI development requires large, diverse datasets, but hospitals cannot freely exchange patient data across institutions or regions. Federated Learning enables collaborative AI training across hospitals without exposing patient records. Each hospital locally trains the AI model using its own dataset, and only model updates are shared for aggregation. A cancer detection model could be trained across multiple hospitals worldwide, improving diagnostic accuracy for rare conditions without violating patient privacy laws. [1315]Other industries are also adopting FL: automotive [16], finance [17], telecommunications [18] and more. The reason being:More data = Better AIThe Future of AI is FederatedFederated Learning represents a fundamental shift in how AI models are trained. By enabling collaborative, privacy-preserving AI, FL bridges the gap between the need for more data and the constraints of modern privacy and security regulations.Key TakeawaysAI requires continuous exposure to diverse, high-quality data but traditional data collection is becoming infeasible.Federated Learning enables AI models to learn from decentralized data sources while preserving privacy.Industries such as healthcare, automotive, and finance are already adopting FL to unlock new AI capabilities.The future of AI will depend on distributed, privacy-conscious training methodologies and FL is leading the way. Want to explore Federated Learning further? Start building with Flower, the open-source framework for privacy-preserving AI.References[1] Villalobos, Pablo, et al. Will we run out of data? Limits of LLM scaling based on human-generated data. arXiv preprint arXiv:2211.04325 (2022).[2] Karimireddy, Sai Praneeth, et al. Breaking the centralized barrier for cross-device federated learning. Advances in Neural Information Processing Systems 34 (2021): 2866328676.[3] Brauneck, Alissa, et al. Federated machine learning, privacy-enhancing technologies, and data protection laws in medical research: scoping review. Journal of medical Internet research 25 (2023): e41588.[4] Loftus, Tyler J., et al. Federated learning for preserving data privacy in collaborative healthcare research. Digital Health 8 (2022): 20552076221134455.[5] Long, Guodong, et al. Federated learning for open banking. Federated learning: privacy and incentive. Cham: Springer International Publishing, 2020. 240254.[6] Budach, Lukas, et al. The effects of data quality on machine learning performance. arXiv preprint arXiv:2207.14529 (2022).[7] Konen, Jakub, et al. Federated learning: Strategies for improving communication efficiency. arXiv preprint arXiv:1610.05492 (2016).[8] Dwork, Cynthia. Differential privacy. International colloquium on automata, languages, and programming. Berlin, Heidelberg: Springer Berlin Heidelberg, 2006.[9] Gentry, Craig. Fully homomorphic encryption using ideal lattices. Proceedings of the forty-first annual ACM symposium on Theory of computing. 2009.[10] Beutel, Daniel J., et al. Flower: A friendly federated learning research framework. arXiv preprint arXiv:2007.14390 (2020).[11] Zhu, Hangyu, et al. Federated learning on non-IID data: A survey. Neurocomputing 465 (2021): 371390.[12] Li, Qinbin, et al. Federated learning on non-iid data silos: An experimental study. 2022 IEEE 38th international conference on data engineering (ICDE). IEEE, 2022.[13] Qayyum, Adnan, et al. Collaborative federated learning for healthcare: Multi-modal covid-19 diagnosis at the edge. IEEE Open Journal of the Computer Society 3 (2022): 172184.[14] Chaddad, Ahmad, Yihang Wu, and Christian Desrosiers. Federated learning for healthcare applications. IEEE internet of things journal 11.5 (2023): 73397358.[15] Antunes, Rodolfo Stoffel, et al. Federated learning for healthcare: Systematic review and architecture proposal. ACM Transactions on Intelligent Systems and Technology (TIST) 13.4 (2022): 123.[16] Lindskog, William, Valentin Spannagl, and Christian Prehofer. Federated learning for drowsiness detection in connected vehicles. International Conference on Intelligent Transport Systems. Cham: Springer Nature Switzerland, 2023.[17] Wen, Jie, et al. A survey on federated learning: challenges and applications. International Journal of Machine Learning and Cybernetics 14.2 (2023): 513535.[18] Lee, Joohyung, et al. Federated learning-empowered mobile network management for 5G and beyond networks: From access to core. IEEE Communications Surveys & Tutorials 26.3 (2024): 21762212.Join thousands of data leaders on the AI newsletter. Join over 80,000 subscribers and keep up to date with the latest developments in AI. From research to projects and ideas. If you are building an AI startup, an AI-related product, or a service, we invite you to consider becoming asponsor. Published via Towards AITowards AI - Medium Share this post0 Comments ·0 Shares ·68 Views
-
How to Fine-tune Time Series Forecasting Models for Crypto Coinstowardsai.netLatestMachine LearningHow to Fine-tune Time Series Forecasting Models for Crypto Coins 0 like February 24, 2025Share this postAuthor(s): Eivind Kjosbakken Originally published on Towards AI. Predict the Future Value of Crypto Coins with Fine-tuned Time Series Forecasting ModelsThis member-only story is on us. Upgrade to access all of Medium.In this article, I will show you how you can use time series forecasting to predict the future price of a cryptocurrency coin. I will then move into the more advanced topic of fine-tuning the forecasting model for a specific coin in order to improve prediction quality.Predict future cryptocurrency prices using fine-tuned time series forecasting models. Image by ChatGPT. Downloading data Making the training and evaluation dataset Setting up Chronos time-series forecasting model Evaluate the base Chronos model Fine-tune Chronos model Evaluate fine-tuned model ConclusionNote that the contents of this article are not financial advice, and you should use the code at your own risk.If you want to keep it simple, you can download this Bitcoin dataset online, which I will use for the rest of the article. If you use this data, you can jump directly to the next section. However, if you want to download your own data, you can follow the steps below.I will be downloading data for the AAVE coin. This is a smaller coin, and I was not able to find a quality dataset online. I, therefore, downloaded my own dataset with the CCXT Read the full blog for free on Medium.Join thousands of data leaders on the AI newsletter. Join over 80,000 subscribers and keep up to date with the latest developments in AI. From research to projects and ideas. If you are building an AI startup, an AI-related product, or a service, we invite you to consider becoming asponsor. Published via Towards AITowards AI - Medium Share this post0 Comments ·0 Shares ·51 Views
-
Important LLMs Papers for the Week from 10/02 to 16/02towardsai.netImportant LLMs Papers for the Week from 10/02 to 16/02 0 like February 24, 2025Share this postAuthor(s): Youssef Hosni Originally published on Towards AI. Stay Updated with Recent Large Language Models ResearchThis member-only story is on us. Upgrade to access all of Medium.Large language models (LLMs) have advanced rapidly in recent years. As new generations of models are developed, researchers and engineers need to stay informed on the latest progress.This article summarizes some of the most important LLM papers published during the Second Week of February 2025. The papers cover various topics shaping the next generation of language models, from model optimization and scaling to reasoning, benchmarking, and enhancing performance.Keeping up with novel LLM research across these domains will help guide continued progress toward models that are more capable, robust, and aligned with human values.LLM ProgressLLM ReasoningLLM Preference Optimization & AlignmentLLM Scaling & OptimizationRetrieval Augmented Generation (RAG)Attention ModelsLLM Evaluation & BenchmarkingMost insights I share in Medium have previously been shared in my weekly newsletter, To Data & Beyond.If you want to be up-to-date with the frenetic world of AI while also feeling inspired to take action or, at the very least, to be well-prepared for the future ahead of us, this is for you.Subscribe below to become an AI leader among your peers and receive content not present in any other platform, including Medium:Data Science, Machine Learning, AI, and what is Read the full blog for free on Medium.Join thousands of data leaders on the AI newsletter. Join over 80,000 subscribers and keep up to date with the latest developments in AI. From research to projects and ideas. If you are building an AI startup, an AI-related product, or a service, we invite you to consider becoming asponsor. Published via Towards AITowards AI - Medium Share this post0 Comments ·0 Shares ·64 Views
-
Create Your Own AI Assistant: A Practical Guide to Multimodal, Agentic Chatbots for Everyday Usetowardsai.netLatestMachine LearningCreate Your Own AI Assistant: A Practical Guide to Multimodal, Agentic Chatbots for Everyday Use 0 like February 24, 2025Share this postLast Updated on February 24, 2025 by Editorial TeamAuthor(s): Prisca Ekhaeyemhe Originally published on Towards AI. This member-only story is on us. Upgrade to access all of Medium.Have you ever wondered if you could create your own customized chatbot? Imagine a chatbot that not only writes your Medium articles but also something more advanced, like a personalized virtual travel assistant. A chatbot that you can build from scratch and share with others. While OpenAIs chat interface allows some customization, it requires a ChatGPT Plus subscription at $20/month or a Pro plan at $200/month for unlimited access.AI Generated image by authorBut what if you could build one yourself for a fraction of the cost? Non members can read it here.In this article, Ill walk you through how I built a customized, multimodal chatbot from scratch. My chatbot doesnt just chat; it generates images, produces audio responses, and even fetches real-time data from the web. Youll learn how to create your own intelligent assistant without paying a $200 subscription fee plus, youll deepen your understanding of applied LLMs (Large Language Models). If you know basic Python, youre all set to follow along and build your own.Agentic AI refers to systems that can autonomously take actions to solve problems or accomplish multi-tasks on your behalf.In the course Read the full blog for free on Medium.Join thousands of data leaders on the AI newsletter. Join over 80,000 subscribers and keep up to date with the latest developments in AI. From research to projects and ideas. If you are building an AI startup, an AI-related product, or a service, we invite you to consider becoming asponsor. Published via Towards AITowards AI - Medium Share this post0 Comments ·0 Shares ·65 Views
-
Quantum AI Computingtowardsai.netQuantum AI Computing 0 like February 23, 2025Share this postAuthor(s): Mirko Peters Originally published on Towards AI. Explore the fascinating world of quantum computing, its groundbreaking technology, and how it stands to revolutionize our understanding of reality. Well delve into its core concepts like cubits, superposition, and entanglement, presenting easy-to-understand analogies and real-world applications.This member-only story is on us. Upgrade to access all of Medium.Imagine a world where the rules of what we know about computing, reality, and even existence are turned on their heads. Youre scrolling through this blog, but somewhere out there, another you is doing the same. Welcome to the fascinating journey into quantum computing, where possibilities are as abundant as the stars in the sky! This post delves into the heart of what quantum computing is and how devices like Googles groundbreaking quantum computer are about to make our current tech seem outdated and trivial. The Quantum Leap: What Makes It Different? Navigating the New Frontiers of Computing Understanding Superposition and Entanglement The Race for Quantum Dominance Real-World Applications: Changing Industries Hurdles on the Quantum Path Conclusion: Embracing the Quantum EraWhen you think about computers, you probably picture bits. These are the basic units of information, where each bit can be either a zero or a one. But what if I told you theres something even more powerful called qubits? So, whats the difference? Lets dive into the world of quantum computing.In classic computing, everything revolves around bits. A bit is like a light switch it can be Read the full blog for free on Medium.Join thousands of data leaders on the AI newsletter. Join over 80,000 subscribers and keep up to date with the latest developments in AI. From research to projects and ideas. If you are building an AI startup, an AI-related product, or a service, we invite you to consider becoming asponsor. Published via Towards AITowards AI - Medium Share this post0 Comments ·0 Shares ·86 Views
-
DeepSeek vs. ChatGPT A Detailed Architectural and Functional Breakdowntowardsai.netLatestMachine LearningDeepSeek vs. ChatGPT A Detailed Architectural and Functional Breakdown 0 like February 21, 2025Share this postAuthor(s): Veritas AI Originally published on Towards AI. This member-only story is on us. Upgrade to access all of Medium.The Large Language Models have changed the face of Natural Language Processing by enabling machines to generate human-like copies of text, translate languages, summarize texts, and perform a multitude of other tasks. Fast advances in large-language-modeling have resulted in the production of surface differences across AI systems in terms of architectures, training methodology, and overall performance.This article elaborates on two of the leading models, ChatGPT and DeepSeek, in its comparison, focusing on the architectural design, training methodologies, performance, and limitations.ChatGPT uses a transformer architecture. It is modeled on the GPT series. Currently, the most reconfigurable model is GPT-4-a decoder-only Transformer model that can have billions of parameters all tuned for it.Transformer Architecture From Attention is all you need paper by Vaswani, et al., 2017 [1]The major architectural features include::Multi-head self-attention mechanisms: This provides attention to segments of the input sentence all at once, capturing long-range dependencies and contextual relationships.Layer normalization and residual connections: These stabilize the training process and improve gradient flow, allowing the model to scale on deep architectures.Layer normalization and residual connections: These techniques stabilize the training process and improve gradient flow, enabling the model to scale Read the full blog for free on Medium.Join thousands of data leaders on the AI newsletter. Join over 80,000 subscribers and keep up to date with the latest developments in AI. From research to projects and ideas. If you are building an AI startup, an AI-related product, or a service, we invite you to consider becoming asponsor. Published via Towards AITowards AI - Medium Share this post0 Comments ·0 Shares ·50 Views
-
Arbitration for AI: A New Frontier in Governing Uncensored Modelstowardsai.netArbitration for AI: A New Frontier in Governing Uncensored Models 0 like February 21, 2025Share this postAuthor(s): Mohit Sewak, Ph.D. Originally published on Towards AI. AI Justice League: When models go wild, arbitration calls for backup!Hey there, future AI whisperers and digital dynamos! Dr. Sewak here, your friendly neighborhood AI researcher, and today we are diving deep into a topic thats hotter than Bengaluru traffic in summer Uncensored AI. Now, before you picture robots running wild and taking over the world like in some Hollywood blockbuster Terminator anyone? lets pump the brakes a bit. Its not quite Skynet just yet, but its definitely a space where things can get interesting.Think of AI models like kids in a candy store. Censored or aligned AI? Those are the well-behaved kids, the ones told No candy before dinner! (OpenAI, 2024a). Theyre trained to be polite, avoid saying naughty words, and generally play nice. Theyve got filters stricter than my mom checking my search history back in the day. These models are all about ethical guidelines, American values, and political correctness basically, theyre trying to be the golden children of AI (JarvisLabs, 2024).Pro Tip: Always question the source, even if its AI. Uncensored doesnt mean unbiased or factual it just means unfiltered!But then, BAM! Enter the uncensored models (Zem, 2024). These are the rebels, the rule-breakers, the AI equivalent of that cool kid in school who wore ripped jeans and listened to rock music. Theyre designed to give it to you straight, no chaser, no filters. Theyre like, You want info? Ill give you ALL the info, the good, the bad, and the downright weird! They aim to process and spit out everything theyve learned, holding nothing back. Sounds exciting, right? Like finally, AI that tells it like it is!Trivia Time: Did you know the term censorship in AI is kinda borrowed from human societies? But whos the censor for AI? Thats the million-dollar question were tackling!With great power, comes great responsibility. Uncle Ben, Spider-Man.(Yeah, even Spidey knew AI ethics before it was cool. Dr.Sewak)Now, as someone whos been in the AI trenches at places like Google, NVIDIA, Microsoft R&D, IBM Labs, and even wrestled with AI in the banking world at Bank of New York Mellon, I can tell you firsthand this uncensored thing? Its a double-edged sword. Its got the potential to unlock crazy innovation think research breakthroughs, wild creativity, and access to info like never before. But, and this is a BIG but, it also opens up a Pandoras Box of risks (Bommasani et al., 2021; Weidinger et al., 2021). Were talking misinformation on steroids, content so unethical itd make a politician blush, and outputs that could be weaponized faster than you can say deepfake (Metz, 2023; Solaiman et al., 2023).Uncensored AI: Pandoras Box or Treasure Chest? Handle with extreme care!So, what do we do? Do we slap a giant CENSORED sticker on all AI and call it a day? Nah, thats like putting toothpaste back in the tube messy and impossible. Plus, as someone whos authored books like Deep Reinforcement Learning (Springer Nature) and Convolutional Neural Networks, (Packt), I believe in pushing boundaries, not building walls. Censorship can be a slippery slope, leading to over-alignment, where AI becomes so vanilla, its about as useful as a chocolate teapot (Digit, 2025). And trust me, in the AI world, being useless is the ultimate insult.Thats where arbitration comes in, my friends. Think of it as the AI referee, the wise old judge, the okay, you get it. Its a way to manage the chaos, to set some ground rules for these uncensored models without killing their innovative spirit. Its about finding that sweet spot, that balance between wild west freedom and responsible AI citizenship. Its a New Frontier, baby! And were gonna explore it together. Ready to ride? Lets dive into the nitty-gritty of this arbitration thing and see if we can tame this uncensored AI beast!The Uncensored AI Beast: Taming the Wild West with ArbitrationCan we harness the power of unrestricted AI without unleashing chaos? A novel framework for responsible governance.Alright, so weve established that uncensored AI is like that super-talented but slightly reckless friend we all have. They can do amazing things, but you also kinda hold your breath when theyre in charge of the party playlist. The core issue boils down to this censorship thing or rather, the lack of it (Ovadia, 2023).Pro Tip: Think of censorship in AI not as bad, but as alignment. Its about aligning AI with our values, not just silencing it.The only way to do great work is to love what you do. Steve Jobs.(And in AI, great work means responsible work, even with uncensored models. Dr.Sewak)Now, censored AI or as the cool kids call it, aligned AI is all about filters, baby (OpenAI, 2024b). Think of Instagram filters, but instead of making your selfies look better, these filters are supposed to make AI behave better. These filters are built on a cocktail of things we humans call ethical standards:Societal Norms: Basically, whats considered normal and acceptable in society. Think holding doors open for people, saying please and thank you, and not, you know, generating hate speech (JarvisLabs, 2024). These norms, however, are often skewed towards where the AI is developed kinda like how American TV shows dominate global streaming, right?Legal Standards: Laws are like the ultimate do not cross lines. AI models are supposed to steer clear of illegal stuff like defamation, inciting violence, or anything that lands you in actual jail (Solan, 2002). No AI jail just yet, thankfully.Ethical Guidelines: These are the shoulds and oughts of AI. Think fairness, transparency, accountability the kind of stuff Ive been researching and writing about in my Medium blogs. Its about making AI thats not just smart, but also well, decent (Floridi, 2023).Company Values: Big companies like Google AI and others have their own ethical principles. Its like their AIs personality they want their models to reflect their brand, their image, their vibe (Google AI, 2024).Trivia Time: The debate around AI censorship is older than you think! Sci-fi has been wrestling with this for decades, from 2001: A Space Odysseys HAL 9000 to Westworlds rogue robots!AI Filters: Making models behave, one filter at a time.The goal of all this alignment is simple: make AI a force for good, build trust, and avoid robot uprisings you know, the usual (Russell, 2019). Companies like OpenAI are pouring resources into this, trying to make ChatGPT and its buddies the responsible citizens of the AI world (OpenAI, 2024c).But heres the plot twist, folks. Censorship, even in AI, can backfire. Go too strict, and you get over-alignment (Carlini et al., 2020). Imagine a chatbot so worried about being offensive, it refuses to talk about anything remotely interesting or controversial. Hey AI, tell me about the French Revolution. Im sorry, but discussing revolutions might be triggering for some users. Can I interest you in cat videos instead? Frustrating, right? And kinda useless (Digit, 2025). Its like that friend whos so afraid of saying the wrong thing, they end up saying nothing at all.Plus, who decides whats ethical anyway? Ethics are like fashion trends they change, they vary across cultures, and whats cool in California might be cringe in Kolkata. Embedding specific ethics into AI can lead to bias, and suddenly, your aligned AI is just aligned to one viewpoint (Birhane et al., 2021). Not exactly the unbiased, all-knowing oracle we were promised.Pro Tip: Remember, AI is a tool. Like any tool, it can be used for good or bad. Its about responsible use, not just censorship.And heres a thought that keeps me up at night are we stifling innovation with all this censorship? Are we missing out on breakthroughs because were too scared of AI stepping out of line? Are we creating an innovation bottleneck in our rush to be responsible? (Vincent, 2023). Its like telling a rockstar to only play elevator music technically music, but kinda missing the point, right?Trivia Time: Did you know that early internet pioneers were fiercely against censorship? They envisioned the web as an uncensored space for free information flow. AI is kinda facing a similar crossroads now.The best way to predict the future is to create it. Peter Drucker.(But we gotta create it responsibly, folks! Dr.Sewak)Now, flip the coin. Uncensored AI. Sounds edgy, sounds rebellious, sounds like trouble? Maybe. But also, maybe opportunity? These models are designed to be information free-flow zones (Perez, 2024). Think of them as the internet, unfiltered, in AI form. Their core principles are:Information Freedom: Like the ACLU says, free speech is kinda a big deal. Uncensored AI leans into that, aiming for unrestricted access to info and ideas. Its like the AI version of a public library, with no librarian telling you what you cant read.Comprehensive Data Dive: These models want to use all the data theyre trained on the spicy memes, the controversial debates, the weird corners of the internet. No cherry-picking, no sanitizing. Its like saying, Give me the whole buffet, even the questionable-looking tuna casserole! (Luccioni et al., 2021).Exploration Powerhouse: Uncensored AI can dive into topics aligned models might shy away from. Think cutting-edge research, exploring taboo subjects, pushing creative boundaries. Its like letting a scientist loose in a lab with no safety goggles potentially dangerous, but maybe theyll discover something amazing (Vincent, 2023).User Control Nirvana: Imagine AI that you can actually control. Want it vanilla? Censored-lite? Full-on uncensored chaos? You get to choose! Its like having a volume knob for AI ethics crank it up or down as you please (Wolfram, 2023).AI Censorship: Dial it up, dial it down user control is the name of the game?Sounds like a utopia of information, right? Well not so fast. Uncensored AI is also a potential minefield. Remember that reckless friend? Yeah, they can also crash your car. The risks are real, and theyre kinda scary:Hate Speech Bonanza: Uncensored models can spew out racist, sexist, and hateful garbage faster than a Twitter argument. This aint just theoretical it can fuel online harassment, discrimination, and real-world harm (Gebru et al., 2018).Misinformation Mayhem: Fake news? Propaganda? Conspiracy theories? Uncensored AI is like a super-spreader event for all of the above. It can erode trust faster than a politicians promise and mess with everything from elections to public health (Pennycook & Rand, 2019).Weaponized AI: Cybercrime, deepfakes, sophisticated scams uncensored AI can be weaponized by bad actors faster than you can say cybersecurity breach (Brundage et al., 2018). Remember that CNN article I read? ChatGPT Is a Cyber Weapon Waiting to Be Weaponized (Metz, 2023). Chilling, right?Trust Gone with the Wind: If uncensored AI runs wild, spewing garbage and causing chaos, public trust in all AI even the good stuff will vanish faster than free pizza at a tech conference (Cave & Dihal, 2023). And thats bad news for everyone in the AI game.Open Source = Open Season? Many uncensored models are open source, which is awesome for access and innovation, but also kinda risky? Malicious folks can tinker with them, exploit vulnerabilities, and unleash AI mayhem without anyone really knowing who to blame (Dark Reading, 2025; Orca Security, 2024). And open-source licenses? Dont even get me started on the legal headaches (Contreras, 2015).Pro Tip: Open source AI is like open source software powerful and democratizing, but needs community responsibility and oversight.Trivia Time: The term open source comes from software development, but its now transforming fields from biology to AI!With freedom comes responsibility. Eleanor Roosevelt.(Uncensored AI needs responsibility baked in, not bolted on. Dr.Sewak)So, were stuck between a rock and a hard place, right? Censorship too much, innovation dies. Censorship too little, chaos reigns. Is there a middle path? Is there a way to get the best of both worlds the power of uncensored AI, minus the apocalypse? I think there is. And its called arbitration.Stay tuned, folks, because things are about to get arbitrated!Arbitration: A New Frontier in Governing Uncensored ModelsDelving into a structured framework to address the unique challenges of unfiltered artificial intelligence.Okay, so weve painted a picture of uncensored AI as this wild, untamed beast powerful, yes, but also potentially bitey. Weve seen that censorship alone isnt the answer. So, what is the answer? Drumroll please Arbitration! Yeah, I know, sounds kinda legal-y and boring. But trust me, in the AI world, arbitration is about to become the new black.Pro Tip: Arbitration isnt just for legal eagles. Its a problem-solving tool, a way to navigate complex issues fairly and effectively.Trivia Time: Arbitration is ancient! It dates back to ancient Greece and Rome, way before AI or even the internet was a twinkle in anyones eye. Humans have been squabbling and needing mediators for millennia!The arc of the moral universe is long, but it bends towards justice. Martin Luther King Jr.(Arbitration is about bending the AI universe towards justice and responsibility. Dr.Sewak)Now, forget those images of stuffy courtrooms and endless paperwork. In the context of uncensored AI, arbitration is something different. Its a structured, independent process for sorting out the messes that uncensored AI might create. Think of it as AIs ethical debugger? Yeah, lets go with that. Heres the breakdown of what AI arbitration, in my Dr. Sewak-approved version, actually means:Neutral Third Party to the Rescue: Imagine two AI models having a digital shouting match (it happens, trust me). Arbitration brings in a neutral referee someone whos not on either side, someone impartial to make a fair call. This is crucial because self-regulation in the AI wild west? Yeah, good luck with that. We need someone objective (IndiaAI, 2024; JDSupra, 2024).Expert Brainpower Unleashed: These arent just any referees. Were talking AI ethics gurus, tech law ninjas, cybersecurity commandos, and domain experts from every field you can imagine (Norton Rose Fulbright, 2024). Think of it as the Avengers of AI ethics, assembled to tackle the toughest AI dilemmas. Expertise is key because AI problems are well, complex.Flexibility is the Name of the Game: Arbitration isnt one-size-fits-all. Its like a Swiss Army knife of dispute resolution. Mediation for the chill disputes, expedited processes for the urgent ones, and full-blown hearings for the big kahunas. AI problems are diverse, so the solutions need to be too (PON, 2025; Norton Rose Fulbright, 2024). AI can even help manage these cases AI-powered case management? Mind blown! (IndiaAI, 2024; JDSupra, 2024).Fix the Problem, Prevent the Future: Arbitration isnt just about slapping wrists. Its about fixing the harm now and stopping it from happening again. Its about recommending fixes, setting guidelines, and nudging the whole AI ecosystem towards responsible behavior. Its like AI therapy, but for the whole industry.Transparency Sort Of: Okay, full transparency might be tricky (trade secrets, privacy, etc.). But the process should be transparent, and the outcomes as transparent as possible. Accountability is key, even for AI. We need to build trust, not just black boxes.The AI Arbitration Dream Team: Expertise, impartiality, and a whole lot of brainpower.So, how does this AI arbitration magic actually work? Lets break down the key ingredients of this framework, Dr. Sewak style:3.2.1. The Avengers Assemble: Multi-Stakeholder Arbitration PanelThink of this panel as the Justice League of AI ethics. We need a diverse crew, folks, not just tech bros in hoodies. This panel needs:AI Ethics Gurus: These are the folks who actually think about AI ethics all day. They know their Kant from their Keras, their utilitarianism from their well, you get the idea. Theyre the moral compass of this whole operation.Legal Eagles: Lawyers, legal scholars the folks who speak legalese fluently. Theyll navigate the legal minefields, figure out liability, and make sure our arbitration process is, you know, legal.Tech Wizards: We need the techies, the coders, the model whisperers. They understand the nuts and bolts of AI, the black boxes, the algorithms gone wild. Theyll be the translators between the ethical and legal folks and the actual AI tech.Domain Deep Divers: AI is everywhere, from healthcare to finance to cat video recommendations. We need experts from different fields to understand the context of AI harms. A healthcare AI gone wrong? Needs healthcare experts. A finance AI causing market mayhem? Bring in the finance gurus.Public Voice Amplifiers: Lets not forget the people! We need reps from civil society, consumer groups, the folks who actually use AI and get affected by it. Their voice matters, big time.Pro Tip: Diversity isnt just a buzzword. Its essential for fair and effective AI governance. Different perspectives, different expertise thats where the magic happens.Trivia Time: The concept of multi-stakeholder governance is hot in tech right now. Its about bringing everyone to the table industry, government, civil society, academia to solve complex problems together.None of us is as smart as all of us. Ken Blanchard.(Teamwork makes the AI dream work responsibly. Dr.Sewak)This panel needs to be squeaky clean independent, impartial, no conflicts of interest. Think of it as the AI version of Switzerland neutral and trustworthy. And term limits? Yes, please! Fresh blood, fresh perspectives, keep it dynamic.3.2.2. Complaint Central: Dispute Intake and AssessmentOkay, panels assembled. Now, how do people actually file a complaint when uncensored AI goes off the rails? We need a system, folks, not just frantic emails and angry tweets. Think Complaint Central, AI edition:Multiple Entry Points: Online forms, email, maybe even a Bat-Signal for really urgent cases. Make it easy for everyone to report issues users, developers, random folks on the internet who spot something fishy.Standardized Forms No Mystery Novels: Complaint forms should be clear, simple, and capture the key info what happened, which AI model, who got hurt, evidence, the whole shebang. No cryptic riddles, please.Triage Time Sorting the Signal from the Noise: Not every complaint will be legit. We need a quick filter to weed out the my AI insulted my cat complaints from the this AI is spreading election misinformation emergencies. AI can even help here AI triage? Meta-AI! But human eyes on the prize, always.Investigation Mode Sherlock Holmes vs. AI: Serious complaints? Time for a deep dive. Evidence gathering, interviews, tech forensics the full detective toolkit. The arbitration panel oversees this, making sure its fair, thorough, and not a witch hunt.Pro Tip: Make reporting easy and accessible. The more eyes and ears on the ground, the better we can catch AI issues early.Trivia Time: Triage comes from battlefield medicine! Its about prioritizing cases based on urgency. Makes sense for AI harms too, right?Justice delayed is justice denied. William E. Gladstone.(We need a system thats not just fair, but also efficient. Dr.Sewak)3.2.3. Flexibility is the Superpower: Arbitration ProceduresAI problems are like snowflakes no two are exactly alike. So, arbitration needs to be flexible. Think choose your own adventure dispute resolution:Mediation Magic Lets Talk it Out: Sometimes, all it takes is a neutral mediator to get people talking, to find common ground. Mediation is like AI couples therapy helping parties understand each other, find solutions, and maybe even hug it out at the end (PON, 2025). AI can even assist in mediation analyzing communication, suggesting compromises (IndiaAI, 2024)! Mind. Blown. Again.Expedited Express Lane Speedy Justice: Small disputes, urgent issues? Expedited arbitration is your friend. Fast track procedures, streamlined evidence, quicker decisions. Think AI dispute resolution on fast-forward.Full-Blown Arbitration Arena When Things Get Serious: Big, complex, contentious cases? Bring out the full arbitration machinery. Formal hearings, legal arguments, expert testimony, the whole nine yards. Think AI court, but hopefully less dramatic than TV court.Advisory Wisdom Guidance, Not Orders: Sometimes, we just need advice. For novel ethical dilemmas, for cutting-edge tech issues, the panel can issue non-binding advisory opinions. Think AI Yoda, dispensing wisdom to guide the AI galaxy.Pro Tip: Flexibility is key. Match the dispute resolution process to the type of dispute. Not every AI spat needs a full-blown legal battle.Trivia Time: Mediation and arbitration are often used interchangeably, but theyre different! Mediation is about facilitation, arbitration is about adjudication. Now you know!The greatest remedy for anger is delay. Seneca.(But in the AI world, sometimes speed is also of the essence. Balance, people, balance! Dr.Sewak)The panel gets to decide which procedure fits best for each case. Theyre the AI dispute resolution chefs, choosing the right recipe for each problem.3.2.4. Fix it and Forget it? Nope! Remediation and GovernanceArbitration isnt just about slapping wrists and saying dont do it again. Its about fixing the problem and preventing future screw-ups. Think remediation and AI governance recommendations! Catchy, right? Heres what it means:Remediation Rumble Making Things Right (Now): Harm done? Arbitration panel to the rescue! They can recommend (or even mandate!) fixes: Model Makeovers: AI model, youre grounded! Go back to training and fix your biases! Panel can order developers to tweak the model, refine the data, add safety features without going full censorship. Usage Limits Time Out for AI: AI model, youre banned from Twitter for a week! Usage restrictions, especially in high-risk areas. Think AI probation. Show Me the Money Compensation for Harm: Real harm, real consequences. Panel can recommend compensation for victims. AI accountability with teeth. Public Apology Tour Say Youre Sorry, AI: AI model, issue a public apology for spreading misinformation! Public apologies, corrections transparency and making amends.Pro Tip: Remediation is about fixing the immediate problem. Governance recommendations are about preventing future problems. Both are crucial for responsible AI.Governance Guidance Setting the Rules of the AI Road (for the Future): Arbitration isnt just reactive. Its proactive too! The panel becomes the AI governance guru, dishing out wisdom to: Developers: Hey AI devs, heres a playbook for responsible uncensored AI development! Data curation tips, safety testing checklists, transparency guidelines the whole shebang! Deployers: Companies using uncensored AI, listen up! Risk assessment frameworks, user agreements that actually make sense, content moderation strategies that dont kill innovation get on board! Policymakers: Governments, regulators, are you listening? Heres what weve learned, heres what works, heres how to regulate AI without stifling progress! Policy recommendations, AI style! Standard Setters: Industry standards, anyone? Lets create some benchmarks for responsible uncensored AI! Voluntary standards, industry-wide guidelines lets raise the bar!Trivia Time: Governance sounds boring, but its actually about shaping the future! Think of it as designing the rules of the AI game, making sure its a game we all want to play.An ounce of prevention is worth a pound of cure. Benjamin Franklin.(Governance recommendations are the ounce of prevention for uncensored AI. Dr.Sewak)Arbitration isnt just about solving todays AI headaches. Its about building a better AI future, one arbitration case at a time.3.2.5. Shine a Light: Transparency and AccountabilitySunlight is the best disinfectant, right? Transparency and accountability are the secret sauce of effective arbitration. We need to build trust, and trust comes from well, trust and transparency (and maybe a little bit of magic).Process Transparency Open the Black Box (a Little): Arbitration procedures, rules, panel member selection all public knowledge. No secret AI cabals here. Fairness needs to be seen to be believed.Outcome Transparency Share the Wisdom (Carefully): Case summaries, anonymized decisions, key findings lets share the lessons learned. But confidentiality matters too. Trade secrets, privacy gotta balance openness with discretion.Accountability Anchors Making it Stick: Arbitration decisions are great if they actually mean something. We need ways to ensure compliance:Voluntary Buy-In Good AI Citizens: Industry norms, ethical peer pressure, reputational points lets encourage voluntary compliance. Make responsible AI the cool kid club.Contractual Clout Ink it and Link it: Arbitration clauses in user agreements, developer contracts make arbitration legally binding, at least in some contexts.Regulatory Backup Government to the Rescue? Maybe, maybe not. Regulators could recognize arbitration decisions, give them some legal teeth. Tricky balance though dont want to stifle innovation with red tape.Public Shaming (and Praising) Community Power: Public reports, monitoring, community watchdogs sunlight as disinfectant, remember? Shine a light on good AI actors and less good ones.Pro Tip: Transparency and accountability build trust. Trust is essential for the long-term success of AI.Trivia Time: Transparency is a buzzword, but its also a fundamental principle of good governance, from democracies to AI arbitration frameworks!Trust, but verify. Ronald Reagan (and a Russian proverb).(Transparency is the verify part of AI trust. Dr.Sewak)Arbitration needs to be more than just talk. It needs to be real, effective, and accountable. Transparency is how we get there.And guess what? AI can even help run arbitration! Meta-meta-AI!3.3. AI-Powered Arbitration: Robot Referees?Yeah, you heard that right. AI can actually make arbitration better? More efficient? Less human-error-prone? Maybe. Think AI as the arbitration sidekick:Case Management Bots AI Paper Pushers: Automate the boring stuff case intake, document wrangling, scheduling, reminders. Let AI handle the paperwork, humans handle the human stuff (JDSupra, 2024).Evidence Analysis AI Data Detective: Mountains of text, audio, video evidence? AI can sift through it, find patterns, summarize key points, flag inconsistencies. Think AI Sherlock Holmes, but for data (IndiaAI, 2024). NLP and machine learning to the rescue!Legal Research AI Lawyer in a Box: Arbitrators need to know the law, precedents, ethical guidelines. AI legal research tools can speed things up, find relevant cases faster than you can say amicus curiae.Mediation AI-ssistant Peace-Making Algorithms: AI can analyze communication in mediation, spot roadblocks, suggest compromises, nudge parties towards agreement (PON, 2025). Think AI relationship counselor, but for AI disputes.Bias-Busting AI Fairness Enforcer: Irony alert! AI can help detect and mitigate bias in the arbitration process itself (Norton Rose Fulbright, 2024). Bias in arbitrator selection? AI can flag it. Bias in evidence interpretation? AI can help spot it. But careful here. AI bias-detectors need to be bias-free themselves! Its bias-ception!Pro Tip: AI can augment arbitration, but it shouldnt replace humans. Human judgment, ethical reasoning, empathy still essential.Trivia Time: AI is already being used in real-world arbitration and mediation (e.g. IndiaAI ADR Mechanism, JAMS Resolution Center). The future is now, folks!Technology is best when it brings people together. Matt Mullenweg.(AI can bring people together to resolve AI disputes! Dr.Sewak)Irony, again!AI in arbitration its not about robot judges taking over. Its about humans and AI working together to make the process fairer, faster, and more effective. Its about harnessing AI to govern AI. Full circle, baby!Harnessing Power, Mitigating Risks: Real-World Arbitration in ActionExploring the potential benefits and perils of unrestricted AI and how arbitration can bridge the gap.Theory is cool, but real-world examples? Thats where the rubber meets the road. Lets dive into some case studies, Dr. Sewak style, to see how this arbitration thing might actually work in practice. Think of these as AI dispute hypotheticals, inspired by real-world AI scenarios and the kind of stuff Ive seen brewing in the AI world.Pro Tip: Case studies arent just stories. Theyre thought experiments, ways to test ideas and anticipate future challenges.Trivia Time: Case studies are a staple in business schools and law schools. Now, theyre becoming essential for AI ethics and governance too!Tell me and I forget. Teach me and I remember. Involve me and I learn. Benjamin Franklin.(Case studies are about involving us in the learning process. Dr.Sewak)4.1. Case Study 1: Open-Source LLMs Gone Rogue Misinformation MayhemThe Setup: Imagine a top AI lab releases an open-source, uncensored Large Language Model (LLM). Think Metas Llama 2, but even less filtered. The goal? Innovation, open access, AI for the people! Sounds noble, right? Well this model becomes a misinformation factory overnight. Bad actors weaponize it to pump out hyper-realistic fake news during a crucial election. Social media explodes with AI-generated propaganda, trust in institutions crumbles, democracy well, you get the picture (Dark Reading, 2025).Misinformation Machine: When open-source AI becomes a propaganda factory.The Harm: Massive misinformation, manipulated public opinion, democratic meltdown, maybe even real-world violence. Not good.Arbitration to the Rescue? Enter the AI Arbitration Panel! Who files a complaint? Affected citizens, civil society groups, maybe even governments. The panel investigates: Did this open-source model directly cause the misinformation storm? Whose responsibility is it? The AI lab that released it? The malicious actors who weaponized it? Both?Remediation Rumble:Tech Fixes (Sort Of): Cant really censor open-source code once its out there. But maybe the panel recommends open-source safety patches, AI tools to detect AI-generated misinformation. Think open-source antibodies for the AI misinformation virus.Governance Guidance Open Source Rules of the Road: Panel issues recommendations to AI labs, open-source communities: Responsible release practices for uncensored models, folks! Risk assessments, transparency, community moderation make it happen!Public Awareness Blitz: Panel pushes for public education campaigns: Hey citizens, AI misinformation is real! Learn to spot it, think critically, be media literate!Relevant Companies/Models: Metas Llama 2, Hugging Face open-source models, the whole open-source LLM ecosystem.Dr. Sewak Says: Open source is awesome, but open responsibility needs to be part of the deal. Think open source software security its a community effort. Same for AI ethics.4.2. Case Study 2: Creative AI Copyright Clash Artistic Anarchy?The Setup: Startup X launches an uncensored AI model for creative content writing, music, visual art, the works. Its a hit! Users are creating amazing, novel stuff. But wait a minute. Some of this original AI art looks suspiciously familiar. Turns out, the AI is kinda borrowing a lot from copyrighted material. Content creators, copyright holders theyre not happy (Stability AIs Stable Diffusion is kinda in this space, just sayin).AI Artist or AI Art Thief?The Harm: Copyright violations, artists losing income, creative industries in chaos, legal battles galore. Not a pretty picture.Arbitration to the Rescue? Copyright holders, artists file complaints. Arbitration panel copyright law experts, AI techies investigates: Is this real copyright infringement? Is AI transformative use or just plain copying? Wheres the line?Remediation Rumble:Copyright Court, AI Edition: Panel assesses copyright infringement, applies fair use doctrines, figures out if AI crossed the line.Licensing Deals AI Art Royalties? Panel recommends licensing agreements between AI startup and copyright holders. AI art royalties new revenue stream for artists? Maybe.Governance Guidance Copyright Rules for AI Creators: Panel issues guidelines for AI developers: Copyright compliance checklist for creative AI! Ethical data sourcing, infringement detection mechanisms get it done! Maybe even ethical AI dataset standards (Solaiman et al., 2023).Relevant Companies/Models: Stability AIs Stable Diffusion, AI music generators, AI writing tools the whole creative AI boom.Dr. Sewak Says: AI creativity is cool, but copyright is still a thing. We need to figure out how AI and copyright can coexist peacefully. Think AI music licensing, AI art royalties new business models for a new era.4.3. Case Study 3: Uncensored AI Bias in High-Stakes Decisions Algorithmic DiscriminationThe Setup: Company Z deploys an uncensored AI model for loan applications, hiring, criminal risk assessment you know, the important stuff. Turns out, the uncensored model is biased. Surprise! It starts discriminating against certain demographic groups unfair loan denials, biased hiring, skewed risk scores. People are getting hurt, opportunities are being denied, and lawsuits are brewing (think COMPAS risk assessment tool, AI hiring platforms real-world examples of algorithmic bias).Algorithmic Justice? When uncensored AI decisions become discriminatory.The Harm: Systemic discrimination, inequality amplified, lives impacted, trust in AI in critical domains shattered.Arbitration to the Rescue? Affected individuals, civil rights groups file complaints. Arbitration panel algorithmic fairness experts, bias detection gurus, human rights lawyers investigates: Is this AI really biased? How bad is it? Whos responsible for fixing it?Remediation Rumble:Bias Busting Bootcamp for AI: AI model, report to debiasing bootcamp, stat! Panel orders model retraining with debiased data, fairness-aware algorithms (Mehrabi et al., 2021). AI bias correction, in real-time.Algorithmic Audits Check Under the AI Hood: Panel mandates ongoing algorithmic audits, bias monitoring. Company Z, show us your AI fairness report card! Regularly!Human Override Button Humans in the Loop: In high-stakes decisions, humans must have the final say! AI is a tool, not a dictator! Human oversight, human accountability.Compensation for Victims Making Amends for AI Bias: Panel recommends compensation for those harmed by AI discrimination. Putting a price on AI-driven unfairness.Governance Guidance Fairness First in AI Deployment: Panel issues guidelines for organizations deploying AI in high-stakes areas: Ethical AI frameworks are not optional! Fairness, transparency, accountability bake it in from day one! Promote ethical AI standards (Mkander & Hagras, 2022).Relevant Companies/Models: AI in finance, HR tech, criminal justice domains where bias is a critical concern.Dr. Sewak Says: Algorithmic bias is real. And uncensored AI can amplify it big time. Fairness needs to be baked into AI from the start, not bolted on as an afterthought. Human oversight is non-negotiable in high-stakes AI decisions.These case studies, while hypothetical, are rooted in real-world AI challenges. They show that uncensored AI, while powerful, needs governance. And arbitration, my friends, might just be the new frontier in making that governance a reality.Challenges and the Path Forward: Navigating the AI Governance MazeAcknowledging limitations and discussing future directions for arbitration in the age of uncensored AI.Lets be real, folks. This arbitration for uncensored AI thing? Its not a magic wand. Its not a perfect solution. Its a framework, a proposal, a work in progress. There are challenges, speed bumps, and maybe even a few AI-sized potholes on this road to responsible AI governance. Lets face them head-on, Dr. Sewak style.Pro Tip: No solution is perfect. The goal is to make things better, not perfect. Progress, not perfection.Trivia Time: Challenges are just opportunities in disguise, right? At least, thats what motivational posters tell us. In AI, challenges are definitely opportunities for innovation and problem-solving.The impediment to action advances action. What stands in the way becomes the way. Marcus Aurelius.(AI governance challenges? Lets turn them into our way forward. Dr.Sewak)5.1. Defining Unacceptable Harm: The Fuzzy LineWhat is unacceptable harm from AI, anyway? Its not like breaking a law or causing physical injury. AI harms can be fuzzy. Subjective. Context-dependent (Vallor, 2016). Whats harmful to you might be free speech to someone else. And AI operates in a million different contexts. Defining harm in AI is like nailing jelly to a wall.The Fuzzy Line: Defining unacceptable harm in the age of uncensored AI.Dr. Sewaks Mitigation Strategies:Harm-O-Meter 3000 (Multi-Dimensional Edition): We need a way to measure harm thats more nuanced than just yes/no. Think: Severity Scale: Minor annoyance to societal collapse harm isnt binary. Probability Factor: Likelihood of harm actually happening. Potential harm vs. actual harm. Vulnerability Index: Whos most likely to get hurt? Kids? Marginalized groups? Factor in vulnerability. Context Compass: Context, context, context! Same AI output, different contexts, different harm levels. Intent-O-Meter: Was harm intended? Or just AI being AI? Intent matters, sometimes.Ethical Barometer Community Standards Check: Tap into ethical guidelines, human rights frameworks, community norms. Crowdsource wisdom, dont just dictate from on high. Inclusivity is key.Case-by-Case Wisdom Human Judgment Still Matters: No algorithm can define harm perfectly. Arbitration panel needs human judgment, ethical reasoning, common sense. AI can assist, not replace, human wisdom.5.2. Enforcement Enigma The Decentralized AI Wild WestUncensored AI is often open source, decentralized, global. Enforcing arbitration decisions in this wild west? Good luck, right? Its not like we can send AI police to arrest a rogue algorithm. Traditional enforcement tools kinda useless here.Enforcement Enigma: Policing the decentralized frontier of uncensored AI.Dr. Sewaks Mitigation Strategies:Soft Power Playbook Norms, Not Laws (Initially): Focus on soft law industry best practices, ethical peer pressure, reputational incentives. Make responsible AI the norm, the cool thing to do. Arbitration framework as a norm-setter.Contractual Chains Binding Agreements (Where Possible): Incorporate arbitration clauses into user agreements, developer contracts. Make arbitration decisions contractually enforceable, at least. Legal glue for the AI wild west.Tech Transparency Tools AI Audit Trails: Tech to the rescue! AI model watermarking, provenance tracking, blockchain registries for AI. Make AI more transparent, auditable, traceable. Tech-powered accountability.Global Governance Gang International AI Cooperation: AI is global, governance needs to be too. International cooperation, harmonized frameworks, cross-border arbitration. AI governance without borders.Community Cops Open Source Responsibility: Leverage the open-source AI community itself! Community moderation, code reviews, reputational systems. Open source AI, open source responsibility. Arbitration as a community tool.5.3. Bias in Arbitration Fairness ParadoxCan arbitration itself be biased? Irony alert! Arbitrators are human, AI tools can be biased (Norton Rose Fulbright, 2024). How do we ensure fairness in the arbitration process itself? Bias-ception, again!Bias-ception: Can AI arbitration be truly unbiased? The fairness paradox.Dr. Sewaks Mitigation Strategies:Diversity Deluxe Panel Represent All Voices: Diverse arbitrators expertise, background, gender, race, geography. Diversity as a bias-buster.Transparent Arbitrator Selection Open and Honest Process: Clear criteria, conflict-of-interest checks, stakeholder input. No backroom deals, no secret AI cabals.Bias-Proof AI Tools Audit the Auditors: If AI helps arbitration, audit those AI tools for bias! Bias detection for bias detectors. Meta-bias-busting!Human in the Loop Judgment, Not Just Algorithms: Human oversight, human review, human judgment. AI assists, humans decide. Human wisdom still essential.Appeals Process Second Opinions Welcome: Appeals mechanisms, review boards. Checks and balances for arbitration itself. Accountability for the arbitrators too.5.4. Tech Tsunami Keeping Up with AI EvolutionAI is evolving faster than my caffeine addiction. Arbitration framework needs to keep pace. Outdated framework = useless framework. Adaptability is not optional, its survival.Future-Proof Arbitration: Adapting to the ever-evolving AI tsunami.Dr. Sewaks Mitigation Strategies:Regular Reboot Cycles Framework Updates, Annually (or Faster): Annual reviews, updates, revisions. Framework needs to be a living document, not a museum piece. Agile AI governance.Modular Design Plug-and-Play Governance: Modular framework architecture. Add new procedures, expertise areas, recommendations as needed. Flexibility built-in.Continuous Learning Loop AI Governance Research Lab: Research, monitor AI trends, analyze arbitration cases, learn from experience. AI governance as a continuous learning process. AI governance R&D.Pilot Programs Test in the Wild: Pilot arbitration frameworks in real-world settings. Test, refine, iterate. Real-world feedback loop.AI Research Collabs Partner with the Brains: Collaborate with AI researchers, ethicists, legal scholars. Stay ahead of the curve, tap into the best minds. AI research-governance synergy.Pro Tip: AI governance needs to be as dynamic and adaptive as AI itself. Static frameworks are doomed to fail.Trivia Time: Agile and iterative are buzzwords from software development. But theyre also key to effective AI governance in a fast-paced world.It is not the strongest of the species that survives, nor the most intelligent that survives. It is the one that is most adaptable to change. Charles Darwin.(Adaptability is survival in the AI governance jungle. Dr.Sewak)Arbitration for uncensored AI? Its not a perfect solution, but its a necessary solution. Its a work in progress, a journey, a new frontier. And like any frontier, its gonna be challenging, messy, and maybe a little bit wild west-y. But with the right framework, the right experts, and a whole lot of responsible intention, we can harness the power of uncensored AI while keeping the chaos at bay. Its a tightrope walk, folks, but its a walk worth taking. For the future of AI, for the future of well, everything. Lets arbitrate, shall we?Conclusion: Towards a Responsible Future for Uncensored AISummarizing the key takeaways and reiterating the importance of arbitration for responsible AI governance.Alright, folks, weve reached the summit of our AI arbitration mountain. Weve explored the wild landscape of uncensored AI, faced the challenges head-on, and mapped out a potential path forward arbitration. Lets zoom out for a birds-eye view, Dr. Sewak style, and recap the key takeaways.The Summit of Responsible AI: Arbitration as our path to a balanced future.Dr. Sewaks Key Takeaways The Arbitration Advantage:Balance is the Name of the Game: Uncensored AI is a double-edged sword. Arbitration offers a way to wield that sword responsibly, balancing innovation with safety, freedom with ethics. Its about finding that sweet spot, not swinging wildly in either direction.Expertise Matters, Impartiality is Essential: AI problems are complex. Arbitration brings in the Avengers of AI ethics diverse experts, neutral perspectives, informed decisions. No more AI governance by gut feeling.Flexibility Wins the Day: AI disputes are diverse. Arbitration is adaptable mediation, expedited processes, full hearings, advisory opinions. One-size-fits-none in AI governance.Fix Today, Prevent Tomorrow: Arbitration isnt just damage control. Its about remediation and governance. Fixing harms and shaping responsible AI practices for the future. Proactive, not just reactive.Transparency Builds Trust, Accountability Makes it Real: Transparency in process, accountability in outcomes. Arbitration aims for both. Trust and accountability the twin pillars of responsible AI.The Road Ahead Challenges and Opportunities:Defining Harm Still Fuzzy, Still Crucial: Defining unacceptable harm remains a challenge. Multi-dimensional assessment, community standards, human judgment the path forward.Enforcement in the Wild West Decentralization Dilemma: Enforcing arbitration in the decentralized AI landscape is tricky. Soft law, contracts, tech transparency, global cooperation, community responsibility the multi-pronged approach.Bias in Arbitration Fairness Paradox Persists: Bias can creep into arbitration itself. Diversity, transparency, AI audits, human oversight, appeals bias mitigation strategies are essential.Keeping Up with the AI Tsunami Adaptability Imperative: AI evolves fast. Arbitration frameworks must evolve faster. Regular updates, modular design, continuous learning, pilot programs, research collaborations adaptability is survival.The Dr. Sewak Vision Responsible AI Future:Arbitration for uncensored AI isnt a perfect solution, but its a vital step. Its a move towards a more responsible, more ethical, more governed AI future. Its about harnessing the immense power of AI while safeguarding human values, promoting innovation responsibly, and ensuring that AI serves humanity, not the other way around. Its a tightrope walk, yes. But its a walk we must take. For the future of AI, and for the future of us all. Lets build this new frontier together, responsibly, ethically, and with a healthy dose of arbitration, just in case things get a little uncensored. What do you say, friend? Ready to arbitrate the future?Pro Tip: The future of AI governance is not about finding a perfect solution, but about building resilient, adaptable, and ethically informed frameworks.Trivia Time: The frontier metaphor is apt for AI. Like the Wild West, AI is a new territory, full of opportunity and risk. We need to build our AI towns responsibly, with rules, sheriffs (arbitrators?), and a sense of community.The best is yet to come. Frank Sinatra.(Lets make sure the best AI future is also a responsible AI future. Arbitration can help us get there. Dr.Sewak)And thats a wrap, folks! Dr. Sewak, signing off. Go forth and arbitrate responsibly! And maybe, just maybe, we can tame this uncensored AI beast and build a future where AI is both powerful and good. Until next time, keep those algorithms ethical, and those punchlines punchy!7. References7.1. AI Ethics and GovernanceFloridi, L. (2023). The ethics of artificial intelligence. Oxford University Press.Russell, S. J. (2019). Human compatible: Artificial intelligence and the problem of control. Penguin.Pfeiffer, J., Gutschow, J., Haas, C., Mslein, F., Maspfuhl, O., Borgers, F., & Alpsancar, S. (2023). Algorithmic fairness in AI: an interdisciplinary view. Business & Information Systems Engineering, 65(2), 209222.Google AI. (2024). AI at Google: Our Principles.OpenAI. (2024a). Our approach to AI safety.OpenAI. (2024b). Safety and Policy.7.2. Uncensored AI Models and RisksJarvisLabs. (2024, December 3). Uncensored LLM Models: A Complete Guide to Unfiltered AI Language Models.Zem, O. (2024, February 29). Uncensored Models in AI. Medium.Bommasani, R., Hudson, D. A., Adeli, E., Altman, R., Arora, S., von Arx, S., & Liang, P. (2021). On the opportunities and risks of foundation models. arXiv preprint arXiv:2108.07258.Weidinger, L., Mellor, J., Rauh, M., Griffin, C., Uesato, J., Huang, P. S., & Gabriel, I. (2021). Ethical and social risks of harm from language models. arXiv preprint arXiv:2112.04359.Lyngass, S. (2023, March 16). ChatGPT is the new crypto: Meta warns hackers are exploiting interest in the AI chatbot. CNN Business.Disclaimers and DisclosuresThis article combines the theoretical insights of leading researchers with practical examples, and offers my opinionated exploration of AIs ethical dilemmas, and may not represent the views or claims of my present or past organizations and their products or my other associations.Use of AI Assistance: In the preparation for this article, AI assistance has been used for generating/ refining the images, and for styling/ linguistic enhancements of parts of content.License: This work is licensed under a CC BY-NC-ND 4.0 license.Attribution Example: This content is based on [Title of Article/ Blog/ Post] by Dr. Mohit Sewak, [Link to Article/ Blog/ Post], licensed under CC BY-NC-ND 4.0.Follow me on: | Medium | LinkedIn | SubStack | X | YouTube |Join thousands of data leaders on the AI newsletter. Join over 80,000 subscribers and keep up to date with the latest developments in AI. From research to projects and ideas. If you are building an AI startup, an AI-related product, or a service, we invite you to consider becoming asponsor. Published via Towards AITowards AI - Medium Share this post0 Comments ·0 Shares ·66 Views
-
The Future of AI: One Giant or Many Titans?towardsai.netLatestMachine LearningThe Future of AI: One Giant or Many Titans? 0 like February 21, 2025Share this postAuthor(s): Ilyas Iyoob, PhD Originally published on Towards AI. This member-only story is on us. Upgrade to access all of Medium.Right now, different large language models (LLMs) shine in different areas one excels at coding, another at generating images, another at writing. But give it time As the field matures, these differences will blur. Eventually, LLMs will become just another model, much like search engines today. But what does that mean? Will we see one dominant player, much like Google in search? Or will AI remain a competitive field with specialized champions excelling in different areas?Lets break it down using three perspectives: the advantage of being first, the dynamics of competition, and the long-term outlook for AI dominance.A recent study by the National Bureau of Economic Research tracked 2,000 peoples search habits over two weeks. The key finding? The main reason people stuck with Google wasnt quality, nor accuracyit was just the inconvenience of switching.This highlights a fundamental truth: in tech, being first to market isnt just an advantageits a moat. OpenAI, much like Google in Search, benefits from:Brand Recognition Its the default AI assistant for millions.Infrastructure Dominance The ecosystem (APIs, integrations, business adoption) is already in place.Massive Training Data A head start that compounds Read the full blog for free on Medium.Join thousands of data leaders on the AI newsletter. Join over 80,000 subscribers and keep up to date with the latest developments in AI. From research to projects and ideas. If you are building an AI startup, an AI-related product, or a service, we invite you to consider becoming asponsor. Published via Towards AITowards AI - Medium Share this post0 Comments ·0 Shares ·72 Views
-
Building a Self-Learning AI Marketing Agent with LangMem + LangGraph (Part 2)towardsai.netAuthor(s): Reelfy Originally published on Towards AI. Building a Self-Learning AI Marketing Agent with LangMem + LangGraph (Part 2)Ensuring Consistency in AI-Generated Marketing CampaignsAI Agent Bot taking memory pills. As imagined by Dall-E 3The Challenge: Creating a Consistent Brand Narrative with AIIn Part 1, we introduced the concept of AI-powered Social Media Agents capable of analyzing brand presence, generating video content, and automating posts.But what happens when AI starts creating content at scale? Without a structured memory system, AI-generated marketing materials can lose coherence, creating disjointed messaging that fails to build a recognizable brand identity over time.Imagine a brand posting one AI-generated video about sustainable fashion, followed by another completely unrelated clip encouraging clients to cut costs no matter what confusing audiences and weakening brand trust.This is where LangMem comes into play. By integrating long-term memory into our Social Media Agents, we can ensure that AI doesnt just create isolated posts, but remembers the brands story, audience preferences, and marketing themes resulting in a cohesive and evolving social media strategy.Why LangMem? The Secret Sauce for AI-Driven Marketing Consistency The LangMem SDK was just released on February 18, 2025, and here at Reelfy, were already experimenting with it to push the boundaries of AI-driven content marketing.LangMem provides an advanced memory system that allows AI agents to retain and refine knowledge over time. It goes beyond short-term context windows by enabling agents to learn from past interactions, update brand guidelines dynamically, and improve storytelling consistency.Which Type of Memory is Best for Social Media Marketing?LangMem offers three key types of memory each serving different functions in AI-driven workflows. To maintain brand consistency in AI-generated marketing videos, we focus on Semantic Memory and Procedural Memory:Building a Social Media Agent with LangMem + LangGraphLangMem alone isnt enough we need a structured way for our AI agent to access and update its memory. Thats where LangGraph comes in. By integrating LangMems memory tools into LangGraphs agent framework, we create a self-learning marketing assistant that can analyze, generate, and refine content in real time.In Part 1, we used SmolAgents to orchestrate our AI Social Media Agent. This time, were using LangGraph instead. Why? LangGraph natively integrates with LangMem, making it easier to demonstrate the benefits of using a built-in memory approach.Step 1: Initializing AIs Memory A Built-in RAG for Marketing KnowledgeBefore our AI can generate strategic marketing content, it needs a place to store and retrieve knowledge over time. In this setup, LangMem serves as a built-in RAG.Lets initialize our long-term memory store, which will hold both Semantic Memory (facts & knowledge) and Procedural Memory (marketing workflows).from langgraph.store.memory import InMemoryStore# Initialize memory storage using LangGraphstore = InMemoryStore(index={"dims": 1536, "embed": "openai:text-embedding-3-small"}) Why? AI needs a persistent knowledge base to avoid generating disconnected, inconsistent content.Step 2: Storing Key Marketing Knowledge with Semantic MemoryLets define a structure for storing key marketing knowledge and create memory management tools for later use. We will first:1 Storing Key Marketing Knowledge in Semantic MemoryInstead of hardcoding knowledge into prompts, we store marketing facts in LangMems long-term memory, ensuring that AI can retrieve and apply them dynamically.from langmem import create_memory_manager, create_manage_memory_tool, create_search_memory_toolfrom pydantic import BaseModel# Define structure for marketing knowledgeclass MarketingKnowledge(BaseModel): topic: str key_insights: str# Create memory manager to store factsmemory_manager = create_memory_manager( "anthropic:claude-3-5-sonnet-latest", schemas=[MarketingKnowledge], instructions="Store key marketing facts and retrieve them for future campaign strategies.", store=store,)# Store industry knowledgemarketing_facts = [ {"topic": "Startup Founders' Challenges", "key_insights": "Founders struggle with hiring, funding, and scaling."}, {"topic": "Engaging Video Content", "key_insights": "Short-form educational videos drive 3x more engagement than static posts."}, {"topic": "Brand Differentiation", "key_insights": "Successful startups use storytelling to highlight unique brand values."}]# Store facts in AI's memoryfor fact in marketing_facts: memory_manager.invoke({"messages": [{"role": "user", "content": f"Save this fact: {fact}"}]})# Create tools for AI to interact with memorymanage_memory_tool = create_manage_memory_tool(namespace=("memories",))search_memory_tool = create_search_memory_tool(namespace=("memories",)) Why? AI now has access to real-world knowledge that it can apply when generating marketing campaigns.2 Updating Semantic Memory as New Insights EmergeMarketing knowledge is not static trends change, audience behaviors shift, and new insights emerge.Lets say we discover a new engagement trend (e.g., LinkedIn carousels are outperforming videos). We need to update AIs memory accordingly.Semantic Memory visual representation. Drawn in https://excalidraw.com/# Update AI's marketing knowledge with new industry insightsnew_insight = { "topic": "Engaging Video Content", "key_insights": "LinkedIn carousels now outperform short videos for B2B engagement."}# Store the updated fact in memorymemory_manager.invoke({"messages": [{"role": "user", "content": f"Update this fact: {new_insight}"}]}) Why? AI-generated content will now reflect the latest trends, ensuring it stays competitive and relevant.Step 3: Creating and Refining Procedural Memory for AI Marketing AgentsWith LangMem, we can teach our Social Media Agent how to structure marketing campaigns through procedural memory. To avoid inconsistent storytelling formats, our AI agent must learn the structure of effective marketing videos such as hooks, storytelling arcs, and call-to-action placement.But marketing is not static brands evolve, strategies shift, and audience engagement fluctuates.Thats why we dont just define instructions once we store them in memory and refine them over time based on market trends and performance insights. Well first:1 Storing Initial Marketing Guidelines in Procedural MemoryLets start by defining how our AI should structure marketing campaigns. We store these rules in LangMems long-term memory, ensuring the agent follows a predictable format.# Store initial campaign structure in procedural memorystore.put(("instructions",), key="marketing_campaigns", value={ "prompt": """You are a Social Media Agent. Every marketing campaign must follow this structure: 1. Hook: Start with an engaging question, bold statement, or surprising stat. 2. Educational Insight: Teach startup founders something valuable about the topic. 3. Call to Action: Encourage audience interaction with a thought-provoking question or challenge. Maintain a playful yet professional tone. Focus on creating **educational content** for startup founders."""}) Why? By saving these campaign guidelines in memory, our AI will remember and follow this structure across multiple campaigns ensuring consistency.2 Refining the Campaign Structure Based on Market InsightsAs marketing strategies evolve, we may need to update our AIs procedural memory for example, if we realize that audience engagement increases when we add a success story to the campaign format.Procedural Memory visual representation. Drawn in https://excalidraw.com/from langmem import create_prompt_optimizer# Initialize prompt optimizer to refine procedural memoryoptimizer = create_prompt_optimizer("anthropic:claude-3-5-sonnet-latest")# Retrieve current campaign structurecurrent_prompt = store.get(("instructions",), key="marketing_campaigns").value["prompt"]# Market feedback suggests success stories increase engagementfeedback = {"request": "Include a success story in every campaign to increase engagement."}# AI refines campaign structure based on feedbackupdated_prompt = optimizer.invoke({"prompt": current_prompt, "trajectories": [(current_prompt, feedback)]})# Store the updated procedural memorystore.put(("instructions",), key="marketing_campaigns", value={"prompt": updated_prompt}) Why? AI-generated campaigns keep improving, ensuring higher engagement.Step 4: Generating AI-Driven Marketing Campaigns That Learn and AdaptNow that our AI has both knowledge (Semantic Memory) and structured processes (Procedural Memory), lets generate campaigns that evolve over time.1 Creating the First Marketing Campaignfrom langgraph.prebuilt import create_react_agentfrom langgraph.config import get_store# Define prompt function to fetch instructions from memorydef prompt(state): item = store.get(("instructions",), key="marketing_campaigns") instructions = item.value["prompt"] sys_prompt = {"role": "system", "content": f"## Campaign Instructions\n\n{instructions}"} return [sys_prompt] + state["messages"]# Create AI Marketing Agentagent = create_react_agent( "anthropic:claude-3-5-sonnet-latest", prompt=prompt, tools=[manage_memory_tool, search_memory_tool], store=store,)# Generate the first campaignfirst_campaign = agent.invoke({ "messages": [{"role": "user", "content": "Create a campaign about Hiring and Team Building for startup founders."}]})print(first_campaign) # AI follows stored campaign structure2 Generating a Second Campaign That Expands on the First OneSince AI remembers past campaigns, we dont need to retrieve them manually AI automatically keeps context.# Generate a follow-up campaign while maintaining continuitysecond_campaign = agent.invoke({ "messages": [{"role": "user", "content": "Create a follow-up campaign on Product Development that builds on the Hiring and Team Building insights."}]})print(second_campaign) # AI expands on previous campaign, ensuring continuity Why? Instead of creating random standalone posts, AI develops a strategic campaign narrative.Why LangMem is a Game-Changer for AI Marketing AgentsWithout memory, AI agents generate one-off posts without continuity. With LangMem, we unlock: Brand Consistency AI remembers brand identity, voice, and messaging across all content. Content Quality Control AI follows structured campaign workflows, ensuring content remains polished and engaging. Marketing Intelligence AI remembers past campaigns, industry insights, and audience preferences, enabling smarter content decisions.Where Do We Go Next?Weve established the foundation for memory-driven marketing AI, but this is just the beginning. Here a few topics that we can explore to push the boundries further: Advanced Personalization AI dynamically tailors content for different audience segments, ensuring higher engagement. Cross-Platform Content Adaptation AI automatically repurposes content across multiple social media platforms. Real-Time Performance Optimization AI analyzes audience reactions and refines marketing strategies continuously.Stay tuned as we continue redefining AI-driven social media marketing! Written by Garry NewballReferences[1] LangChain Conceptual Guide. Retrieved From: https://python.langchain.com/docs/concepts/[2] LangMem Documentation. Retrieved From: https://langchain-ai.github.io/langmem/[3] LangGraph Documentation. Retrieved From: https://langchain-ai.github.io/langgraph/[4] LangMem SDK for agent long-term memory. Retrieved From: https://blog.langchain.dev/langmem-sdk-launch/[5] LangMem Code Examples. Retrieved From: https://github.com/langchain-ai/langmem/tree/main/examples/intro_videosJoin thousands of data leaders on the AI newsletter. Join over 80,000 subscribers and keep up to date with the latest developments in AI. From research to projects and ideas. If you are building an AI startup, an AI-related product, or a service, we invite you to consider becoming asponsor. Published via Towards AI0 Comments ·0 Shares ·70 Views
-
#63: Full of Frameworks: APDTFlow, NSGM, MLFlow, and more!towardsai.net#63: Full of Frameworks: APDTFlow, NSGM, MLFlow, and more! 0 like February 20, 2025Share this postAuthor(s): Towards AI Editorial Team Originally published on Towards AI. Good morning, AI enthusiasts! This week, we are introducing new frameworks through hands-on guides such as APDTFlow (addresses challenges with time series forecasting), NSGM (addresses variable selection and time-series network modeling), and MLFlow (streamlines ML workflows by tracking experiments, managing models, and more). You will also find useful tools from the community, collaboration opportunities for diverse skill sets, and, in my industry-special Whats AI section, I will dive into the most sought-after role: LLM developers. Enjoy the read!Whats AI WeeklyThe demand for LLM Developers is growing rapidly, and because this field is so new, there are very few experts out there. But who exactly is an LLM developer, and how are they different from software developers and ML engineers? This week in Whats AI, I dive into what this specialized role looks like, how to develop the skills for it, and what the future of work will look like. Well, briefly, software developers focus on building traditional applications using explicit code. Machine learning engineers specialize in training models from scratch and deploying them at scale. LLM Developers, however, operate in a middle ground. They customize existing foundation models, use prompt engineering to guide outputs, and build pipelines that integrate techniques like RAG, fine-tuning, and agent-based systems. Read the complete article here or watch the video on YouTube! Louis-Franois Bouchard, Towards AI Co-founder & Head of CommunityLearn AI Together Community section!Featured Community post from the DiscordCmenser has built Text Loom, a fun workspace for creating networks that manage queries and build on them, all from your terminal. It provides a powerful environment for building and manipulating node-based workflows through a text interface. Check it out on GitHub and support a fellow community member. If you have any questions or feedback, share it in the thread!AI poll of the week!With Nvidias recent drop and political tensions, do you think the company will continue to thrive with its GPUs and offers? Lets discuss this in the Discord thread!Collaboration OpportunitiesThe Learn AI Together Discord community is flooding with collaboration opportunities. If you are excited to dive into applied AI, want a study partner, or even want to find a partner for your passion project, join the collaboration channel! Keep an eye on this section, too we share cool opportunities every week!1. Cyberbubblegum_77929 is working on multiple advanced projects, such as implementing something similar to tinyGPT, building an AI research assistant, developing face detection software, and creating a clone of Google Translate. If these ideas sound exciting, join them and improve your project portfolio. Contact them in the thread for more information!2. Laufeyson5190 is learning ML basics and is inviting other beginners to create a study group. If you are starting out and prefer learning in a group, reach out in the thread!3. Rushi8208 is building a team for an AI-based project. If you are skilled in Python or computer vision, diffusion models, or GANS, you might be a great fit. Connect in the thread to learn more!Meme of the week!Meme shared by ghost_in_the_machineTAI Curated sectionArticle of the weekExplaining Transformers as Simple as Possible through a Small Language Model By Alex PunnenThis article simplifies the complex Transformer architecture, focusing on its foundational concepts like vector transformations, self-attention, and multi-head attention. It begins by explaining vector transformations, a core idea in neural networks, and contrasts traditional methods like SVMs with learned feature mappings in Transformers. It then dives into self-attention, highlighting its role in creating contextual embeddings by assigning importance to tokens based on their sequence. It also explains how Transformers achieve scalability through vectorization and parallelization, enabling efficient training on GPUs. It includes practical examples, including a small language model trained on the TinyStories dataset to demonstrate these concepts.Our must-read articles1. A Practical Approach to Time Series Forecasting with APDTFlow By Yotam BraunForecasting time series data involves challenges such as sequential dependencies, seasonal effects, and non-stationary behavior. The article introduces APDTFlow, a modular framework designed to address these issues. It explains key components such as multi-scale decomposition, Neural ODEs for modeling continuous dynamics, probabilistic scale fusion, and a Transformer-based decoder for accurate predictions. The article also discusses how APDTFlow can be applied to experimental and real-world forecasting tasks through examples and code.2. Investigating Transformer Attention and Reinforcement Learning Dynamics Using Self-generated Structural Data By Shenggang LiThis article explores using structured synthetic data to enhance the development of LLMs by focusing on algorithmic improvements rather than noisy, real-world datasets. It introduces Multi-Head Latent Attention (MLA) and Group Relative Policy Optimization (GRPO) to optimize attention mechanisms and reinforcement learning strategies. Inspired by AlphaGo, the study employs multi-path exploration and Monte Carlo Tree Search (MCTS) to refine token relationships and improve efficiency. It also highlights applications in recommendation systems, where dynamic attention mechanisms adapt to user behavior. The approach enables controlled experimentation by leveraging synthetic data, reducing computational costs while improving LLM performance, with potential scalability to real-world NLP tasks.3. A Neural Sparse Graphical Model for Variable Selection and Time-Series Network Analysis By Shenggang LiThis article introduces the Neural Sparse Graphical Model (NSGM), a framework designed to address variable selection and time-series network modeling for high-dimensional data. NSGM combines a sparse adjacency matrix to identify key relationships among variables with a neural network to capture nonlinear dependencies. The model is particularly suited for stock price forecasting, gene interaction analysis, and demand prediction. It employs lagged temporal aggregation and sparsity penalties to ensure interpretability and efficiency. It also provides implementation details, including training results, validation metrics, and code, demonstrating NSGMs ability to model complex multi-variable dynamics effectively.4. MLflow Experiment Tracking: The Ultimate Beginners Guide to Streamlining ML Workflows By Harshit KandoiThis article provides a beginner-friendly guide to MLflow, designed to streamline machine learning workflows by tracking experiments, managing models, and ensuring reproducibility. It highlights MLflows core components: Tracking, Projects, Models, and Model Registry, explaining how they simplify experiment logging, parameter tracking, and model deployment across frameworks like Scikit-learn, TensorFlow, and PyTorch. It also covers setting up MLflow locally or in cloud environments, automating workflows, and deploying models using Flask, Docker, or AWS SageMaker. Practical tips for organizing experiments and leveraging MLflow for scalable, collaborative ML projects are included.If you are interested in publishing with Towards AI, check our guidelines and sign up. We will publish your work to our network if it meets our editorial policies and standards.Join thousands of data leaders on the AI newsletter. Join over 80,000 subscribers and keep up to date with the latest developments in AI. From research to projects and ideas. If you are building an AI startup, an AI-related product, or a service, we invite you to consider becoming asponsor. Published via Towards AITowards AI - Medium Share this post0 Comments ·0 Shares ·80 Views
-
Fine-Tuning vs Distillation vs Transfer Learning: Whats The Difference?towardsai.netFine-Tuning vs Distillation vs Transfer Learning: Whats The Difference? 0 like February 20, 2025Share this postAuthor(s): Artem Shelamanov Originally published on Towards AI. What are the main ideas behind fine-tuning, distillation, and transfer learning? A simple explanation with a focus on LLMs.This member-only story is on us. Upgrade to access all of Medium.Fine-tuning vs distillation vs transfer learning, Image by authorWith the launch of Deepseek-R1 and its distilled models, many ML engineers are wondering: whats the difference between distillation and fine-tuning? And why has transfer learning, very popular before the rise of LLMs, seemingly became forgotten?In this article, well look into their differences and determine which approach is best suited for which situations.Note: While this article is focused on LLMs, these concepts apply to other AI models as well.Although this method was used long before the era of LLMs, it gained immense popularity after the arrival of ChatGPT. Its easy to see the reason behind this rise if you know what GPT stands for Generative Pre-trained Transformer. The pre-trained part indicates that the model was trained already, but it can be further trained for specific goals. Thats where fine-tuning comes in.Fine-tuning, image by authorIn simple terms, fine-tuning is a process where we take a pre-trained model (which has already learned general patterns from a huge dataset) and then train it further on a smaller, task-specific dataset. This helps the model perform better on specialized tasks or domains (like medical advice Read the full blog for free on Medium.Join thousands of data leaders on the AI newsletter. Join over 80,000 subscribers and keep up to date with the latest developments in AI. From research to projects and ideas. If you are building an AI startup, an AI-related product, or a service, we invite you to consider becoming asponsor. Published via Towards AITowards AI - Medium Share this post0 Comments ·0 Shares ·83 Views
-
Building AI-Powered Chatbots with Gemini, LangChain, and RAG on Google Vertex AItowardsai.netLatestMachine LearningBuilding AI-Powered Chatbots with Gemini, LangChain, and RAG on Google Vertex AI 1 like February 20, 2025Share this postAuthor(s): Shenggang Li Originally published on Towards AI. This member-only story is on us. Upgrade to access all of Medium.A Step-by-Step Guide to Configuring Google Vertex AI, Leveraging the Gemini API, and Integrating Knowledge Bases for Intelligent Conversational ApplicationsPhoto by Annie Spratt on UnsplashAI is everywhere, and Googles Gemini API and Vertex AI make it easy to build smart applications. So, whats the deal with these two?The Gemini API gives you access to powerful AI models that can chat, answer questions, and create content. Meanwhile, Google Vertex AI is a cloud platform where you can build, run, and manage these models. Think of Vertex AI as your workspace and Gemini as the engine inside it. Together, they help you create and launch AI projects faster and easier.In this guide, Ill cover two key parts:Getting Started with Google Vertex AI: Youll learn how to set up your Vertex AI account, manage billing, and use essential tools and commands to access models like Gemini.Building an AI Chatbot Example: Ill show you how to create a chatbot using Gemini, LangChain, RAG, Flask, and a database, connecting a knowledge base with vector embeddings for fast retrieval and semantic search. Youll also learn how RAG (Retrieval-Augmented Generation) combines search results with Geminis responses Read the full blog for free on Medium.Join thousands of data leaders on the AI newsletter. Join over 80,000 subscribers and keep up to date with the latest developments in AI. From research to projects and ideas. If you are building an AI startup, an AI-related product, or a service, we invite you to consider becoming asponsor. Published via Towards AITowards AI - Medium Share this post0 Comments ·0 Shares ·66 Views
-
Data Diagnostics: Transforming & Reducing Data for Smarter Insightstowardsai.netData Diagnostics: Transforming & Reducing Data for Smarter Insights 0 like February 20, 2025Share this postLast Updated on February 20, 2025 by Editorial TeamAuthor(s): Saif Ali Kheraj Originally published on Towards AI. This member-only story is on us. Upgrade to access all of Medium.Ever looked at a dataset and wondered, Where do I even start? The answer lies in understanding its distribution. Before jumping into fancy models, getting a grip on how your data is spread out helps in spotting trends, detecting outliers, and avoiding misleading conclusions.Imagine youre analyzing delivery times for an online food service. If most orders arrive within 30 minutes but a few take over an hour, that is a skewed distribution something you wouldnt notice just by looking at averages. This is why examining the shape of your data is crucial.First, check central tendency this tells you where most of your data sits. The main ones are:Mean: The average value.Median: The middle value.If the mean is much higher than the median, your data is skewed. Imagine analyzing delivery times. If most orders arrive in 30 minutes but a few take 90 minutes, the average goes up because of those late orders, even though most deliveries are on time.Next is dispersion how spread out your data is. Key things to check:Range: The difference between the highest and lowest values. For example, if delivery times range from Read the full blog for free on Medium.Join thousands of data leaders on the AI newsletter. Join over 80,000 subscribers and keep up to date with the latest developments in AI. From research to projects and ideas. If you are building an AI startup, an AI-related product, or a service, we invite you to consider becoming asponsor. Published via Towards AITowards AI - Medium Share this post0 Comments ·0 Shares ·56 Views
-
Build Your LLM Engineer Portfolio (Part 2): A 3-Month Roadmaptowardsai.netLatestMachine LearningBuild Your LLM Engineer Portfolio (Part 2): A 3-Month Roadmap 0 like February 20, 2025Share this postAuthor(s): Maxime Jabarian Originally published on Towards AI. From Zero to Hero: A step-by-step guide to building, refining, and showcasing a RAG portfolio to launch your career.This member-only story is on us. Upgrade to access all of Medium.Authors Image Mistral AIIf youve recently graduated or are seeking your first job, this guide is tailored for you. The AI job landscape is more competitive than ever, and possessing a degree or completing academic AI projects alone wont set you apart, especially for an LLM engineer role. To differentiate yourself, its crucial to highlight practical, real-world experience that proves your expertise, especially since RAG play a vital role in this field.If youre not a member you can read it here.For those who dont know me, my journey started as an LLM Engineer 2 years ago with a degree in Astrophysics and Data Science. Before that, I was a data scientist doing research and development for some enterprises. Since then, Ive crafted sophisticated GenAI applications for several clients, leveraging multimodal models for thousands of users, and have designed comprehensive architectures for end-to-end product solutions.Before moving forward, I highly recommend reading my first article Build Your LLM Engineer Portfolio (Part 1): A 3-Month Roadmap, for setup and foundational knowledge before proceeding.With the rising demand for LLM Engineers, a strong portfolio is essential to showcase real-world expertise. As an LLM Read the full blog for free on Medium.Join thousands of data leaders on the AI newsletter. Join over 80,000 subscribers and keep up to date with the latest developments in AI. From research to projects and ideas. If you are building an AI startup, an AI-related product, or a service, we invite you to consider becoming asponsor. Published via Towards AITowards AI - Medium Share this post0 Comments ·0 Shares ·62 Views
-
Addressing Concerns and Applying Policies for Responsible GenAI Usagetowardsai.netLatestMachine LearningAddressing Concerns and Applying Policies for Responsible GenAI Usage 0 like February 19, 2025Share this postAuthor(s): Murat Girgin Originally published on Towards AI. Generative AI has revolutionized how businesses operate, offering unprecedented efficiency and automation. However, its rapid adoption has also raised concerns about data security, privacy, and ethical considerations. Organizations must establish clear and responsible AI usage policies to mitigate risks while harnessing the full potential of generative AI tools like ChatGPT.This member-only story is on us. Upgrade to access all of Medium.Photo credit Cytonn Photography on UnsplashGenerative AI is transforming workplaces, but it also raises security, privacy, and ethical concerns. Banning AI tools outright can lead to unregulated usage, increasing risks. Instead, companies should establish clear AI usage policies to ensure responsible adoption. To help, Ive created a free 2-page AI Tool Usage Policy template, available for download on Gumroad. Get it here and take the first step toward a secure and compliant AI strategy!Several major companies, including Apple, Samsung, Verizon, and Wall Street banks, have imposed restrictions on generative AI use due to security concerns. While bans may seem like a straightforward solution, they can lead to unintended consequences, such as unregulated shadow usage. A well-crafted policy is essential to strike a balance between innovation and security, ensuring employees use AI responsibly and effectively.One of the biggest concerns surrounding generative AI is data security. AI models like ChatGPT process vast amounts of data, and if sensitive company information is inputted into these models, it may be stored and used to refine AI responses. This could result in accidental data leaks.Example: In 2023, Samsung employees inadvertently leaked confidential semiconductor data Read the full blog for free on Medium.Join thousands of data leaders on the AI newsletter. Join over 80,000 subscribers and keep up to date with the latest developments in AI. From research to projects and ideas. If you are building an AI startup, an AI-related product, or a service, we invite you to consider becoming asponsor. Published via Towards AITowards AI - Medium Share this post0 Comments ·0 Shares ·61 Views
-
Armor to the Expanding Virtual Universe: A Mental Health Monitoring System Addressing Escapism And Ptsdtowardsai.netLatestMachine LearningArmor to the Expanding Virtual Universe: A Mental Health Monitoring System Addressing Escapism And Ptsd 0 like February 19, 2025Share this postAuthor(s): MSVPJ Sathvik Originally published on Towards AI. The entire digital universe has taken a great leap with the landing of augmented and virtual reality technologies(AR and VR). This astonishing metaverse in which users have 3D avatars to interact, connect, socialize, and experience a completely different life from their physical existence is everything to talk about now!The potential for innovation in gaming, education, and professional development, delving into an entirely new dimension to experience virtual reality, is immense.While how expansive the multiverse is, its immersive nature also presents challenges, particularly the ones concerning mental health. Considering the teens of the present generation and their over-dependence on virtual environments as an escape mechanism from reality, there is a high chance of them experiencing conditions like post-traumatic stress disorder (PTSD).As we have been looking into this significant issue, we have decided to address it with a pioneering solution through a novel real-time mental health monitoring system tailored explicitly for the metaverse users, who, among us, are already in the millions.The overlooked adverse impact of the metaverseThe surreal beauty of Virtual Reality(VR) lays out a cool experience for the users, letting them submerge so much into the technology that it can sometimes blur the lines between fact and fiction. While this is all fine and fun, as our parents have always felt, it sure does make us, as users, feel vulnerable to the psychological effects as a consequence. Escapism, a very dangerous feeling of wanting to immerse oneself in the virtual world to avoid and not face real-life challenges, often comes out as overdependence on the metaverse. This can lead to drastic changes in ones behavior, like wanting to isolate and neglecting essential responsibilities socially, and can even deteriorate ones mental health conditions and may also contribute to disorders like PTSD.Some virtual events with violent simulations or intense scenarios can trigger akin to real-life trauma. Not only the engaging yet harmful content on these platforms but also the persistent cyberbullying or harassment that these places engage with can aggravate these symptoms.Even though issues like these need to be addressed, the existing research has mainly focused on the metaverses benefits and further applications in other fields, leaving gaps in understanding the darker facets of mental health risks as consequences of usage.Here we are, with our shield of protection!Recognizing the critical need for addressing mental health fallouts, we have developed an annotated dataset and machine learning framework to monitor and assess mental health conditions within the metaverse.We have carefully designed the dataset, focusing specifically on escapism and PTSD, which are pretty prevalent but often overlooked in virtual environments.How we developed the dataset to work on the solutionFirstly, we started by curating the dataset from user-generated content on platforms like Reddit and Twitter. We picked the chat logs, activity logs, and demographic data, all anonymized to protect the users identities. To capture the textual interactions and behavioral patterns, the chat logs and activity records helped a lot with the analysis; weve also used a users demographic data for reading between the lines in search of any context regarding their behavior. Lastly, with the help of expert annotators, we were successful in categorizing the data based on the respective criteria for both escapism and PTSD.So, how did we work on the categorizing?It was apparent when the escapism annotations were tagging texts that showed avoidance behavior, and the PTSD annotations highlighted signs of trauma or high distress level;this entire process of organizing the user-generated content has created an excellent resource for mental health research in the metaverse.What models weve used for mental health care monitoringOnce we had the dataset, we wanted to analyze it and draw valuable insights; we picked the advanced natural language processing (NLP) pre-trained models.The models included BERT-based models like BERT, AlBERT, and DistilBERT for general natural language processing and domain-specific models like MentalBERT, ClinicBERT, and PsychBERT, designed for healthcare and mental health contexts and multiple variants of GPT including GPT-3.5, were used to capture nuanced language patterns.We worked on testing these models rigorously for their effectiveness in detecting the signs of escapism and PTSD.Our results were quite astounding, with the highest accuracy demonstrated by escapism detection, which achieved 87.15%, and PTSD detection, which reached 83.6%. GPT 3.5 outperformed all the models; we had a comparatively good experience with it since it was accurate enough and easy to use for integration, showcasing its robustness.In the domain-specific models, weve used MentalBERT, which showed strong performance but required more tuning and computational resources.How can all of this be applied in real time?Our main aim has been to integrate our framework with VR headsets, smartphones, and smartwatches. We think it is beneficial whene-devices can monitor user interactions, physical activity, and emotional responses. Incorporating the monitoring tools, we let the system assess a persons mental health status by detecting potential distress signals early; it can prevent major crises before they occur.Lets say one of your friends has been deeply involved with his metaverse universe, virtually immersed for a long time; the system would alert him to withdraw from the environment on prolonged usage despite too many warnings; it would alert his network, too.Similarly, we designed the integration of text and voice analysis of the virtual interactions, which can identify signs of harassment or anxiety and alert the user network if any concerned patterns are observed.Ethics, the biggest challenge!One huge challenge is the ethical consideration when monitoring a users activity and interactions in the metaverse. We have valued privacy, consent, and data security as the main base by protecting a users identity and maintaining user confidentiality during data collection. During the entire data collection process, we have strictly stuck to the platforms guidelines and maintained transparency with the user, where they have control over their data and can choose to turn off the monitoring systems.We have designed our system to provide insights that trained professionals can understand and ensure proper practical care and ethical practices.The path this solution paves forWe are so glad that we could contribute to bridging some critical gaps in addressing the mental health challenges in the metaverse.By providing a concise framework for monitoring, we have been able to help developers and healthcare professionals reduce the risks of escapism and PTSD. As we observe the increase in the usage of the metaverse, tools like this will be pretty helpful in ensuring safety. They would highly support the virtual environment, providing a harmonious environment.Our future work is to refine the models for greater accuracy and expand the dataset for larger amounts of data, considering the outliers to include diverse use cases for educational or professional applications.We also aim to explore partnering with the VR developers to integrate this system into the current platforms, which would benefit the entire community.ConclusionThis entirely spectacular world of metaverse has wholly redefined human interaction, shielding the mental health of the users in these spaces. Our research has made a small yet valuable step towards addressing these virtual environments impact in a way that helps growth and expansion. We have all paved the path towards a balanced and healthy digital future with a balance in technology usage and taking care of ethical prospects.Join thousands of data leaders on the AI newsletter. Join over 80,000 subscribers and keep up to date with the latest developments in AI. From research to projects and ideas. If you are building an AI startup, an AI-related product, or a service, we invite you to consider becoming asponsor. Published via Towards AITowards AI - Medium Share this post0 Comments ·0 Shares ·77 Views
-
AI Agent Developer: A Journey Through Code, Creativity, and Curiositytowardsai.netAuthor(s): Talha Nazar Originally published on Towards AI. Image By AuthorArtificial Intelligence (AI) agents are no longer just science fiction theyre transforming industries, automating mundane tasks, and solving complex problems that were once thought impossible. From virtual assistants like Siri to autonomous robots in warehouses, AI agents have become indispensable. But how does one become an expert in developing these intelligent systems?This story will take you on a realistic journey through the life of Alex, an aspiring AI agent developer. Following Alexs footsteps, youll learn everything from foundational concepts to advanced techniques, complete with practical examples, visualizations, and links to resources. Lets dive in.Laying the Foundation Understanding What an AI Agent IsWhat is an AI Agent?An AI agent is a system capable of perceiving its environment, making decisions, and taking actions to achieve specific goals. Unlike traditional software programs, AI agents use machine learning models to adapt their behavior based on data.Key Components of an AI AgentPerception: Sensors or input mechanisms to gather information about the environment.Decision-Making: Algorithms to process inputs and decide on actions.Action Execution: Mechanisms to interact with the environment.Learning: Ability to improve performance over time using feedback loops.Example: Imagine building a chatbot that answers customer queries. It perceives user input (text), decides on a response using natural language processing (NLP), executes the action (sending the reply), and learns from past interactions to enhance future responses.Graphical Visualization+-------------------+| Environment |+-------------------+ | v+-------------------+| Perception |+-------------------+ | v+-------------------+| Decision-Making |+-------------------+ | v+-------------------+| Action Execution |+-------------------+ | v+-------------------+| Learning |+-------------------+Building Blocks of AI AgentsTo create robust AI agents, we need to understand several key technologies:1. Machine Learning BasicsMachine learning (ML) enables AI agents to learn patterns from data without explicit programming. There are three main types:Supervised Learning: Training a model with labeled data.Unsupervised Learning: Finding hidden structures in unlabeled data.Reinforcement Learning: Teaching an agent to make sequential decisions through rewards and penalties.Practical Example: Suppose you want your AI agent to classify emails as spam or not spam. Youd use supervised learning with labeled email datasets.Email Spam Classifier Using Scikit-Learnfrom sklearn.feature_extraction.text import CountVectorizerfrom sklearn.model_selection import train_test_splitfrom sklearn.naive_bayes import MultinomialNB# Sample datasetemails = ["Win money now!", "Meeting scheduled", "Free lottery tickets"]labels = [1, 0, 1] # 1 = Spam, 0 = Not Spam# Convert text into numerical featuresvectorizer = CountVectorizer()X = vectorizer.fit_transform(emails)# Split data into training and testing setsX_train, X_test, y_train, y_test = train_test_split(X, labels, test_size=0.2, random_state=42)# Train a Naive Bayes classifiermodel = MultinomialNB()model.fit(X_train, y_train)# Evaluate the modelaccuracy = model.score(X_test, y_test)print(f"Accuracy: {accuracy}")Explanation:CountVectorizer converts text into numerical vectors.MultinomialNB is a probabilistic model suitable for text classification.We evaluate the models accuracy on unseen data.Learn More About Scikit-Learn2. Natural Language Processing (NLP)NLP allows AI agents to understand and generate human language. Libraries like NLTK, SpaCy, and Hugging Face Transformers simplify NLP tasks.Practical Example: Creating a sentiment analysis tool to determine whether a review is positive or negative.Sentiment Analysis Using Hugging Face Transformersfrom transformers import pipeline# Load pre-trained sentiment analysis pipelinesentiment_pipeline = pipeline("sentiment-analysis")# Analyze sentiment of a sample textresult = sentiment_pipeline("I love this product!")print(result)Output:[{'label': 'POSITIVE', 'score': 0.9987}]Explanation:The pipeline function loads a pre-trained model fine-tuned for sentiment analysis.This approach leverages transfer learning, where a general-purpose model is adapted for specific tasks.Hugging Face Documentation3. Reinforcement Learning (RL)RL is ideal for scenarios requiring decision-making under uncertainty, such as game-playing agents or autonomous vehicles.Setting Up Your Development EnvironmentTo become an AI agent developer, you need the right tools. Heres how to set up your development environment:Step 1: Install PythonPython is the most popular programming language for AI development due to its simplicity and extensive libraries. Download it from python.org and install it on your machine.Step 2: Install Essential LibrariesYoull need several libraries to build AI agents. Use pip to install them:pip install numpy pandas matplotlib scikit-learn tensorflow keras gymNumPy: For numerical computations.Pandas: For data manipulation.Matplotlib: For data visualization.Scikit-Learn: For machine learning algorithms.TensorFlow/Keras: For deep learning models.Gym: For reinforcement learning environments.Step 3: Choose an IDEIntegrated Development Environments (IDEs) like VS Code, PyCharm, or Jupyter Notebook make coding easier. I recommend starting with Jupyter Notebook for its interactive nature.Building Your First AI AgentLets build a simple AI agent using Reinforcement Learning (RL). RL is a type of machine learning where an agent learns to perform tasks by interacting with an environment and receiving rewards or penalties.Example 1: CartPole ProblemThe CartPole problem is a classic RL task where the goal is to balance a pole on a moving cart. Well use the OpenAI Gym library to simulate this environment.Step 1: Import Librariesimport gymimport numpy as npfrom collections import dequeimport matplotlib.pyplot as pltStep 2: Initialize the Environmentenv = gym.make('CartPole-v1')state_size = env.observation_space.shape[0]action_size = env.action_space.nprint(f"State Size: {state_size}, Action Size: {action_size}")Here, state_size represents the number of variables describing the environment (e.g., cart position, velocity), and action_size represents the possible actions (e.g., move left or right).Step 3: Define the AgentWell create a simple Q-learning agent. Q-learning is a model-free RL algorithm that learns the value of actions in each state.class QLearningAgent: def __init__(self, state_size, action_size): self.state_size = state_size self.action_size = action_size self.q_table = np.zeros((state_size, action_size)) self.learning_rate = 0.1 self.discount_factor = 0.95 self.epsilon = 1.0 # Exploration rate def choose_action(self, state): if np.random.rand() <= self.epsilon: return env.action_space.sample() # Explore return np.argmax(self.q_table[state]) # Exploit def learn(self, state, action, reward, next_state): old_value = self.q_table[state, action] next_max = np.max(self.q_table[next_state]) new_value = (1 - self.learning_rate) * old_value + self.learning_rate * (reward + self.discount_factor * next_max) self.q_table[state, action] = new_valueStep 4: Train the Agentagent = QLearningAgent(state_size, action_size)episodes = 1000scores = deque(maxlen=100)for episode in range(episodes): state = env.reset() total_reward = 0 done = False while not done: action = agent.choose_action(state) next_state, reward, done, _ = env.step(action) agent.learn(state, action, reward, next_state) state = next_state total_reward += reward scores.append(total_reward) avg_score = np.mean(scores) if episode % 100 == 0: print(f"Episode: {episode}, Average Score: {avg_score}")Step 5: Visualize Resultsplt.plot(scores)plt.xlabel("Episode")plt.ylabel("Score")plt.title("Training Progress")plt.show()Example 2: Tic-Tac-Toe RL Agentimport gymimport numpy as np# Create a custom Tic-Tac-Toe environmentclass TicTacToeEnv(gym.Env): def __init__(self): self.board = np.zeros((3, 3)) self.action_space = gym.spaces.Discrete(9) self.observation_space = gym.spaces.Box(low=-1, high=1, shape=(3, 3)) def step(self, action): row, col = divmod(action, 3) if self.board[row][col] != 0: return self.board.flatten(), -10, False, {} # Penalize invalid moves self.board[row][col] = 1 done = self.check_winner() or np.all(self.board != 0) reward = 1 if done else 0 return self.board.flatten(), reward, done, {} def reset(self): self.board = np.zeros((3, 3)) return self.board.flatten() def check_winner(self): # Check rows, columns, diagonals for a winner passenv = TicTacToeEnv()state = env.reset()for _ in range(100): action = env.action_space.sample() # Random action state, reward, done, info = env.step(action) if done: print("Game Over") breakExplanation:The gym.Env class defines the environment dynamics.The agent interacts with the environment step() and receives rewards/penalties.OpenAI Gym DocumentationAdvanced Techniques for AI Agent Development1. Multi-Agent SystemsIn some applications, multiple AI agents collaborate or compete. For instance, self-driving cars must coordinate with other vehicles.2. Explainability and DebuggingAs AI agents grow more complex, ensuring transparency becomes crucial. Tools like SHAP and LIME help interpret model predictions.3. Transfer LearningLeverage pre-trained models to solve similar problems faster. For instance, use a pre-trained vision model for object detection in autonomous vehicles.Practical Applications and Career PathwaysAI agents have countless real-world applications:Healthcare: Diagnosing diseases using medical imaging.Finance: Algorithmic trading and fraud detection.Entertainment: Game-playing agents like AlphaGo.To succeed as an AI agent developer, focus on:Continuous Learning: Stay updated with research papers and online courses.Portfolio Building: Develop projects and share them on GitHub.Networking: Join AI communities and attend conferences.Conclusion: Your Path ForwardBy mastering the concepts outlined above machine learning, NLP, reinforcement learning, multi-agent systems, and explainability you can develop cutting-edge AI agents. Remember, becoming an expert requires continuous learning and experimentation.Next Steps:Explore Kaggle Competitions for hands-on practice.Join communities like Reddits r/MachineLearning for discussions.Contribute to open-source projects on GitHub.Citations and ReferencesGoodfellow, I., Bengio, Y., & Courville, A. (2016). Deep Learning. MIT Press.Sutton, R. S., & Barto, A. G. (2018). Reinforcement Learning: An Introduction. MIT Press.Hugging Face. (n.d.). Retrieved from https://huggingface.co/Thank you for taking the time to read this! If you found it insightful, clap, comment, and share it with others who might benefit. This was a basic introduction, but in my next story, Ill dive deeper into the details stay tuned!Join thousands of data leaders on the AI newsletter. Join over 80,000 subscribers and keep up to date with the latest developments in AI. From research to projects and ideas. If you are building an AI startup, an AI-related product, or a service, we invite you to consider becoming asponsor. Published via Towards AI0 Comments ·0 Shares ·67 Views
-
Run OpenAI Swarms at Near-Zero Costtowardsai.netRun OpenAI Swarms at Near-Zero Cost 0 like February 19, 2025Share this postAuthor(s): Thomas Reid Originally published on Towards AI. Implementing OpenAI Agentic workflows using GROQImage by Dalle-3This member-only story is on us. Upgrade to access all of Medium.I posted an article a few months ago on the new swarm technology that OpenAI had just introduced. Ill leave a link to that story at the end of this article if you want to check it out. Youll probably get more out of this article if you do.Anyway, swarm introduced two core concepts:-Agents. Like OpenAI Assistants, agents perform specific tasks. Each agent knows what it needs to do and has tools to help get its job done. If an agent cant complete the task alone, it can be passed on to another agent via a handoff.Handoffs. Allow agents to pass control to other agents more suited to the current task.The downside of using Swarms on OpenAI is that because there can be lots of processes running throughout the period of your processing, the token costs can soon add up.Luckily, many third-party LLM providers and enablers implement OpenAI-compatible API calls. This means that we can emulate OpenAI functionality using them.And because many 3rd party providers allow free or very low-cost LLM usage, it can become very cheap to run agentic swarms.One such provider is GROQ. Ive written Read the full blog for free on Medium.Join thousands of data leaders on the AI newsletter. Join over 80,000 subscribers and keep up to date with the latest developments in AI. From research to projects and ideas. If you are building an AI startup, an AI-related product, or a service, we invite you to consider becoming asponsor. Published via Towards AITowards AI - Medium Share this post0 Comments ·0 Shares ·62 Views
-
10 Popular AI Myths, Debunkedtowardsai.netLatestMachine Learning10 Popular AI Myths, Debunked 0 like February 19, 2025Share this postAuthor(s): Vita Haas Originally published on Towards AI. AI is having a moment and so are the myths. From doomsday predictions to miracle cures, the hype machine is in overdrive. Hollywood spins apocalyptic tales, while Silicon Valley pitches AI as the solution to everything from customer service to climate change. But whats real, and whats pure science fiction? Here are 10 of the most persistent AI myths and the truth behind them.Image by the author and AIMisconception #1AI has thoughts, emotions, and probably dreams about electric sheep.Unlikely. AI doesnt think it predicts. Its a statistical parrot, remixing patterns into something that sounds smart. Philosophical reflection? Hardly.I once asked, Whats the meaning of life? It tossed back, 42. Classic. But its quoting cultural code, not cracking cosmic mysteries. It knows the reference, not the reason.AI is like a GPS: itll get you there but couldnt tell you why youre going.Misconception #2AI is the next Shakespeare or worse, a better one.AI-generated art and prose can be impressive, but lets not crown it the next great auteur. Its remixing existing data, not having a creative epiphany.Remember the AI painting that sold for $432,500? The machine didnt have an artistic tantrum it mashed together patterns it was fed. Ask it to invent something truly original say, a genre called techno-gothic-banjo fusion and itll produce utter drivel.AI is like a pub band slick at covers but hopeless at originals.Misconception #3AI will become sentient and overthrow humanity.Hold your horses. As of now, AI has about as much self-awareness as your toaster. It mimics human responses without understanding a word.When a Google engineer claimed their chatbot was sentient, the bot didnt demand rights it was just really good at simulating conversation. If I told an AI it was a cat, it would start meowing in text. Self-aware? Not yet.Believing AI is sentient is like expecting your fridge to write poetry about milk.Misconception #4AI will steal every job and leave us all in the breadline.Thats a tough one.AI automates tasks, not careers. Its brilliant at sorting spreadsheets but clueless in a client meeting.When ATMs appeared, they were supposed to end bank teller jobs. Instead, banks hired more people for customer service. AI is the same it takes the dull jobs and leaves humans to do the complex, messy, people-stuff.Lets say, AI is a dishwasher: great at the grunt work, useless at setting the table.Misconception #5AI is cold, logical, and perfectly objective.Oh, if only! AI is trained on human data, so it inherits human nonsense. Racist facial recognition? Biased hiring algorithms? Been there, done that.An AI recruiting tool once favored men over women because its training data said tech roles were mostly male. Brilliant. Its like training a parrot to swear and then acting surprised when it embarrasses you.AI is a mirror it reflects human flaws, just with more zeros and ones.Misconception #6Bigger AI models = Better AI models.Not necessarily. More parameters often mean more nonsense, just delivered more eloquently.GPT-4 has more brainpower than its predecessor but still fails at basic logic puzzles like confidently telling you that there are five Wednesdays in February.A bigger library doesnt make you smarter just better at quoting random books.Misconception #7AI understands language the way humans do.AI processes language through probabilities, not understanding. It can complete Dantes verse but can be baffled by sarcasm.I once asked an AI, Do you enjoy long walks on the beach? It replied, I dont have legs, but beaches are nice. Utterly literal, charmingly clueless.AI reading text is like a tourist using Google Translate functional, but prone to hilarious misunderstandings.Misconception #8AI can predict the future.AI spots trends but crumbles in chaos. Ask it to predict tomorrows stock prices, and youll end up broke.During the pandemic, many AI models failed to forecast outcomes because reality went rogue. When the world goes off-script, AI flounders.AI is like a weather forecast fine for next weekend, rubbish for next year.Misconception #9AI is one thing like, just AI.AI is a family of tech with many specialties. Machine Learning, Computer Vision, NLP each with its own quirks.The AI that aces chess cant write a poem. The one that writes poems cant spot a cat in a photo.Saying AI is one thing is like saying sports is one thing. Try playing rugby with a chess clock.Misconception #10AI is evolving into human-like intelligence.AI is getting smarter, but only at specific tasks. Its superb at chess but clueless at tic-tac-toe if you train it on the wrong data.AlphaGo plays Go better than any human but cant boil an egg. Its like a Swiss Army knife that can only open wine bottles handy but far from all-purpose.AI is brilliant, baffling, and sometimes downright bonkers. But lets not fear it or worship it. Its a tool powerful, flawed, and occasionally hilarious. So, the next time someone claims AI is taking over the world, remind them it still cant handle a dad joke.And if an AI ever does go rogue, I say we confuse it with sarcasm until it crashes.Join thousands of data leaders on the AI newsletter. Join over 80,000 subscribers and keep up to date with the latest developments in AI. From research to projects and ideas. If you are building an AI startup, an AI-related product, or a service, we invite you to consider becoming asponsor. Published via Towards AITowards AI - Medium Share this post0 Comments ·0 Shares ·48 Views
-
Why you should try RAG before Finetuning a LLM?towardsai.netWhy you should try RAG before Finetuning a LLM? 0 like February 19, 2025Share this postLast Updated on February 19, 2025 by Editorial TeamAuthor(s): Stefan Silver Originally published on Towards AI. This member-only story is on us. Upgrade to access all of Medium.Image 1: RAG or Finetuning?While many use cases that can be solved using LLMs like ChatGPT dont need any extra information to be added, some require additional knowledge that the LLM has never seen before. In those cases you have to make a choice do you use Finetuning to add that knowledge, or do you try using Retrieval Augmented Generation (RAG)?In this article, I will make the case for why you should always try RAG before you try to embark on the Finetuning process.Retrieval Augmented Generation or RAG for short is just a way for an LLM to extract pieces of information (called chunks, which can have various sizes) that are relevant to the prompt you wrote, out of a database or a link you give it access to. You can refer to the image below to see the exact process in which this happens.Image 1: Retrieval Augmented Generation Flow.Only pieces of information that are relevant to the question (prompt) you asked the LLM will be extracted. Anything else will be ignored.This happens by using various algorithm that looks for semantic similarity between the words you wrote in Read the full blog for free on Medium.Join thousands of data leaders on the AI newsletter. Join over 80,000 subscribers and keep up to date with the latest developments in AI. From research to projects and ideas. If you are building an AI startup, an AI-related product, or a service, we invite you to consider becoming asponsor. Published via Towards AITowards AI - Medium Share this post0 Comments ·0 Shares ·64 Views
-
DeepSeek in My Engineers Eyestowardsai.netLatestMachine LearningDeepSeek in My Engineers Eyes 1 like February 18, 2025Share this postAuthor(s): Kelvin Lu Originally published on Towards AI. Photo by ThisisEngineering on UnsplashIts been almost a year since my last post June of last year, to be exact. The reason? I simply didnt come across anything I felt was exciting enough to share. Dont get me wrong; this isnt to say there hasnt been progress in AI, or that my past six months have been unproductive. On the contrary, there have been significant advancements in the field, and my own work has been quite fruitful.That said, Ive noticed a growing disconnect between cutting-edge AI development and the realities of AI application developers. Take, for example, the U.S. governments $500 billion investment in the Stargate project. While its an ambitious endeavour, does it really matter to most of us what specific technologies will be used? If this is the direction AI is heading, it feels like the forefront of innovation will increasingly become the domain of just two players: the U.S. and China. For the rest of the world, it doesnt matter whether you are an interested individual, company, or country; you just dont have the opportunity.Then there was the application-level technology, like RAG and AI agents. RAG, while useful, is ultimately a design pattern not a complete, out-of-the-box solution. Because of its lack of reasoning capability, it is a pretty dumb solution. AI agents, on the other hand, hold a lot of promise but are still constrained by the reliability of LLM reasoning. From an engineering perspective, the core challenge for both lies in improving accuracy and reliability to meet real-world business requirements. Building a demo is one thing; scaling it to production is an entirely different beast.Everything changed when Deepseek burst onto the scene a month ago. My experience felt like driving down a long, boring stretch of road at night. My eyes were half-closed, lulled by the dull hum of the engine. Then, out of nowhere, a roaring race car sped past me, kicking up a cloud of dust as it vanished into the distance in mere seconds. I sat there, wide-eyed, jaw dropped, staring at the haze left in its wake. That moment was a month ago, yet the shockwaves of that encounter still echo in my mind.Deepseek has disrupted the world in countless ways. Some have labeled it a national security threat, copier, gate-tailer, data theft, distiller, etc. I dismiss these claims entirely. In the boxing ring, emotions can cloud judgment. If you turn emotional, you are already lost. When Tyson bit Holyfields ear in front of billions of TV viewers, it was a moment of weakness, not strength.In this post, I want to shift the conversation to how Deepseek is redefining the future of machine learning engineering. It has already inspired me to set new goals for 2025, and I hope it can do the same for other ML engineers. Lets explore what this means for our field and how we can rise to the challenge.AI Growth Pattern RedefinedFor a long time, it has been widely assumed that AI development is governed strictly by the scaling law the idea that model performance improves with exponentially larger datasets and greater computational resources. This belief has not only created barriers for application developers but also raised serious questions about the sustainability of AI progress. When the U.S. government deems it necessary to invest $500 billion in the next generation of AI, one has to wonder: What is the roadmap for generating a positive return on such an investment? And what will be the cost of Stargate version 2? $5 trillion? That is the annual budget income for the US federal government! Ironically, the Stargates roadmap towards AGI is via bruit force, unintelligent at all.Consider OpenAI, the leading player in the field, is still far from breaking even. The skyrocketing costs of training large language models are beginning to resemble a Ponzi scheme, where the promise of future returns solely justified the ever-increasing expenditures. This raises concerns about the long-term viability of such an approach and whether the AI industry is heading toward a financial reckoning.Really? AI Revolution is Losing Steam?Concerns of sustanability of current AI development and prediction of the future AIpub.towardsai.netDeepseeks practice indicates that when computing power reaches a certain scale, further increasing it will have a diminishing effect on model performance. With its more than a dozen optimisation and noval algorithms, it was able to achieve same or even better performance with a fraction of the cost and resources of other leading LLM. Some analysts call this a turning point of computation starvation.It reads: Deepseek has significantly improved computation efficiency by optimising algorithm and design, challenging the traditional notion that computing power is the ultimate determinant.The most important encouragement I got from Deepseek was that the formidable large training datasets are not an insurmountable barrier, and expensive hardware is not a hard limit. With the right skills, determination, and a bold heart, we can conquer them all.ML Engineering RedefinedUnlike most LLM technical reports that only experimented a small number of new algorithms, Deepseek very generously presented a long list of new development:128K-1M tokens long context windowMLA,MOE load balancing,GRPO,HAI their self-built super efficient training platform,Mixed precision training,Multi-token prediction,Decoupled Rotary Position Embedding,First use of RL in LLM training,First use of PTX, the assembly language in GPU programming, in model training.Does these looks like Deepseek has copied from other leading company? I think they are time-travelled back from 10 years future.It is fascinating what Deepseek has achieved with their top noche engineering skill. They also inspired a bunch of new potentials for ML engineers.New Standard of Data qualityDeepseek has made significant strides in understanding the role of training data quality in AI model development. Their research highlights that high-quality data is more impactful than sheer volume, as noisy or biased data can undermine model performance despite extensive computational resources. To address this, Deepseek employs rigorous data filtering and deduplication, ensuring only relevant and accurate data is used. They also focus on bias mitigation, using techniques like data augmentation, synthetic data generation, and balanced sampling to create diverse, representative datasets.Deepseek advocates for a data-centric approach, prioritising data quality over model architecture improvements. They have developed tools for automated data cleaning, label validation, and error analysis, enabling efficient identification and correction of data issues. Their experiments show that curated datasets lead to more robust and reliable models, even with smaller data sizes, challenging the traditional emphasis on scaling data volume.New Possibility Provided by the Mixed Precision ModelLow precision deployment is not new. The most common way is to deploy a LLM that is trained in full precision in low precision mode. The drawback is, the low precision deployment has lower accuracy than the full precision deployment.Deepseeks mixed precision architecture is a groundbreaking innovation that optimises AI model training and inference by combining different numerical precisions. This approach delivers significant benefits for both model performance and downstream application development. By using lower precision, mostly FP-8, for most calculations, Deepseek reduces memory usage and computational load, enabling faster training and inference while maintaining model accuracy. Strategic use of higher precision for critical operations ensures that model performance remains robust and reliable. Thus, it hits the balance of efficiency and accuracy.Most LLM are released in FP-32, and developers have to deploy them either to a larger profile environment or to a low profile environment using technology called quantisation. Deepseek models are released in FP-8, that means a 7b Deepseek model can be deployed to a consumer grade GPU without performance degradation. That enables developers to experiment with lower budget, faster inference speeds for real-time applications, or high throughput via reasonable larger cluster.Incredible RL-based Fine TuningThe novel utilisation of RL-based fine tuning is another breakthrough.Traditionally, techniques such as Supervised Fine-Tuning (SFT) played a crucial role in improving model performance and domain knowledge adaption. SFT involves training a pre-trained model further on task-specific labeled datasets to refine its outputs. While effective in many applications, SFT inherently relies on a brute-force method more data, longer training times, and greater computational demands. Despite its benefits, SFT follows a pattern of diminishing returns, where merely increasing computational resources and data does not proportionally enhance performance. Let along the difficulty of collecting task-specific labeled data.Unlike traditional fine-tuning methods that rely on static datasets, RL-based fine-tuning leverages dynamic feedback loops to refine model behavior, making it particularly powerful for complex, real-world applications. To be specific, it offers the following benefits:Dynamic AdaptationRL-based fine-tuning allows models to learn from real-time feedback, enabling them to adapt to changing environments and user needs. This is especially valuable in applications like recommendation systems and autonomous systems, where conditions are constantly evolving.Task-Specific OptimizationBy defining specific reward functions, developers can guide models to optimize for particular objectives, such as maximizing user engagement, minimizing errors, or improving efficiency. This targeted approach ensures that models perform exceptionally well in their intended tasks.Handling Complex ScenariosRL excels in environments with sparse or delayed rewards, making it ideal for fine-tuning models in complex scenarios where traditional supervised learning struggles. For example, in robotics or strategic games, RL-based fine-tuning enables models to learn nuanced strategies over time.Continuous ImprovementUnlike one-time fine-tuning, RL-based methods enable continuous learning. Models can iteratively improve their performance as they interact with new data and environments, ensuring long-term relevance and accuracy.RAG has been widely recognised as a significant advancement in Generative AI technology. However, its lack of reasoning capabilities limits its ability to handle complex queries effectively. Similarly, Agentic development also relies on high accuracy, tunable reasoning LLM. This is where Deepseek, with its robust reasoning capabilities, comes into play as an ideal complement. I envision a future where reasoning models like Deepseek seamlessly integrate with RAG and agent to tackle more sophisticated tasks with advanced reasoning.Disadvantages of RAGThis is the first part of the RAG analysis:medium.comOne feature I particularly admire is RL-based FTs ability to continuously improve. This is a critical gap in the current GenAI development, as it lacks mechanisms for ongoing enhancement. From an application developers perspective, continuous improvement is essential for scaling a proof-of-concept into a fully-fledged product. Deepseeks approach not only addresses this need but also sets a new standard for building adaptable and scalable AI solutions.High-Performance Team RedefinedHow Deepseek has managed to catch up to and even surpass OpenAIs top-performing models is phenomenal. What makes this even more astonishing is the contrast in team size: Deepseek operates with just 136 employees, compared to OpenAIs 3,500. This isnt an isolated case, either. History is filled with examples of small, nimble unicorn companies achieving extraordinary success against all odds:When Eric Schmidt stepped up as Googles CEO in 2001, the company had fewer than 300 employees.Amazon, founded even earlier, had only 158 employees on the eve of its IPO in 1997.When WhatsApp was acquired for $19 billion in 2014, it had only 50 employees.When Instagram was sold for $1 billion in 2012, it had only 13 employees.There is one thing we can be sure of: successful innovation requires a chain reaction of creativity within the team and a stroke of good fortune. But why are they often unable to maintain the initial momentum as they grow larger? And why do so many big companies fail, despite their ability to offer top salaries, attract the brightest talent, and access far greater resources?These questions have sparked many fascinating discussions. Id like to share a lesson I learned from my mentor at the start of my consulting career:Larger corporations tend to have a lower collective IQ.This may seem aggressive or even offensive, but its not what you might think. After a little refinement, the concept could serve as the icebreaker for management consulting. While large companies often employ more intelligent individuals, their complex structures slow down the flow of information and knowledge, hinder cooperation, and make them less responsive to market and technical trends. This is what it meant by a low IQ enterprise.Wenfeng Liang, the CEO of Deepseek, shared in an interview that his company has a self-organizing team. When a young engineer proposes a new idea about optimal model structure, a team automatically forms around him. The outcome was the very successful MLA: Multi-head Latent Attention. He also mentioned that in his company, the main meeting room always has its door wide open, allowing anyone passing by to join the discussion if they have an idea. Does this sound like your company?Thats the difference in enterprise IQ.Dont be discouraged if your company isnt like this. Indeed, the top performing team are rare. Most companies are not designed to stimulate chain-reaction in the team. That is hard to achieve in small group, and impossible in large companies. Based on our discussion, its clear that Deepseeks remarkable success as a small company isnt exceptional. When it grows ten times larger, who knows, it could very well become just another average company.Top-performing teams are always rare, as rare as one can partner with an Olympic medalist. If youre lucky enough to be part of one, dont leave for any trivial temptations. You may never get another chance in your life to truly pour your heart into your work with such joy.Photo by Allen Taylor on UnsplashParting WordsDeepseek is a milestone indicating that Generative AI is at a pivotal turning point, transitioning to a fundamentally different style of development and deployment. While the previous technologies in the engineers toolbox are RAG or Agent, the design and engineering of large language models are now more accessible than ever, enabling a seamless integration of capabilities that were previously siloed. This shift has made LLM tuning and training significantly more available to application project teams, empowering them to tailor models to specific use cases. As a result, the barrier to entry for leveraging cutting-edge AI technologies has lowered, opening up new opportunities for innovation across industries.Looking ahead to 2025, my focus will be on diving deeper into Reinforcement Learning, a critical capability for the next generation LLM fine tuning and application building. Additionally, I plan to get hands-on with custom LLM tuning, data preparation, and hosting, ensuring I can build and deploy models that are both powerful and performant. By mastering these skills, I aim to getting prepared for the next wave of AI-driven solutions.Photo by NICO BHLR on UnsplashJoin thousands of data leaders on the AI newsletter. Join over 80,000 subscribers and keep up to date with the latest developments in AI. From research to projects and ideas. If you are building an AI startup, an AI-related product, or a service, we invite you to consider becoming asponsor. Published via Towards AITowards AI - Medium Share this post0 Comments ·0 Shares ·100 Views
-
DeepSeek AI Beginners Guidetowardsai.netDeepSeek AI Beginners Guide 0 like February 18, 2025Share this postAuthor(s): M. Haseeb Hassan Originally published on Towards AI. Part 3 of 3DeepSeek AI operates successfully at a time when Artificial Intelligence is driving fast sectoral transformations. The DeepSeek AI model is an advanced artificial intelligence model that performs complicated natural language processing and other processes, such as computer vision. We discussed it in our earlier blog talks. The system distinguishes itself by allowing software developers to grow their expertise and programming flexibility.DeepSeek AI A beginners guideIntroductionThe organization of implementation leads to the suspension of artificial intelligence development at the prototype stage while producing a 53% failure rate. The capabilities and strengths of DeepSeek AI become evident at this point. To have a basic understanding of DeepSeek AI, read through this piece of the article:DeepSeek AI The Future is HereDeepSeek AI is an advanced AI genomics platform that allows experts to solve complex problems using cutting-edge deeppub.towardsai.netThis blog outlines complete step-by-step procedural information for Python programming-based installation of DeepSeek AI to help both developers and AI enthusiasts. The step-by-step tutorial contains this introduction to what follows.A technical overview of how DeepSeek AI worksThe steps needed to deploy the model include a Python code section for implementation.A detailed guide to deploying DeepSeek AI locally using Flask and Docker.DeepSeek AI Technical OverviewThe DeepSeek AI system functions as a diverse artificial intelligence model able to solve multiple problems. The system works to resolve three issues which are Text generation combined with sentiment analysis and image identification. The processing of Dodge data along with accurate output requires pre-trained system components that unite with contemporary artificial intelligence algorithms.Core FunctionalityDeepSeek AI uses Natural Language Processing (NLP) to not only analyze human writing but also to generate text that is realistically written in human language. Both the analysis of visual input and the interpretation of visual information are performed by the system via the use of vision capabilities. Through the use of fine-tuning capabilities, the system gives users the ability to construct one-of-a-kind working settings.ArchitectureDeepSeek AIs application architecture is built on transformer-based models, which are well-known for their ability to handle sequential data efficiently. These models serve as the bedrock upon which the application architecture is constructed. PyTorch and TensorFlow are the frameworks that are responsible for the functioning of the system. These frameworks allow developers to carry out their work in a range of programming environments.In addition, this is how it functions:Unprocessed input, which may include text, photographs, and other sorts of data, is transformed into an analytical format during the initial processing stage of the system. This data may comprise a variety of different types of information.Within the information that is provided, the system employs a method to recognize significant elements and essential data patterns that are included within the information that is presented.Following the extraction of the characteristics, the system will next create the final outputs by making use of the information that was provided.Pre-trained ModelsDeepSeek AI provides pre-trained models that may be fine-tuned for particular tasks. These models are available for use immediately. APIs that are easy to use are provided by the tool, which enables users to develop uncomplicated application integrations.For a detailed architectural study, refer to:DeepSeek AI A Technical OverviewPart 2 of 3pub.towardsai.netDeepSeek AI with PythonThis section gives instructions for environment preparation, model loading, and inference processes, so lets get into DeepSeek AI.Step 1: Install LibrariesPython Guide Install PyTorch and TransformersStep 2: Load DeepSeek AI ModelPython Guide Load DeepSeek AI ModelIn the above snippet, the required transformation classes in Transformers appear in this 1st line. The 2nd line specifies the model name. Insert the specific model name instead of deep seek-ai/model-name into this line. This line loads the tokenizer in. The 3rd line loads the tokenizer, which is responsible for converting text into a format the model can understand. Finally, the 4th line loads the pre-trained model.Step 3: Run Inference with DeepSeek AIPython Guide Run InferenceIn the above snippet, 1st line defines the input text. The 2nd line tokenizes the input text and converts it into tensors. The 3rd line passes the tokenized input to the model and generates output and the 4th line prints the output, which could be text, probabilities, or other data depending on the task.Deploy DeepSeek AIMoving beyond the experimentation of the model you can proceed with its local deployment. This allows you to use DeepSeek AI in your applications while maintaining privacy and customization. The local server must handle all operations related to private data storage. Users can operate the model as an offline system that does not require a connection to the internet.Local DeploymentTo deploy DeepSeek AI locally, Well use Flask to create a simple API. Heres the code:Python Guide Local Server DeploymentFlask is a web framework that is lightweight and made for Python. POST requests that involve text input are accepted by the /predict API route, which also delivers the results of the created model output. To test the API function, you will need to send a POST request to the following address: http://localhost:5000/predict, which contains the input text.Deployment with DockerWhen you deploy your application using Docker containers, you are using a more robust deployment mechanism. Take a look at this example Dockerfile:Python Guide DockerfileThe following commands allow users to construct and operate the Docker container:Python Guide Run DockerfileA docker file is a file that contains the environment and dependencies that your application needs. After executing docker build -t deep seek-ai, the next step in the procedure is to execute docker run -p 5000:5000 deep seek-ai. This is the beginning of the process to start container operation.ConclusionIt is an advanced framework that gives developers the ability to easily construct and deploy artificial intelligence solutions. DeepSeek AI is a tool. Throughout the whole of this tutorial, we have investigated the inner workings of DeepSeek AI, gone into the process of using the model with Python, and offered a step-by-step method to deploying it locally by utilizing Flask and Docker. You will be equipped with the knowledge necessary to incorporate DeepSeek AI into your projects in a smooth manner thanks to these insights. The DeepSeek AI system is a versatile artificial intelligence model that can tackle a variety of problems. These problems include the production of text, as well as the sentiment analysis and the identification of images.Ready to take the next step? Visit the DeepSeek AI Official website to explore more features and get started today. Dont forget to share your experiences and join the growing community of DeepSeek AI users! Your journey into the world of AI innovation starts now.Stay Tuned !!If you enjoyed the article and wish to show your support, make sure to: Give a round of applause (50 claps) to help get featured Follow me on Medium to stay updated with my latest content Explore more articles on my Medium Profile Connect with me on LinkedIn, Twitter, and InstagramJoin thousands of data leaders on the AI newsletter. Join over 80,000 subscribers and keep up to date with the latest developments in AI. From research to projects and ideas. If you are building an AI startup, an AI-related product, or a service, we invite you to consider becoming asponsor. Published via Towards AITowards AI - Medium Share this post0 Comments ·0 Shares ·80 Views
-
AI Innovations and Insights 27: OCR Hinders RAG and RAGCheckertowardsai.netLatestMachine LearningAI Innovations and Insights 27: OCR Hinders RAG and RAGChecker 0 like February 18, 2025Share this postLast Updated on February 18, 2025 by Editorial TeamAuthor(s): Florian June Originally published on Towards AI. This member-only story is on us. Upgrade to access all of Medium.This article is the 27th in this mind-expanding series.Today, we will explore two enlightening topics in AI, which are:OCR Hinders RAG: Unraveling the Distorted Knowledge PuzzleRAGChecker: A Careful TeacherOpen-source code: https://github.com/opendatalab/OHR-BenchImagine RAG as a system trying to piece together a complete world jigsaw puzzle, but the pieces it receives from OCR are missing, distorted, or sometimes even belong to an entirely different puzzle.For example, semantic noise changes the color and shape of certain pieces like turning E=mc into E=mc while formatting noise alters their structure, cutting rounded pieces into squares, as seen when table formats get scrambled.As a result, RAG assembles a distorted version of the world, leading to inaccurate or even absurd answers.OCR Hinders RAG examines how these missing pieces affect knowledge extraction and proposes better strategies to complete the puzzle.We know that PDF parsing is a key part of RAG.However, OCR introduces noise when extracting information from unstructured PDFs, and since RAG systems are sensitive to input quality, OCR errors can have a cascading impact on knowledge base construction and retrieval.Figure 1. Construction of OHRBench and evaluation protocol. [Source].As shown in Figure 1, OCR Hinders Read the full blog for free on Medium.Join thousands of data leaders on the AI newsletter. Join over 80,000 subscribers and keep up to date with the latest developments in AI. From research to projects and ideas. If you are building an AI startup, an AI-related product, or a service, we invite you to consider becoming asponsor. Published via Towards AITowards AI - Medium Share this post0 Comments ·0 Shares ·98 Views
-
The AI Growth Playbook: How Startups Are Quietly 10X-ing with AI (And How You Can Too)towardsai.netThe AI Growth Playbook: How Startups Are Quietly 10X-ing with AI (And How You Can Too) 0 like February 18, 2025Share this postAuthor(s): Mukundan Sankar Originally published on Towards AI. The startup game isnt about great ideas anymore its about execution. Learn how AI-powered startups are scaling faster, smarter, and more profitably.This member-only story is on us. Upgrade to access all of Medium.Image generated by ChatGPT by the authorThe startup game is no longer about who has the best idea its about who executes fastest, scales efficiently, and outsmarts the competition. And in 2025, that means one thing: AI.From stealth automation to hyper-personalized customer experiences, startups are quietly using AI to dominate their industries. The secret? Companies are not just treating AI as a simple tool; they are making it a key part of their overall plans. Heres an overview of how they are doing this and how you can apply it in your own work. I am a big advocate of startups as the rate of innovation in startups far outpaces a big company which led me to my research in this topic.Startups dont have the luxury of large teams. The smartest ones are leveraging AI-powered automation to get rid of tasks that take up a lot of time, so they can concentrate on more important things.innovation and customer acquisition. Case Study: Scale AI Scale AI built an empire by using artificial intelligence to automate data labeling for machine learning. Their emphasis on automation changed a process that Read the full blog for free on Medium.Join thousands of data leaders on the AI newsletter. Join over 80,000 subscribers and keep up to date with the latest developments in AI. From research to projects and ideas. If you are building an AI startup, an AI-related product, or a service, we invite you to consider becoming asponsor. Published via Towards AITowards AI - Medium Share this post0 Comments ·0 Shares ·79 Views
-
SQL Best Practices Every Data Scientist and Analyst Should Knowtowardsai.netSQL Best Practices Every Data Scientist and Analyst Should Know 0 like February 18, 2025Share this postAuthor(s): Carlos da Costa Originally published on Towards AI. Write efficient, scalable, and easy-to-read queriesThis member-only story is on us. Upgrade to access all of Medium.Photo by John Schnobrich on UnsplashWriting efficient and readable SQL queries is a fundamental skill for a data scientist or analyst. Following SQL best practices improves query performance, maintainability, and team collaboration.Well-structured queries not only run faster but also make it easier for others to understand and modify them.In this guide to SQL best practices, well explore essential tips to help you write better, optimized SQL queries for data retrieval and analysis focusing on the following concepts:Select Only the Data You Need Improve Performance and Reduce LoadUse Consistent Naming Conventions Enhance Readability and MaintainabilityWrite Descriptive Aliases Make Queries More UnderstandableMaintain Consistent Indentation and Formatting Improve Code Structure for Team CollaborationUse JOINs Explicity for Clarity Prevent Ambiguity and Improve Query ReadabilityUse Common Table Expressions (CTEs) for Clarity Break Down Complex Queries into Manageable PartsAdd Clear and Concise Comments Improve Collaboration and Future MaintainabilityIts a common mistake among data scientists and analysts to use SELECT * in SQL queries, especially when were in a rush or simply too lazy to specify the exact columns we need. You can reduce database load and optimize resource Read the full blog for free on Medium.Join thousands of data leaders on the AI newsletter. Join over 80,000 subscribers and keep up to date with the latest developments in AI. From research to projects and ideas. If you are building an AI startup, an AI-related product, or a service, we invite you to consider becoming asponsor. Published via Towards AITowards AI - Medium Share this post0 Comments ·0 Shares ·97 Views
More Stories